Mining non-redundant closed flexible periodic patterns.
Engineering Applications of Artificial Intelligence(2018)
Abstract
Mining periodic patterns from time series databases is needed to predict any future situation. Flexible pattern mining is a special kind of periodic pattern mining where intermediate events can be overlooked purposely. Mining such patterns from time series data is advantageous due to its capability of modeling various real life scenarios. The goal of mining closed flexible patterns is to avoid unnecessary flexible patterns but preserving the same information of a complete set of patterns. Though it has wide range of application domains, existing algorithms failed to mine closed flexible patterns without generating any false positive, i.e. non-closed and ∕or redundant patterns. In this paper, a new algorithm NRCFP (Non-Redundant Closed Flexible Pattern) has been proposed that generates complete set of non-redundant closed flexible patterns in time series databases. Three pruning techniques- BackScan (existing), RangeScan (proposed) and Column-pruning (proposed) have been applied to avoid generation of non-closed patterns, redundant flexible patterns and fictitious patterns. Proposed NRCFP efficiently mines non-redundant closed flexible periodic patterns. The performance of our algorithm has been extensively analyzed using several real-life databases based on runtime and memory consumption and compared with existing state-of-the-arts approach to prove effectiveness of the algorithm with respect to required processing time and memory consumption. Some applications of our proposed algorithm in various real life domains are discussed.
MoreTranslated text
Key words
Data mining,Time series databases,Periodic patterns,Closed periodic patterns,Flexible patterns,Non-redundant patterns
AI Read Science
Must-Reading Tree
Example
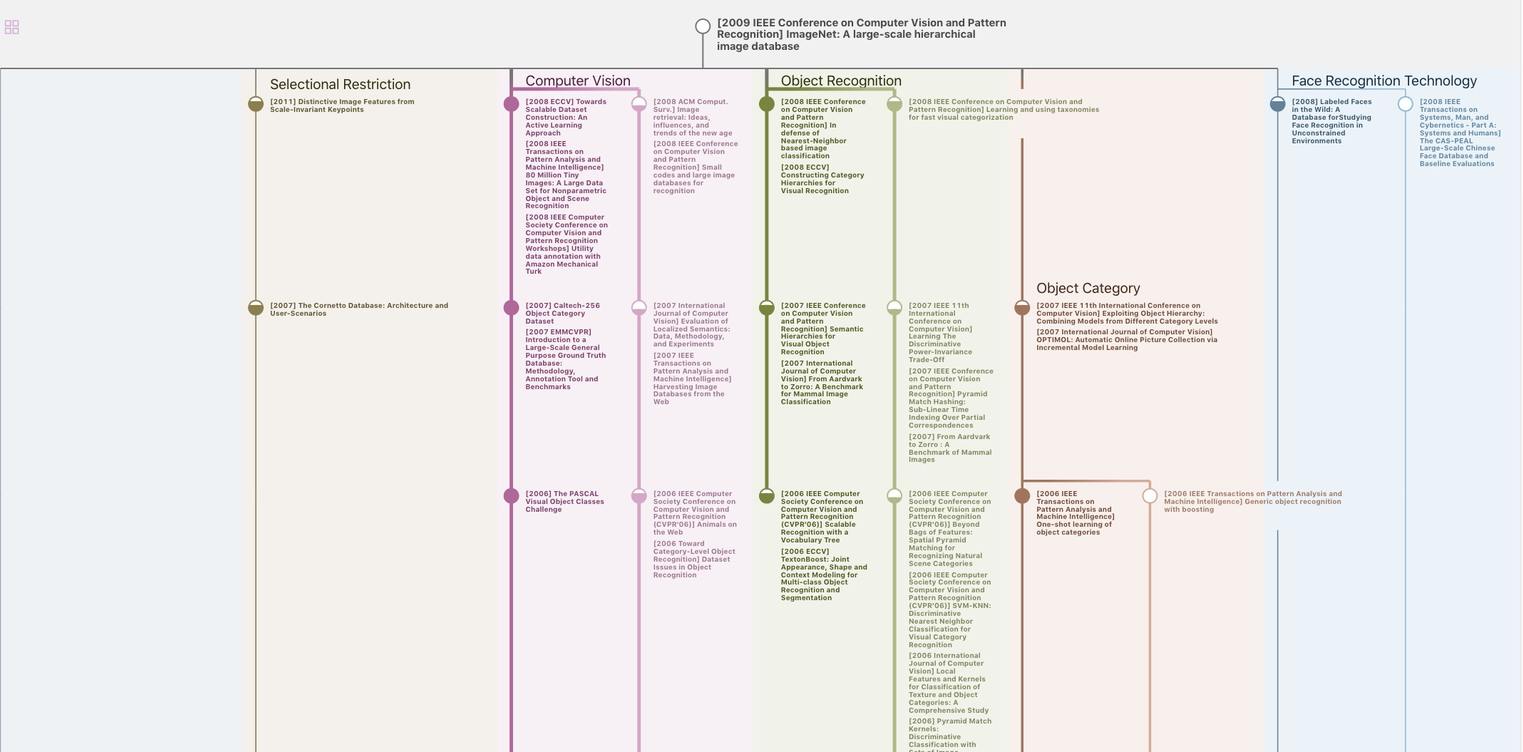
Generate MRT to find the research sequence of this paper
Chat Paper
Summary is being generated by the instructions you defined