Multi-Layer Linear Model For Top-Down Modulation Of Visual Attention In Natural Egocentric Vision
2017 24TH IEEE INTERNATIONAL CONFERENCE ON IMAGE PROCESSING (ICIP)(2017)
摘要
Top-down attention plays an important role in guidance of human attention in real-world scenarios, but less efforts in computational modeing of visual attention has been put on it. Inspired by the mechanisms of top-down attention in human visual perception, we propose a multi-layer linear model of top-down attention to modulate bottom-up saliency maps actively. The first layer is a linear regression model which combines the bottom-up saliency maps on various visual features and objects. A contextual dependent upper layer is introduced to tune the parameters of the lower layer model adaptively. Finally, a mask of selection history is applied to the fused attention map to bias the attention selection towards the task related regions. Efficient learning algorithm with single-pass polynomial complexity is derived. We evaluate our model on a set of natural egocentric videos captured from a wearable glass in real-world environments. Our model outperforms the baseline and state-of-the-art bottom-up saliency models.
更多查看译文
关键词
ego-centric, visual attention, real-world
AI 理解论文
溯源树
样例
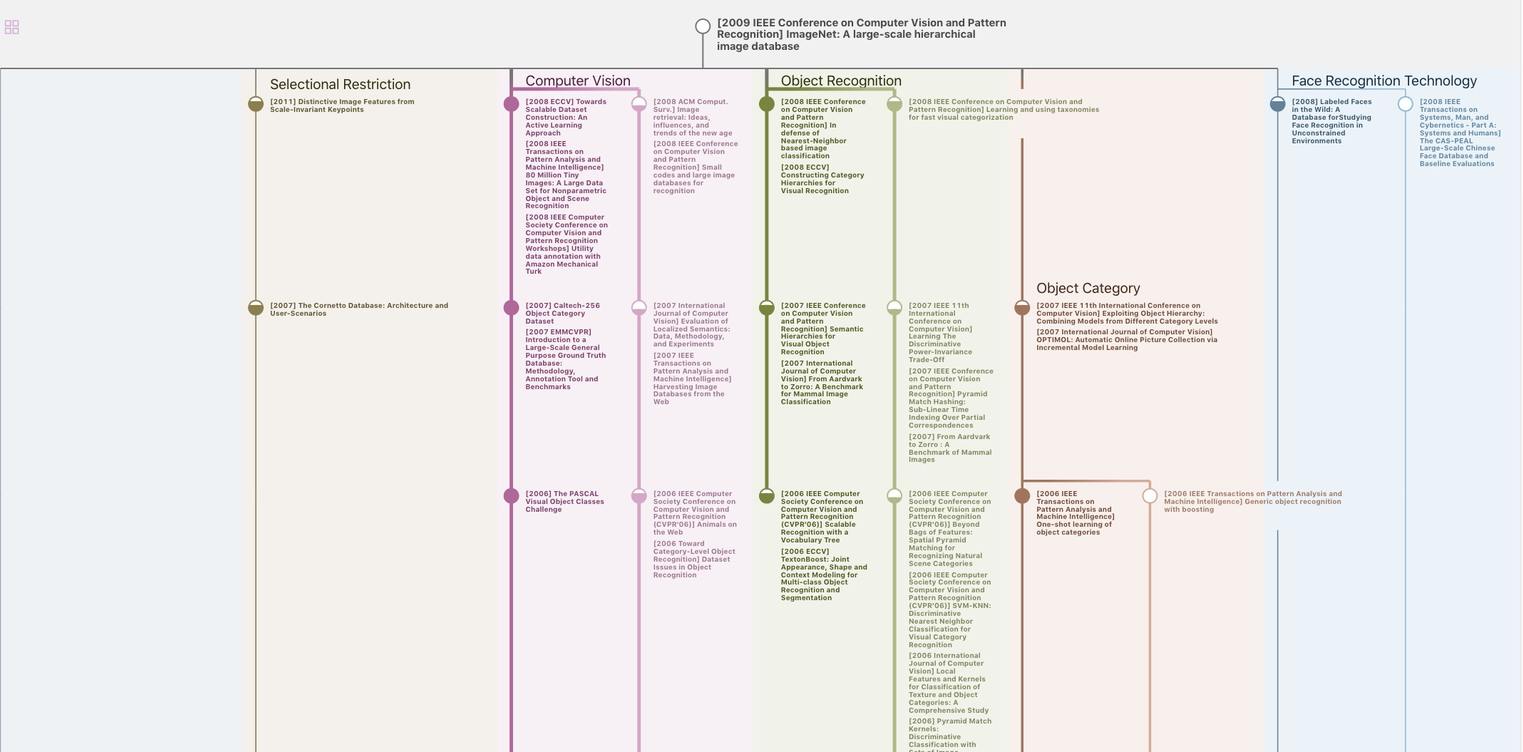
生成溯源树,研究论文发展脉络
Chat Paper
正在生成论文摘要