Using Convolutional Encoder-Decoder For Document Image Binarization
2017 14TH IAPR INTERNATIONAL CONFERENCE ON DOCUMENT ANALYSIS AND RECOGNITION (ICDAR), VOL 1(2017)
摘要
Document image binarization is one of the critical initial steps for document analysis and understanding. Previous work mostly focused on exploiting hand-crafted features to build statistical models for distinguishing text from background. However, these approaches only achieved limited success because: (a) the effectiveness of hand-crafted features is limited by the researcher's domain knowledge and understanding on the documents, and (b) a universal model cannot always capture the complexity of different document degradations. In order to address these challenges, we propose a convolutional encoder-decoder model with deep learning for document image binarization in this paper. In the proposed method, mid-level document image representations are learnt by a stack of convolutional layers, which compose the encoder in this architecture. Then the binarization image is obtained by mapping low resolution representations to the original size through the decoder, which is composed by a series of transposed convolutional layers. We compare the proposed binarization method with other binarization algorithms both qualitatively and quantitatively on the public dataset. The experimental results show that the proposed method has comparable performance to the other hand-crafted binarization approaches and has more generalization capabilities with limited in-domain training data.
更多查看译文
AI 理解论文
溯源树
样例
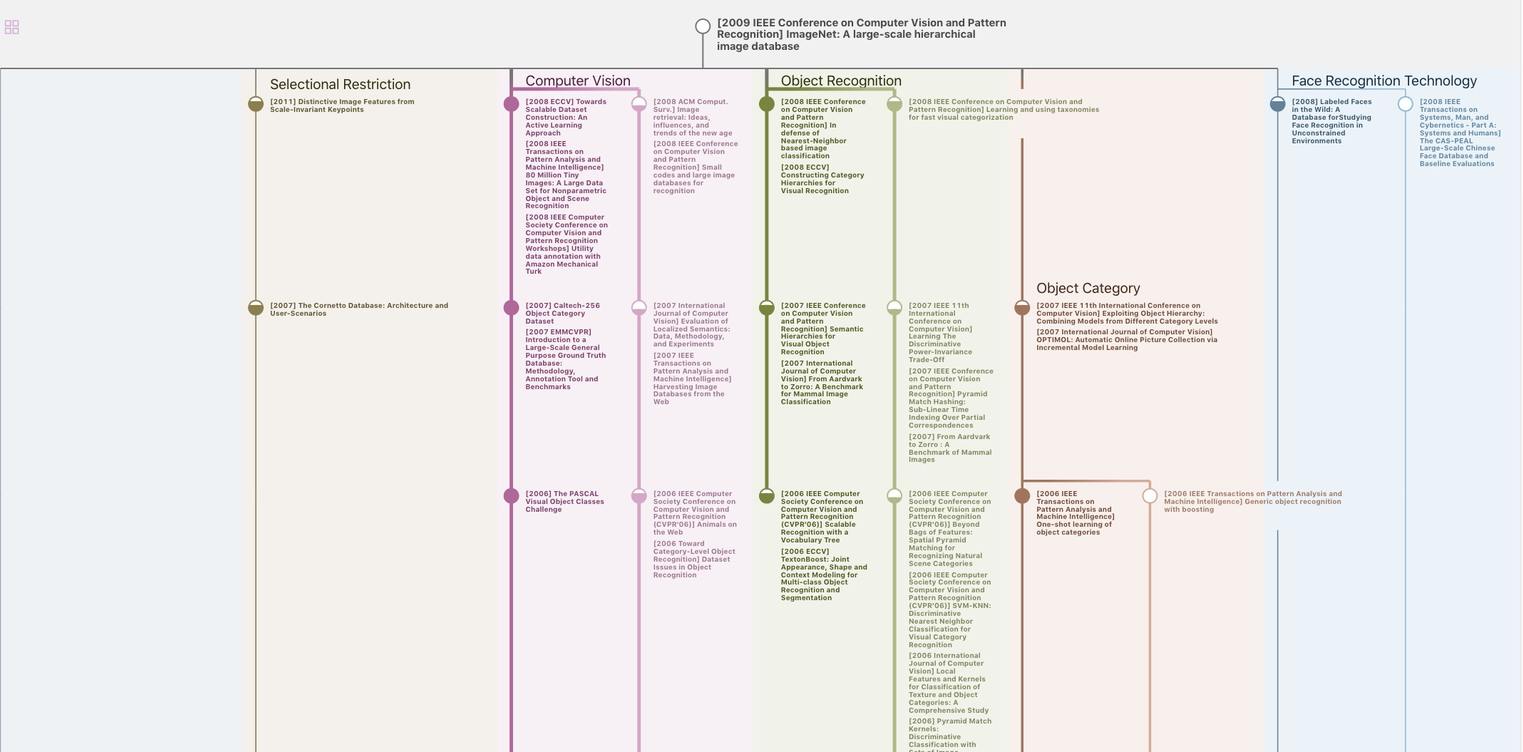
生成溯源树,研究论文发展脉络
Chat Paper
正在生成论文摘要