Joint coarse-and-fine reasoning for deep optical flow
2017 24TH IEEE INTERNATIONAL CONFERENCE ON IMAGE PROCESSING (ICIP)(2017)
摘要
We propose a novel representation for dense pixel-wise estimation tasks using CNNs that boosts accuracy and reduces training time, by explicitly exploiting joint coarse-and-fine reasoning. The coarse reasoning is performed over a discrete classification space to obtain a general rough solution, while the fine details of the solution are obtained over a continuous regression space. In our approach both components are jointly estimated, which proved to be beneficial for improving estimation accuracy. Additionally, we propose a new network architecture, which combines coarse and fine components by treating the fine estimation as a refinement built on top of the coarse solution, and therefore adding details to the general prediction. We apply our approach to the challenging problem of optical flow estimation and empirically validate it against state-of-the-art CNN-based solutions trained from scratch and tested on large optical flow datasets.
更多查看译文
关键词
optical flow, convolutional neural networks, regression, classification, flownet, coarse-and-fine
AI 理解论文
溯源树
样例
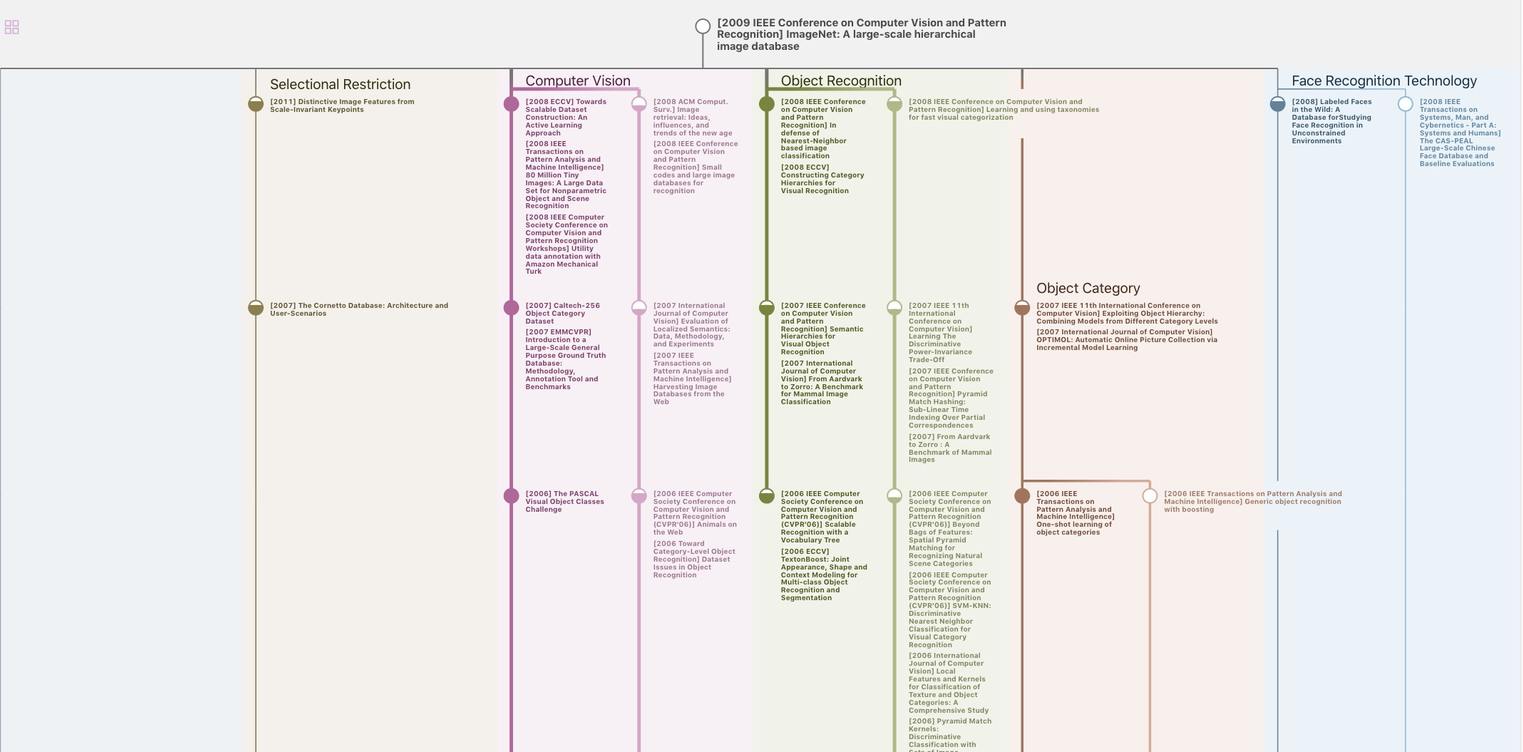
生成溯源树,研究论文发展脉络
Chat Paper
正在生成论文摘要