Extragradient Method in Optimization: Convergence and Complexity
J. Optimization Theory and Applications(2017)
Abstract
We consider the extragradient method to minimize the sum of two functions, the first one being smooth and the second being convex. Under the Kurdyka–Łojasiewicz assumption, we prove that the sequence produced by the extragradient method converges to a critical point of the problem and has finite length. The analysis is extended to the case when both functions are convex. We provide, in this case, a sublinear convergence rate, as for gradient-based methods. Furthermore, we show that the recent small-prox complexity result can be applied to this method. Considering the extragradient method is an occasion to describe an exact line search scheme for proximal decomposition methods. We provide details for the implementation of this scheme for the one-norm regularized least squares problem and demonstrate numerical results which suggest that combining nonaccelerated methods with exact line search can be a competitive choice.
MoreTranslated text
Key words
Extragradient,Descent method,Forward–Backward splitting,Kurdyka–Łojasiewicz inequality,Complexity, first-order method,LASSO problem
AI Read Science
Must-Reading Tree
Example
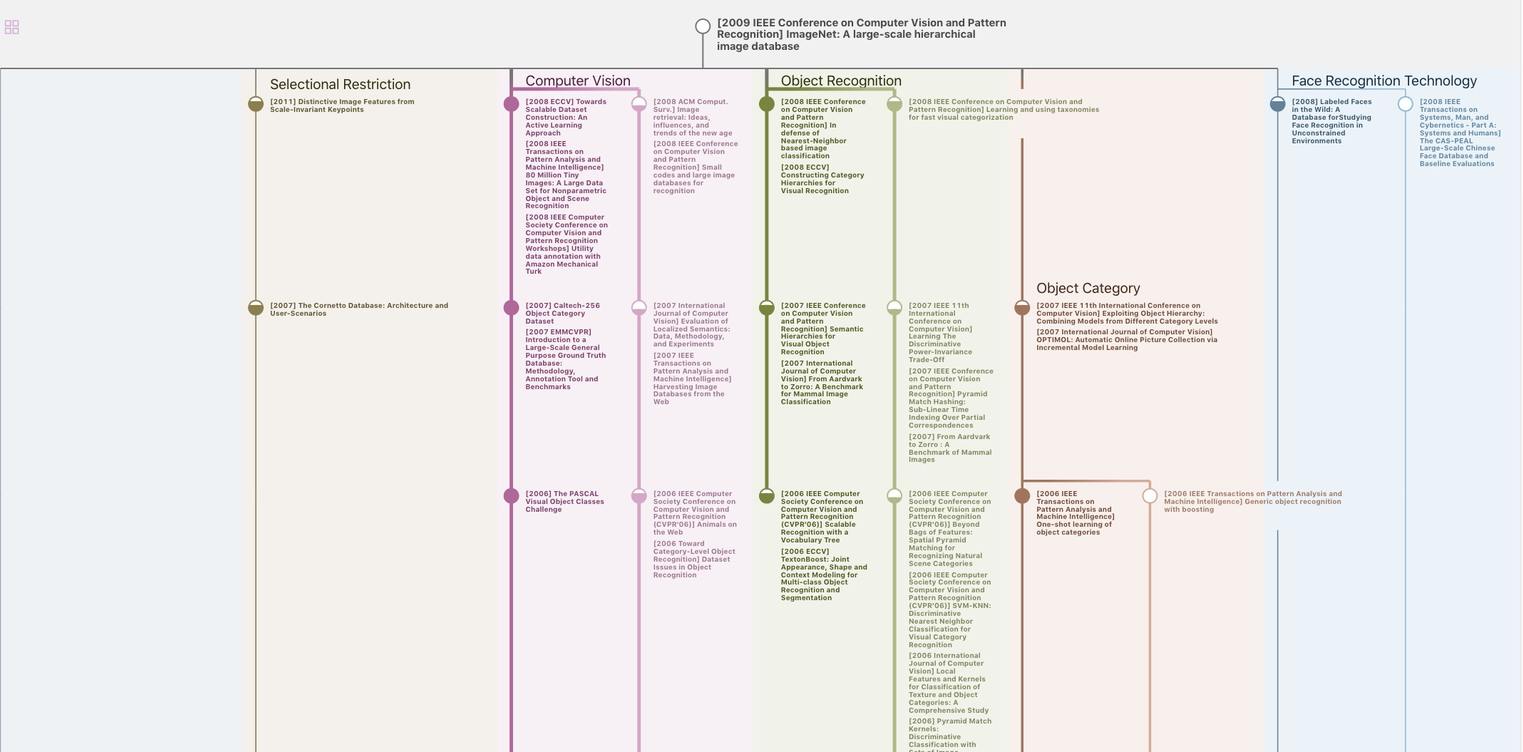
Generate MRT to find the research sequence of this paper
Chat Paper
Summary is being generated by the instructions you defined