Deep Image Super Resolution via Natural Image Priors
2018 IEEE International Conference on Acoustics, Speech and Signal Processing (ICASSP)(2018)
摘要
Single image super-resolution (SR) via deep learning has recently gained significant attention in the literature. Convolutional neural networks (CNNs) are typically learned to represent the mapping between low-resolution (LR) and high-resolution (HR) images/patches with the help of training examples. Most existing deep networks for SR produce high quality results when training data is abundant. However, their performance degrades sharply when training is limited. We propose to regularize deep structures with prior knowledge about the images so that they can capture more structural information from the same limited data. In particular, we incorporate in a tractable fashion within the CNN framework, natural image priors which have shown to have much recent success in imaging and vision inverse problems. Experimental results show that the proposed deep network with natural image priors is particularly effective in training starved regimes.
更多查看译文
关键词
high-resolution,deep network,deep structures,natural image priors,vision inverse problems,deep image super resolution,deep learning,convolutional neural networks,low-resolution,patches,CNN framework
AI 理解论文
溯源树
样例
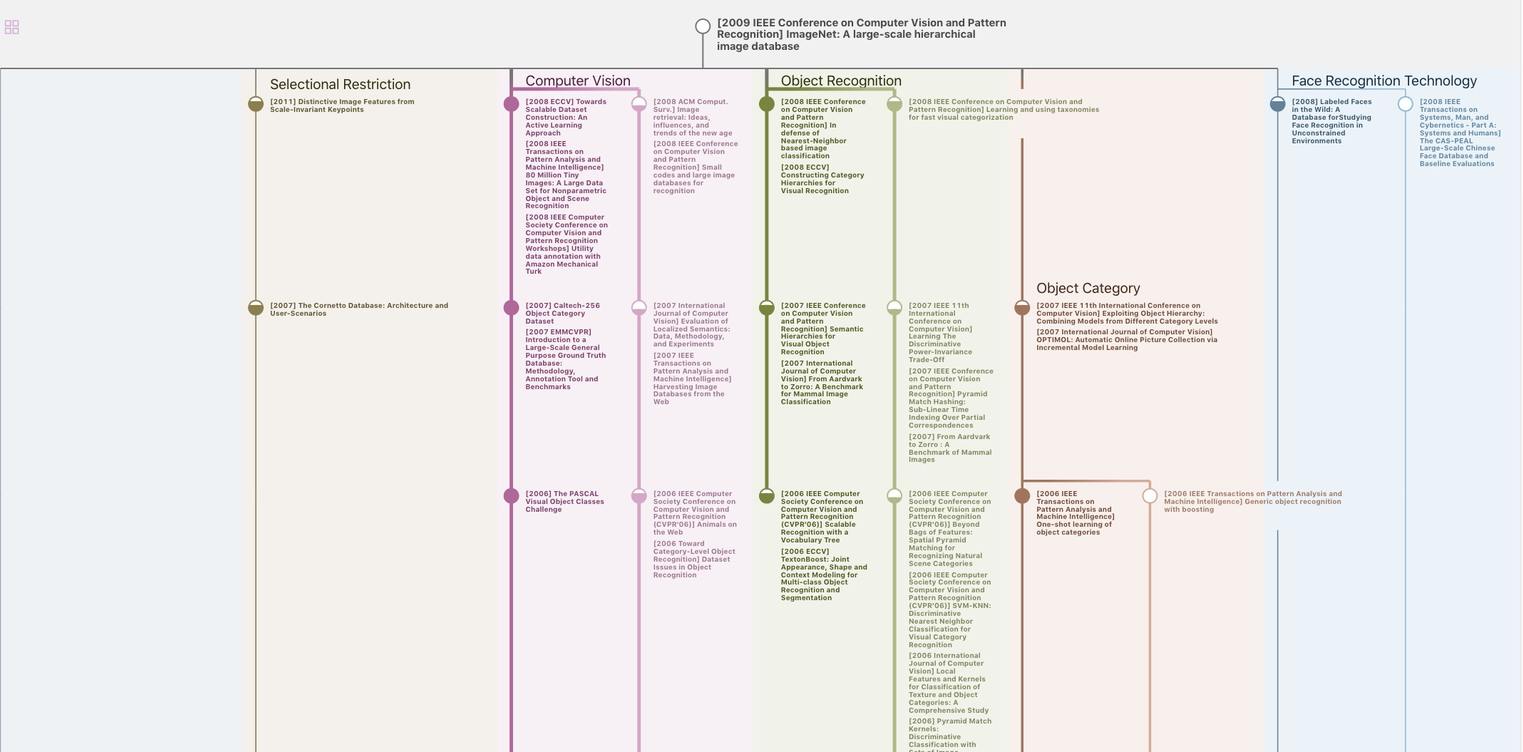
生成溯源树,研究论文发展脉络
Chat Paper
正在生成论文摘要