Human Action Recognition Using Similarity Degree Between Postures And Spectral Learning
IET COMPUTER VISION(2018)
摘要
In recent years, there has been renewed interest in developing methods for skeleton-based human action recognition. In this study, the challenging problem of the similarity degree of skeleton-based human postures is addressed. Human posture is described by screw motions between 3D rigid bodies, which can be seen as a relation matrix of 3D rigid bodies (RMRB3D). A linear subspace, a point of a Grassmannian manifold, is spanned by the orthonormal basis of matrix RMRB3D. A powerful way to compute the similarity degree between postures is researched to solve the geodesic distance between points on the Grassmannian manifold. Then representative postures are extracted through spectral clustering over representative postures. An action will be represented by a symbol sequence generated with a global linear eigenfunction constructed by spectral embedding. Finally, dynamic time warping and hidden Markov model (HMM) are used to classify these action sequences. The experimental evaluations of the proposed method on several challenging 3D action datasets show that the proposed approaches achieve promising results compared with other skeleton-based human action recognition algorithms.
更多查看译文
关键词
feature extraction,matrix algebra,eigenvalues and eigenfunctions,image motion analysis,learning (artificial intelligence),pattern clustering,image sequences,hidden Markov models,image recognition,human action recognition,spectral learning,skeleton-based human action recognition,skeleton-based human posture similarity degree,screw motions,relation matrix of 3D rigid bodies,Grassmannian manifold,matrix RMRB3D orthonormal basis,geodesic distance,spectral clustering,representative postures,symbol sequence,global linear eigenfunction,spectral embedding,dynamic time warping,hidden Markov model,HMM,action sequences
AI 理解论文
溯源树
样例
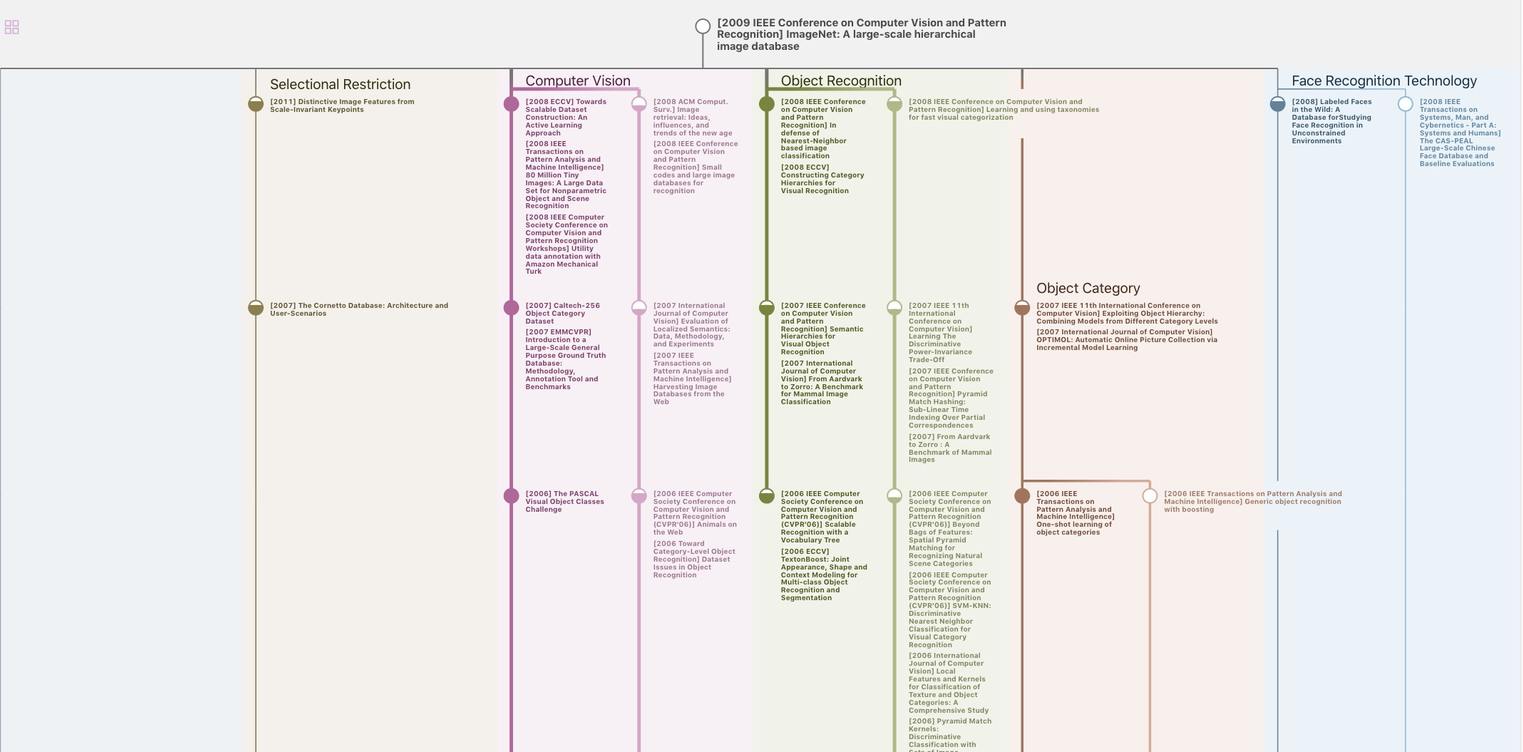
生成溯源树,研究论文发展脉络
Chat Paper
正在生成论文摘要