Online Sequential Learning Based on Extreme Learning Machines for Particulate Matter Forecasting
2017 Brazilian Conference on Intelligent Systems (BRACIS)(2017)
Abstract
Microscopically small solid particles and liquid droplets suspended in the air, known as particulate matter (PM), may significantly affect not only human health but also urban, natural and agricultural systems. Therefore, it is imperative to keep the concentration levels of these pollutants below harmful thresholds. Forecasting tools based on machine learning have been used to estimate the concentration of PM and other pollutants in the atmosphere. However, PM data are uninterruptedly collected over time, thus producing a stream of data whose distribution may evolve over time. As traditional machine learning techniques do not have mechanisms to handle changes on data distribution at running time, they usually present limited prediction accuracy when facing such scenario. The overall goal of this work is to evaluate whether online sequential learning can improve the estimation accuracy of PM forecasting. To do so, online and offline algorithms based on Extreme Learning Machines (ELM) were compared, in order to evaluate their performance when applied to forecast hourly concentrations of PM. The experiments were performed using real-world data streams of PM concentration from different cities of the State of São Paulo, Brazil. The obtained results show not only that online sequential learning approaches lead to smaller mean squared errors but also that the stability of the results is enhanced when such approaches are combined in ensembles.
MoreTranslated text
Key words
Particulate Matter,Machine Learning,Extreme Learning Machines,Data Streams
AI Read Science
Must-Reading Tree
Example
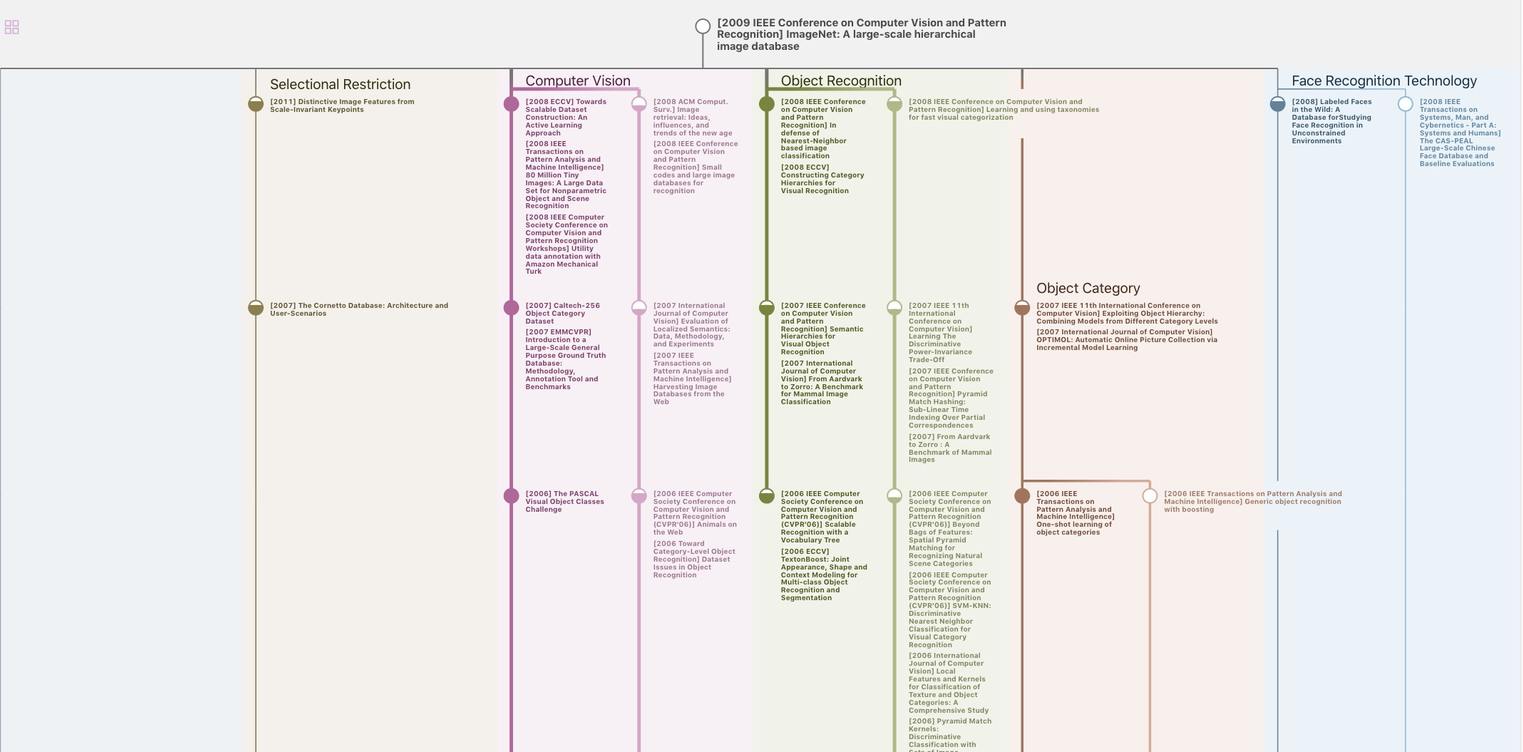
Generate MRT to find the research sequence of this paper
Chat Paper
Summary is being generated by the instructions you defined