Complex Process Monitoring Using KUCA With Application to Treatment of Waste Liquor.
IEEE Trans. Contr. Sys. Techn.(2018)
Abstract
In this paper, a new nonlinear process modeling method called kernel uncorrelated component analysis (KUCA) is proposed. Several advantages of KUCA are summarized as follows. First, KUCA is superior to traditional data-driven modeling methods such as principal component analysis, independent component analysis, and so on, for it has no constraints on data distribution and data structure. In other word, it can be applied to modeling non-Gaussian and nonlinear process. Second, the proposed KUCA allows us to extract uncorrelated components (UCs) from observations directly without considering the second-order or higher order statistic, which is of low computational load. In addition, KUCA is applied to a numerical example to evaluate its performance of source signal separation. Finally, on the basis of KUCA, the corresponding processes monitoring scheme is developed, and the hot galvanizing pickling waste liquor treatment process is taken to evaluate the monitoring performance of the proposed methods. Experiment results demonstrate the feasibility of the proposed monitoring scheme.
MoreTranslated text
Key words
Monitoring,Kernel,Principal component analysis,Signal processing algorithms,Integrated circuits,Heuristic algorithms,Data structures
AI Read Science
Must-Reading Tree
Example
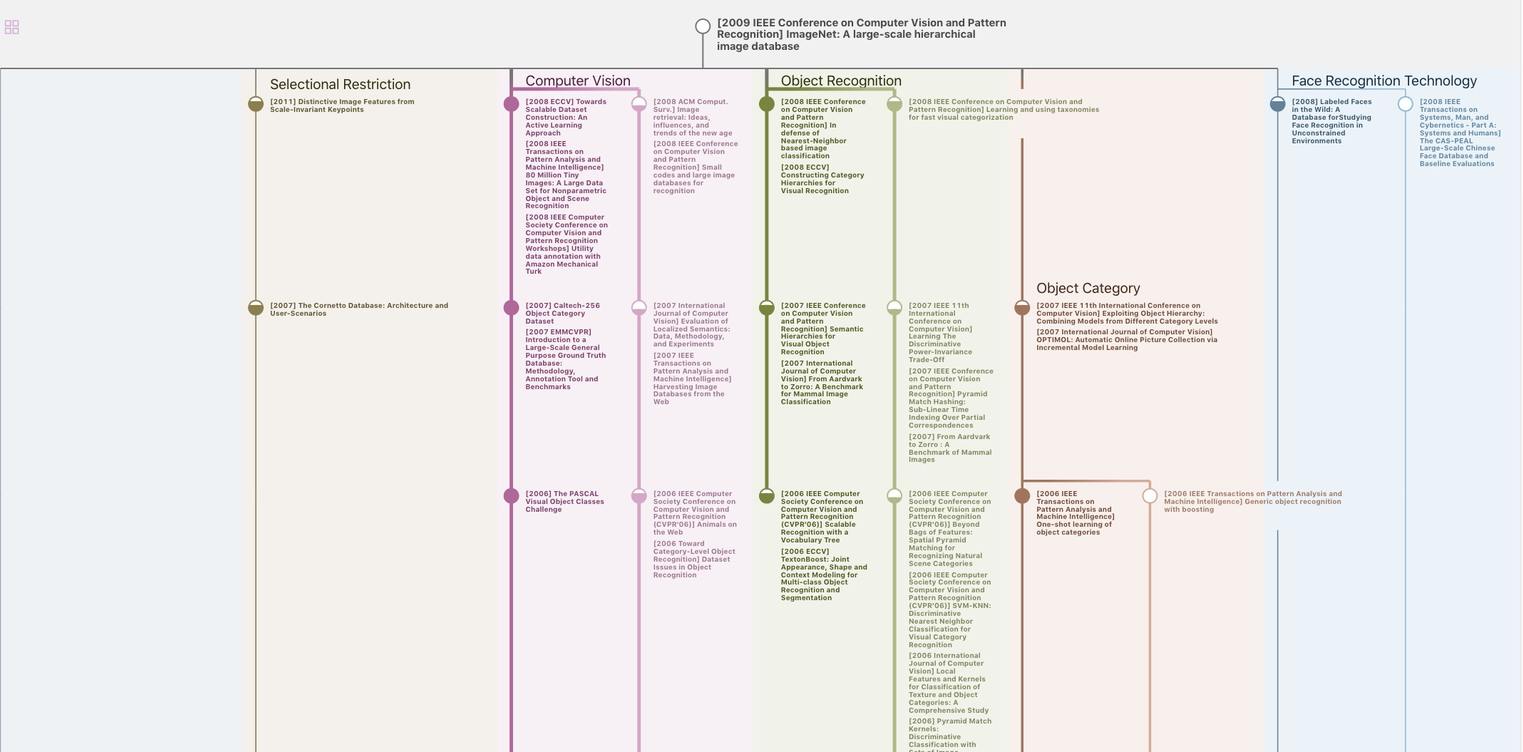
Generate MRT to find the research sequence of this paper
Chat Paper
Summary is being generated by the instructions you defined