Review Of Disruption Predictors In Nuclear Fusion: Classical, From Scratch And Anomaly Detection Approaches
PROCEEDINGS OF THE IECON 2016 - 42ND ANNUAL CONFERENCE OF THE IEEE INDUSTRIAL ELECTRONICS SOCIETY(2016)
摘要
Disruption predictors are implemented as data-driven models obtained from machine learning techniques. These data-driven models are deduced from a training process with thousands of discharges (both disruptive and non-disruptive). ITER or DEMO, the next step devices cannot afford to wait for hundreds of disruptions to start predicting. A novelty approach for disruption prediction is to avoid the use of past discharges for learning purposes. The objective is to learn in every discharge how a safe plasma evolution is and to trigger an alarm when anomalies in the data flow appear. Of course, these anomalies have to be signatures of the phenomenon's precursors. By applying these ideas in JET to a dataset of more than 1700 non-disruptive shots and more than 550 that ended in a disruption, the success rate is about 90% and the false alarm rate is slightly above 7%. On average, the alarm is triggered with an anticipation time above 200 ms.
更多查看译文
关键词
disruption prediction,anomaly detection,nuclear fusion
AI 理解论文
溯源树
样例
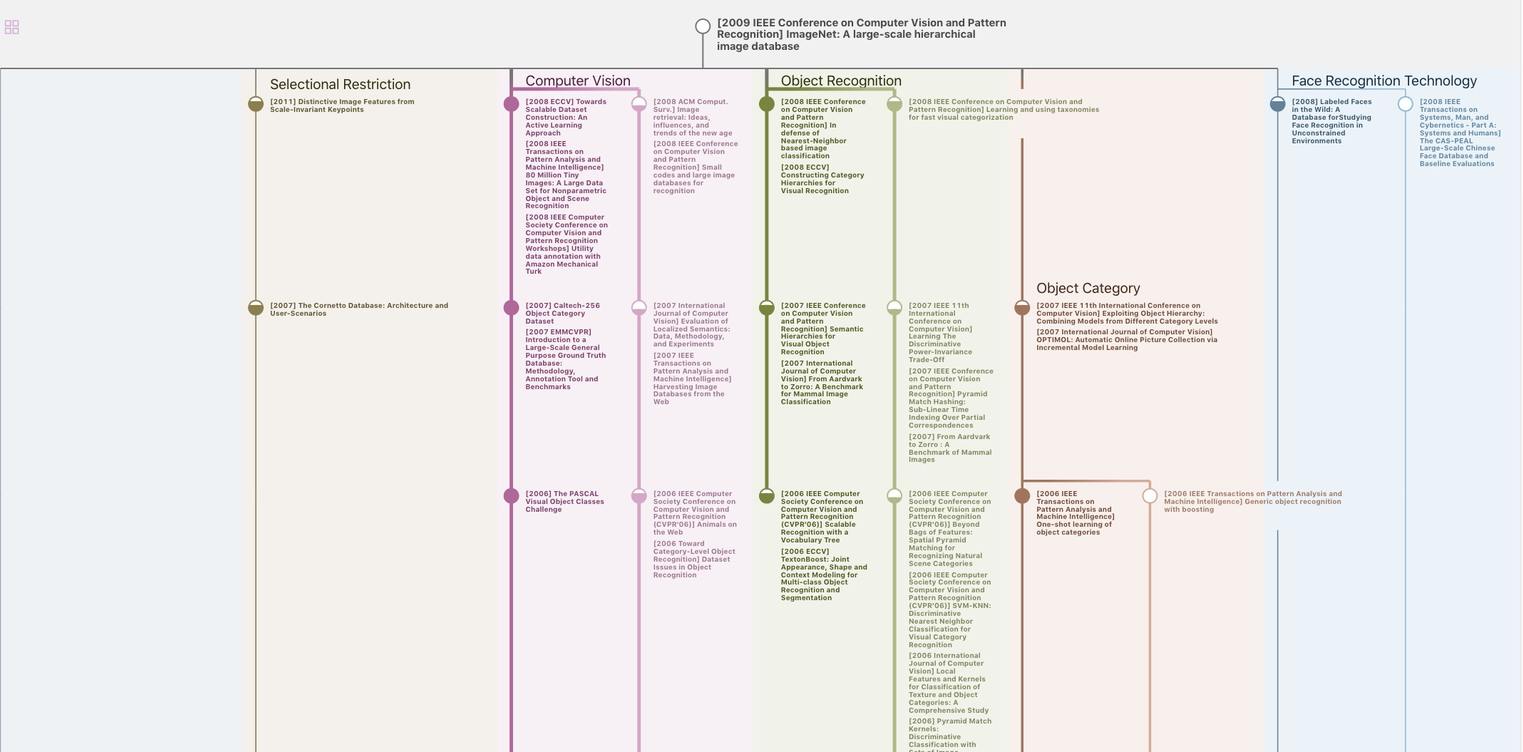
生成溯源树,研究论文发展脉络
Chat Paper
正在生成论文摘要