Application Of Integral Reinforcement Learning For Optimal Control Of A High Speed Flux-Switching Permanent Magnet Machine
PROCEEDINGS OF THE IECON 2016 - 42ND ANNUAL CONFERENCE OF THE IEEE INDUSTRIAL ELECTRONICS SOCIETY(2016)
摘要
A novel control method using H-infinity tracking and integral reinforcement learning is applied to a flux-switching permanent magnet (FSPM) machine in a hostile environment. The proposed controller can maintain high performance in the presence of motor parameter uncertainties and load disturbances. The conventional design procedure for an H-infinity controller is to solve the Hamilton-Jacobi-Isaacs (HJI) equation which requires full information of the system model. The novel control method, the integral reinforcement learning (IRL) makes use of neural networks to parametrically represent the control policy and the performance of system, and learns the solution of HJI equations online. Therefore, the FSPM machine can work optimally under parameter uncertainties due to different operating conditions. The simulation in Matlab/Simulink vividly 9000 rpm, 12/5 poles flux-switching permanent magnet machine.
更多查看译文
关键词
integral reinforcement learning,optimal control,high speed flux-switching permanent magnet machine,H∞ tracking,FSPM machine,motor parameter uncertainties,load disturbances,H∞ controller design,Hamilton-Jacobi-Isaacs equation,HJI equation,IRL,neural networks,Matlab,Simulink,rotor speed,poles flux-switching permanent magnet machine
AI 理解论文
溯源树
样例
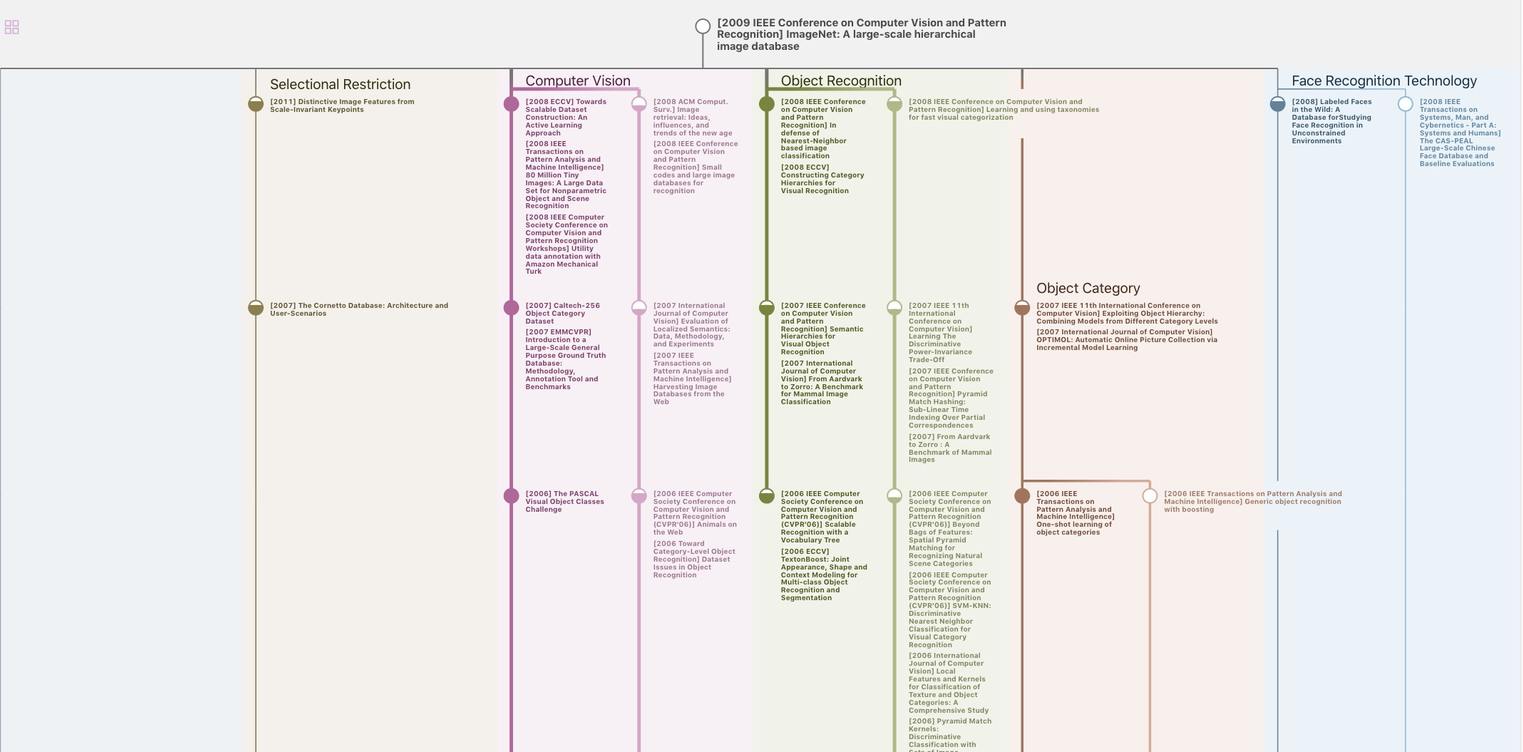
生成溯源树,研究论文发展脉络
Chat Paper
正在生成论文摘要