Sketch 'Em All: Fast Approximate Similarity Search for Dynamic Data Streams.
WSDM 2018: The Eleventh ACM International Conference on Web Search and Data Mining Marina Del Rey CA USA February, 2018(2018)
摘要
Recommender systems are an integral part of many web applications. With increasingly larger user bases, scalability has become an important issue. Many of the most scalable algorithms with respect to both space and running times are based on locality sensitive hashing. However, a significant drawback is that these methods are only able to handle insertions to user profiles and tend to perform poorly when items may be removed. We initiate the study of scalable locality sensitive hashing (LSH) for dynamic input. Specifically, using the Jaccard index as similarity measure, we design (1) a sketching algorithm for similarity estimation via a black box reduction to $\ell_0$ norm estimation and (2) a locality sensitive hashing scheme maintainable in fully dynamic data streams that quickly filters out low-similarity pairs. Our algorithms have little to no overhead in terms of running time compared to previous LSH approaches for the insertion only case, and drastically outperform previous algorithms in case of deletions.
更多查看译文
关键词
locality sensitive hashing, dynamic data streams, Jaccard index
AI 理解论文
溯源树
样例
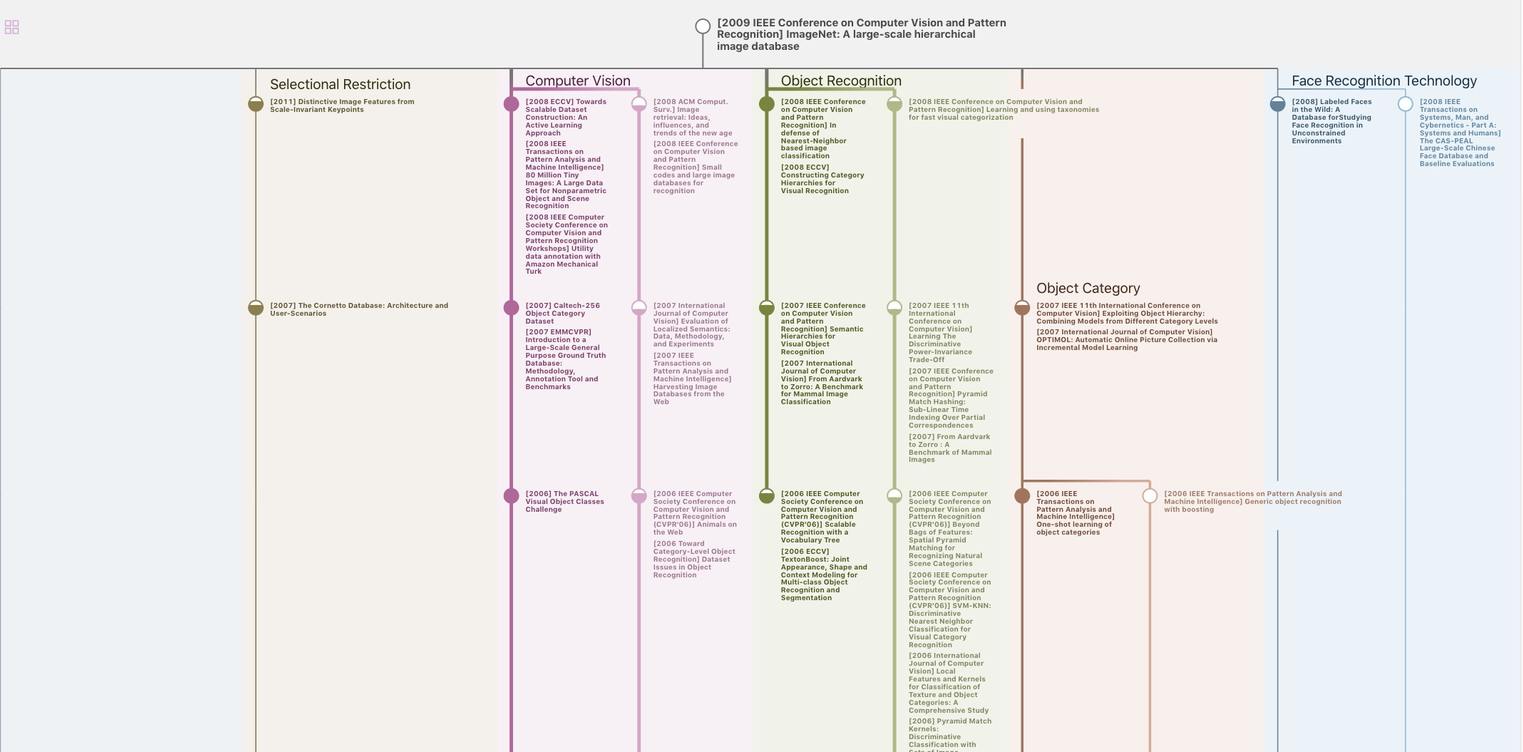
生成溯源树,研究论文发展脉络
Chat Paper
正在生成论文摘要