Learning Affordance for Autonomous Driving.
MobiCom '17: The 23rd Annual International Conference on Mobile Computing and Networking Snowbird Utah USA October, 2017(2017)
摘要
Today, there are two major paradigms for vision-based autonomous driving systems: mediated perception approaches that parse an entire scene to make a driving decision, and behavior reflex approaches that directly map an input image to a driving action by a regressor. In this paper, we propose a third paradigm: a direct perception based approach to estimate the affordance for driving. We propose to map an input image to a small number of key perception indicators that directly relate to the affordance of a road/traffic state for driving. Our representation provides a set of compact yet complete descriptions of the scene to enable a simple controller to drive autonomously. Falling in between the two extremes of mediated perception and behavior reflex, we argue that our direct perception representation provides the right level of abstraction. We evaluate our approach in a virtual racing game as well as real world driving and show that our model can work well to drive a car in a very diverse set of virtual and realistic environments.
更多查看译文
关键词
Autonomous driving, self-driving car
AI 理解论文
溯源树
样例
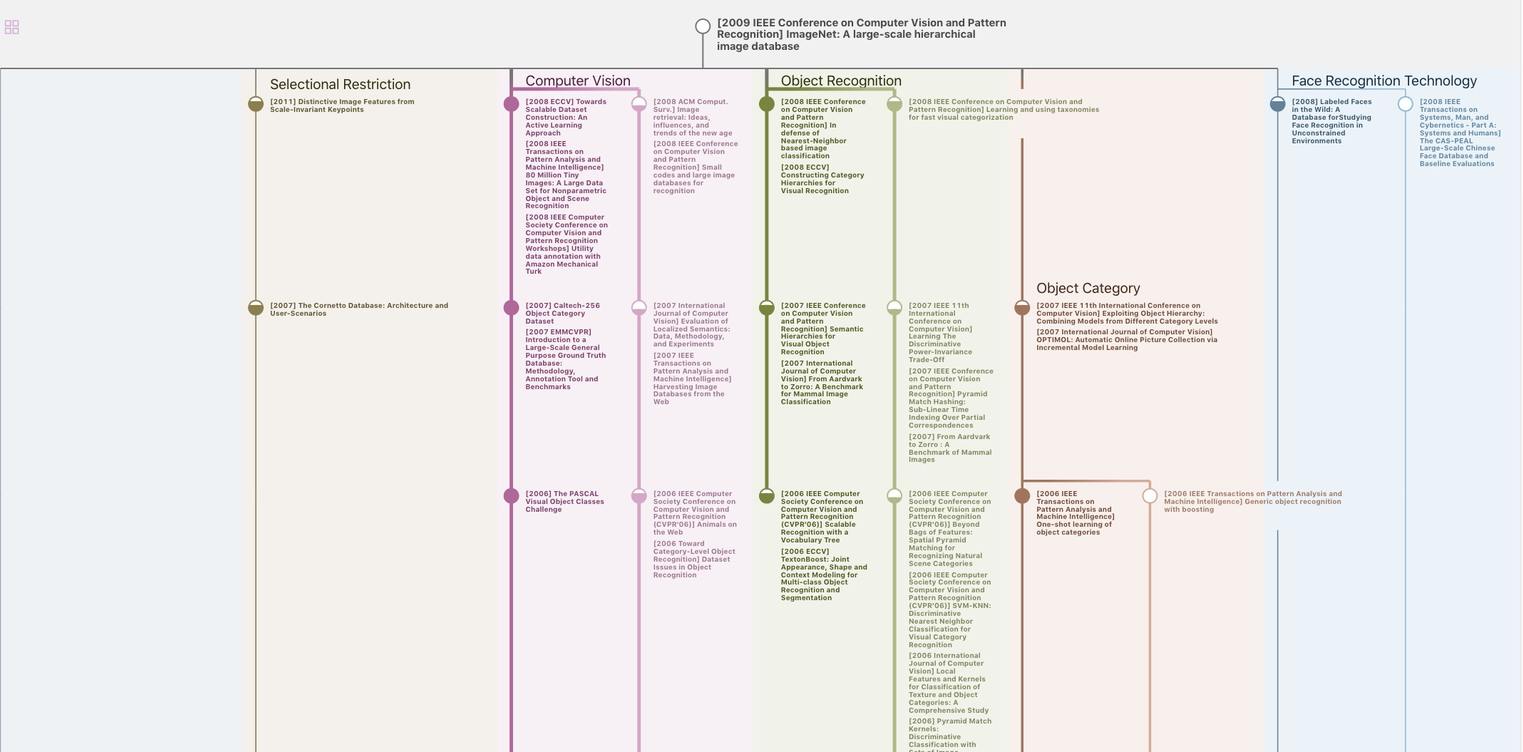
生成溯源树,研究论文发展脉络
Chat Paper
正在生成论文摘要