Supercharging Crowd Dynamics Estimation in Disasters via Spatio-Temporal Deep Neural Network
2017 IEEE International Conference on Data Science and Advanced Analytics (DSAA)(2017)
Abstract
Accurate estimation of crowd dynamics is difficult, especially when it comes to fine-grained spatial and temporal predictions. A deep understanding of these fine-grained dynamics is crucial during a major disaster, as it guides efficient disaster managements. However, it is particularly challenging as these fine-grained dynamics are mainly caused by high-dimensional individual movement and evacuation. Furthermore, abnormal user behavior during disasters makes the problem of accurate prediction even more acute. Traditional models have difficulties in dealing with these high dimensional patterns caused by disruptive events. For example, the 2016 Kumamoto earthquakes disrupted normal crowd dynamics patterns significantly in the affected regions. We first perform a thorough analysis of a crowd population distribution dataset during Kumamoto earthquakes collected by a major mobile network operator in Japan, which shows strong fine-grained temporal autocorrelation and spatial correlation among geographically neighboring grids. It is also demonstrated that temporal autocorrelation during disasters is more than simple diurnal patterns. Moreover, there are many factors that could potentially influence spatial correlations and affect the dynamics patterns. Then, we illustrate how a spatial-temporal Long-Short-Term-Memory (LSTM) deep neural network could be applied to boost the prediction power. It is shown that the error in terms of Mean Square Error (MSE) is reduced by as much as 55.1-69.4% compared to regressive models such as AR, ARIMA and SVR. Furthermore, LSTM outperforms the aforementioned models significantly even when little training data is available right after the mainshock. Finally, we also show a Region-aware LSTM does not necessarily outperform a regular LSTM.
MoreTranslated text
Key words
Data Mining,Data-Driven Modeling,Disaster Management,Spatio-Temporal Dynamics,Deep Neural Network
AI Read Science
Must-Reading Tree
Example
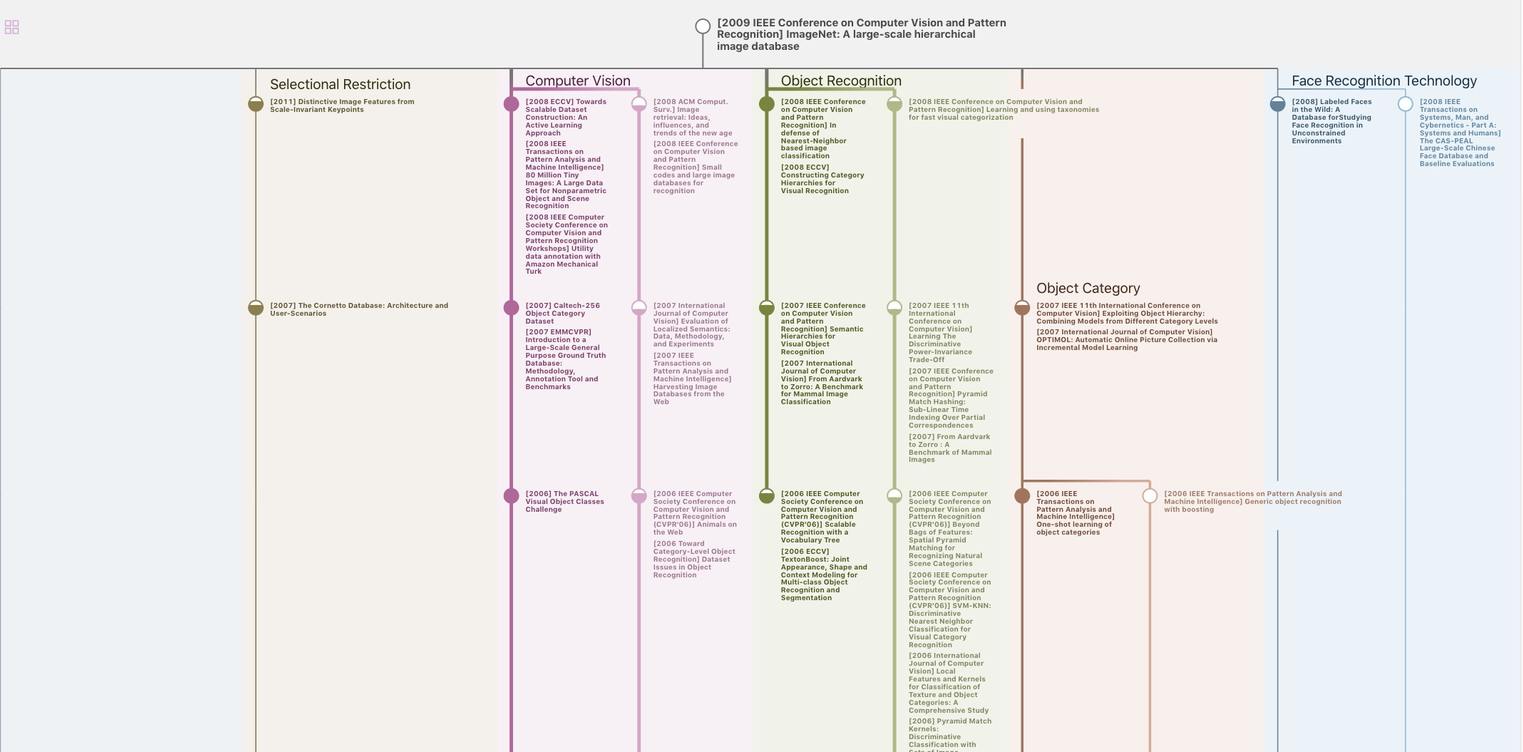
Generate MRT to find the research sequence of this paper
Chat Paper
Summary is being generated by the instructions you defined