Computationally efficient deep tracker: Guided MDNet.
National Conference on Communications NCC(2017)
摘要
The main objective of the paper is to recommend an essential improvement to the existing Multi-Domain Convolutional Neural Network tracker (MDNet) which is used to track unknown object in a video-stream. MDNet is able to handle major basic tracking challenges like fast motion, background clutter, out of view, scale variations etc. through offline training and online tracking. We pre-train the Convolutional Neural Network (CNN) offline using many videos with ground truth to obtain a target representation in the network. In online tracking the MDNet uses large number of random sample of windows around the previous target for estimating the target in the current frame which make its tracking computationally complex while testing or obtaining the track. The major contribution of the paper is to give guided samples to the MDNet rather than random samples so that the computation and time required by the CNN while tracking could be greatly reduced. Evaluation of the proposed algorithm is done using the videos from the ALOV300++ dataset and the VOT dataset and the results are compared with the state of art trackers.
更多查看译文
关键词
computationally efficient deep tracker,guided MDNet,track unknown object,video-stream,fast motion,background clutter,scale variations,offline training,online tracking,CNN,ground truth,target representation,guided samples,random samples,Convolutional Neural Network,ALOV300++ dataset,VOT dataset,MultiDomain Convolutional Neural Network tracker
AI 理解论文
溯源树
样例
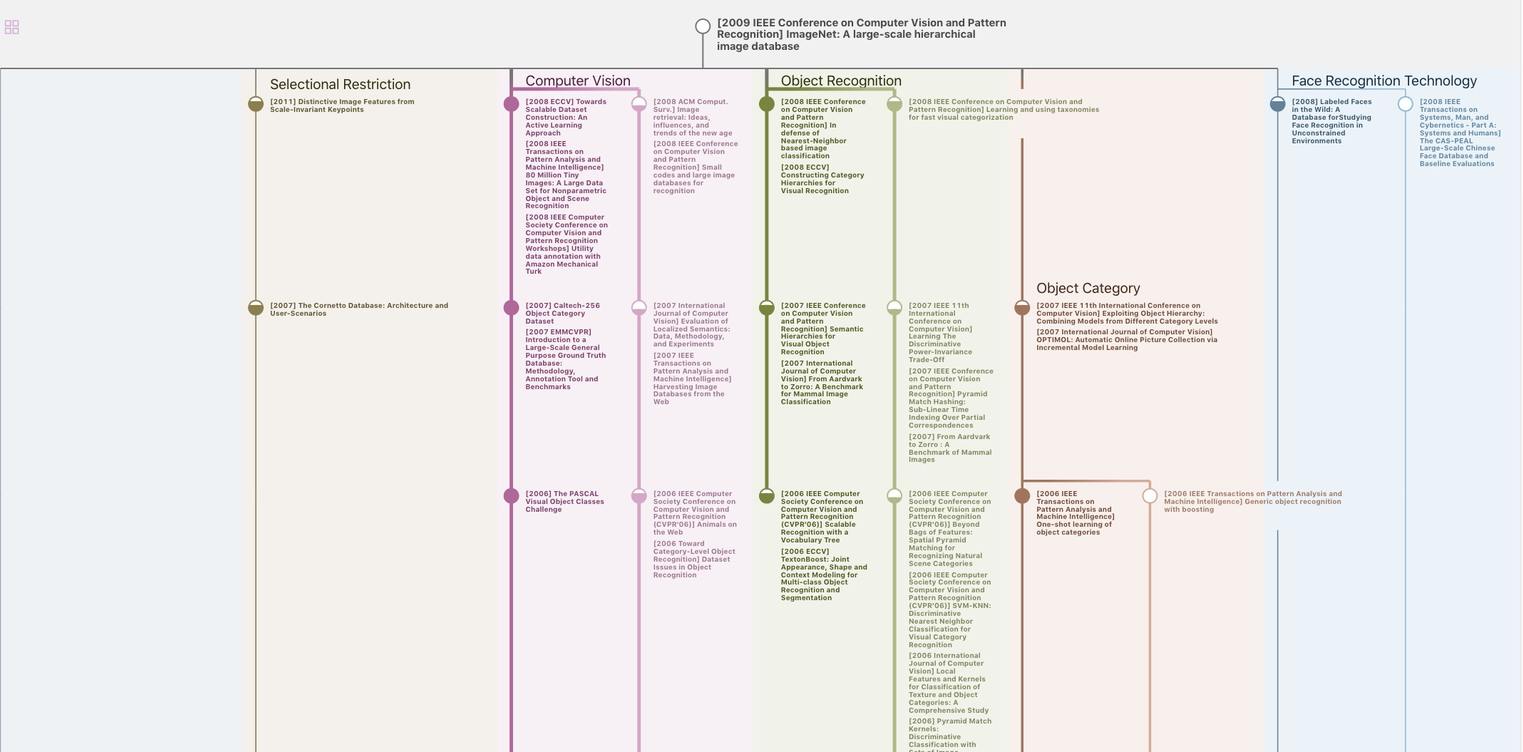
生成溯源树,研究论文发展脉络
Chat Paper
正在生成论文摘要