Exponential convergence of testing error for stochastic gradient methods.
COLT(2018)
摘要
We consider binary classification problems with positive definite kernels and square loss, and study the convergence rates of stochastic gradient methods. We show that while the excess testing loss (squared loss) converges slowly to zero as the number of observations (and thus iterations) goes to infinity, the testing error (classification error) converges exponentially fast if low-noise conditions are assumed. To achieve these rates of convergence we show sharper high-probability bounds with respect to the number of observations for stochastic gradient descent.
更多查看译文
AI 理解论文
溯源树
样例
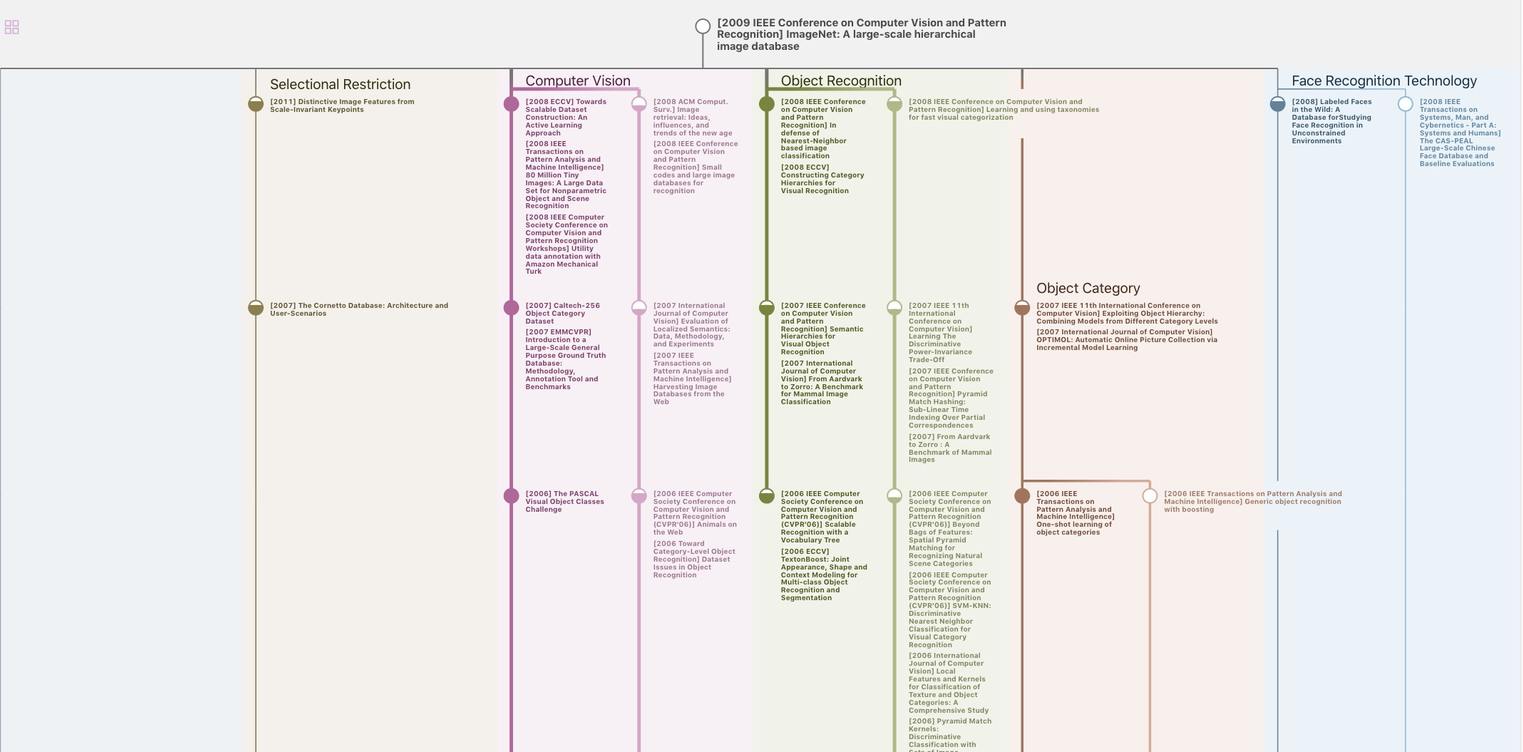
生成溯源树,研究论文发展脉络
Chat Paper
正在生成论文摘要