Qualitative data analysis of disaster risk reduction suggestions assisted by topic modeling and word2vec
2017 International Conference on Asian Language Processing (IALP)(2017)
摘要
In this study, we examine suggestions for disaster risk reduction strategies provided by residents in selected disaster-prone areas in the Philippines. The study utilizes 976 suggestions on how their barangay can help them better prepare for a disaster. These were collected through Malasakit, an e-participation platform designed by University of California, Berkeley and National University (Philippines) to engage community participation in gathering qualitative and quantitative data. Analyses were conducted through biterm topic modeling (BTM) and word embedding using gensim. For better accuracy, data preprocessing was performed to remove irrelevant or noisy data. Based on the BTM result, we identified the following important codes: preparedness, disaster, awareness, community, help, seminars, kanal (canal), linisin (clean), drainage, garbage, basura (garbage). Analyses of the topic models show that disaster preparedness is an integral part in disaster risk reduction by improving solid waste management, providing seminars for public awareness and evacuation preparation. A word intrusion test was conducted where BTM scored 55.71% which implies strong cohesion of the words with their topics. For word embedding, we drilled down on the following words: community, preparedness, emergency, barangay (village), help, kanal (drainage), basura (garbage), awareness, seminars, information. The word2vec results has a cosine similarity score of 0.902 which implies strong relatedness of each word. The result shows that the participants give importance to community preparedness for emergency, helping the barangay in clean-up drive, and awareness through seminars and information dissemination.
更多查看译文
关键词
word embedding,biterm topic modeling,gensim,scikit learn,Malasakit toolkit,disaster risk reduction
AI 理解论文
溯源树
样例
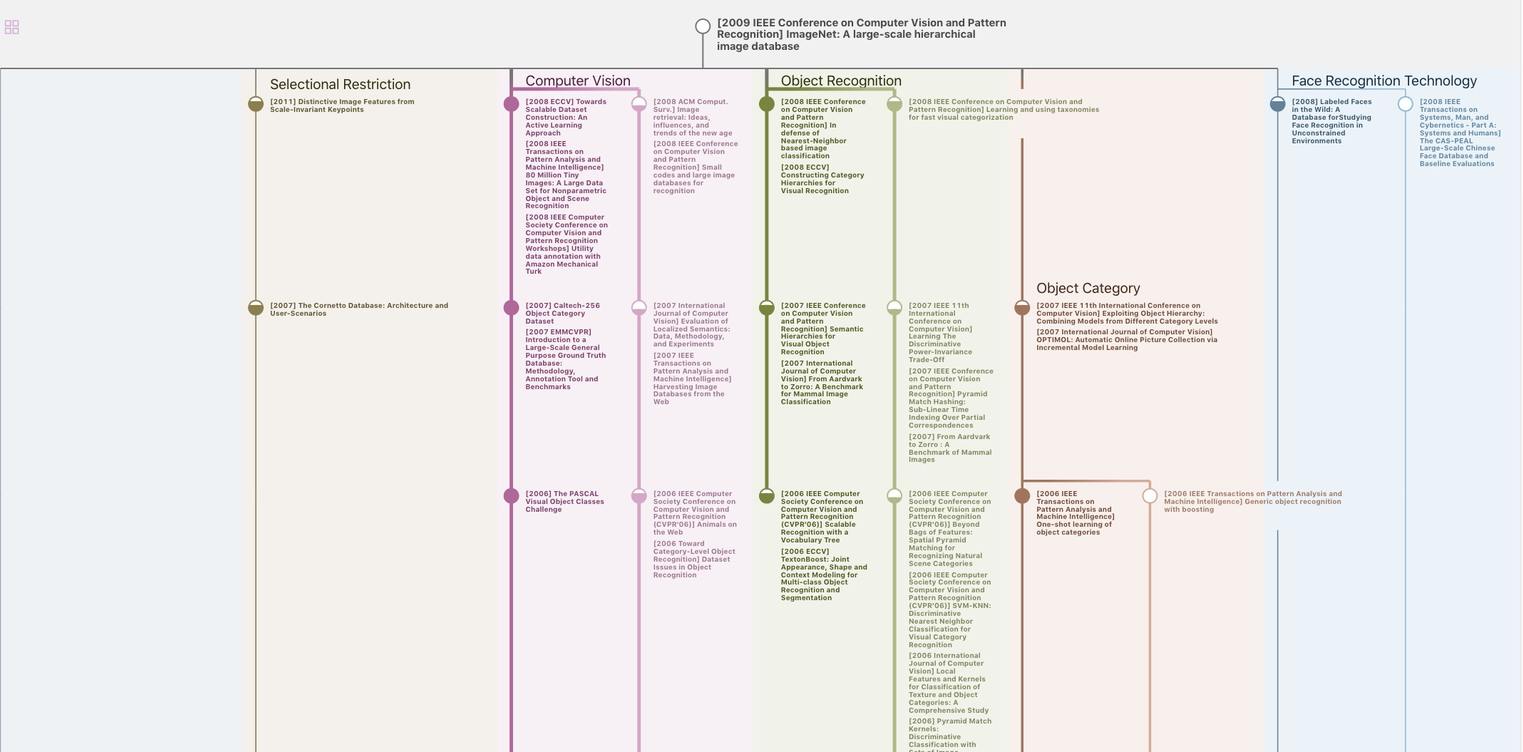
生成溯源树,研究论文发展脉络
Chat Paper
正在生成论文摘要