A Heterogeneous Ensemble Learning Voting Method for Fatigue Detection in Daily Activities.
JOURNAL OF ADVANCED COMPUTATIONAL INTELLIGENCE AND INTELLIGENT INFORMATICS(2018)
摘要
Lower extremity fatigue is a risk factor for falls and injuries. This paper proposes a machine learning system to detect fatigue states, which considers the different influences of common daily activities on physical health. A wearable inertial unit is devised for gait data acquisition. The collected data are reorganized into nine data subsets for dimension reduction, and then preprocessed via gait cycle division, visualization, and oversampling. Then, a heterogeneous ensemble learning voting method is employed to train nine classifiers. The results indicate that the method reaches an accuracy of 92%, which is obtained by the plurality voting method using data subset prediction classes. Comparing the results shows that the final result is more accurate than the results of each individual data subset, and the heterogeneous voting method is advantageous when balancing out individual weaknesses of a set of equally well-performing models.
更多查看译文
关键词
lower extremity fatigue,gait data,heterogeneous voting method
AI 理解论文
溯源树
样例
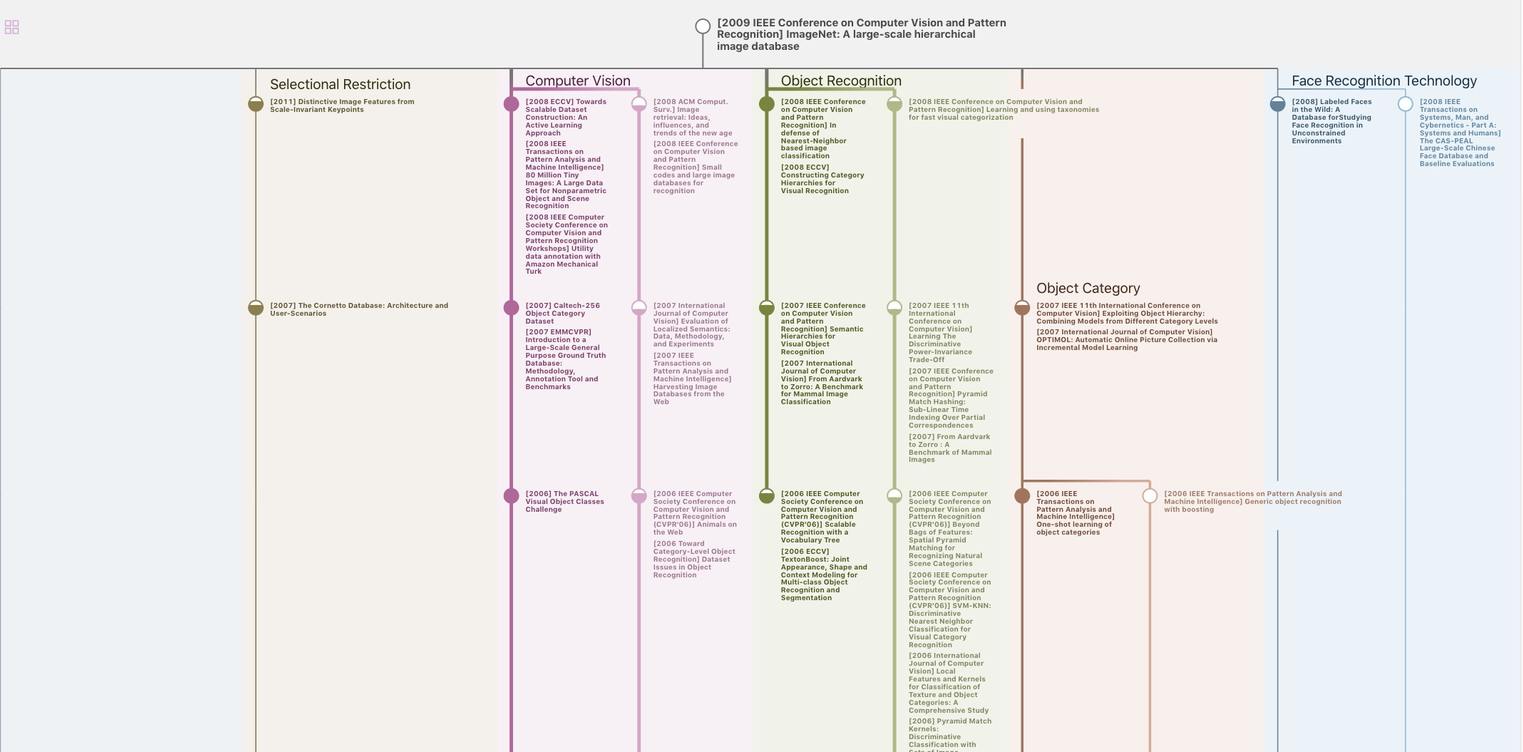
生成溯源树,研究论文发展脉络
Chat Paper
正在生成论文摘要