Consensus Convolutional Sparse Coding
2017 IEEE INTERNATIONAL CONFERENCE ON COMPUTER VISION (ICCV)(2017)
摘要
Convolutional sparse coding (CSC) is a promising direction for unsupervised learning in computer vision. In contrast to recent supervised methods, CSC allows for convolutional image representations to be learned that are equally useful for high-level vision tasks and low-level image reconstruction and can be applied to a wide range of tasks without problem-specific retraining. Due to their extreme memory requirements, however, existing CSC solvers have so far been limited to low-dimensional problems and datasets using a handful of low-resolution example images at a time.In this paper, we propose a new approach to solving CSC as a consensus optimization problem, which lifts these limitations. By learning CSC features from large-scale image datasets for the first time, we achieve significant quality improvements in a number of imaging tasks. Moreover, the proposed method enables new applications in high-dimensional feature learning that has been intractable using existing CSC methods. This is demonstrated for a variety of reconstruction problems across diverse problem domains, including 3D multispectral demosaicing and 4D light field view synthesis.
更多查看译文
关键词
consensus convolutional sparse coding,unsupervised learning,computer vision,convolutional image representations,high-level vision tasks,low-level image reconstruction,problem-specific retraining,extreme memory requirements,CSC solvers,low-dimensional problems,low-resolution example images,consensus optimization problem,CSC features,large-scale image datasets,imaging tasks,high-dimensional feature learning,CSC methods,reconstruction problems,diverse problem domains,3D multispectral demosaicing,4D light field view synthesis
AI 理解论文
溯源树
样例
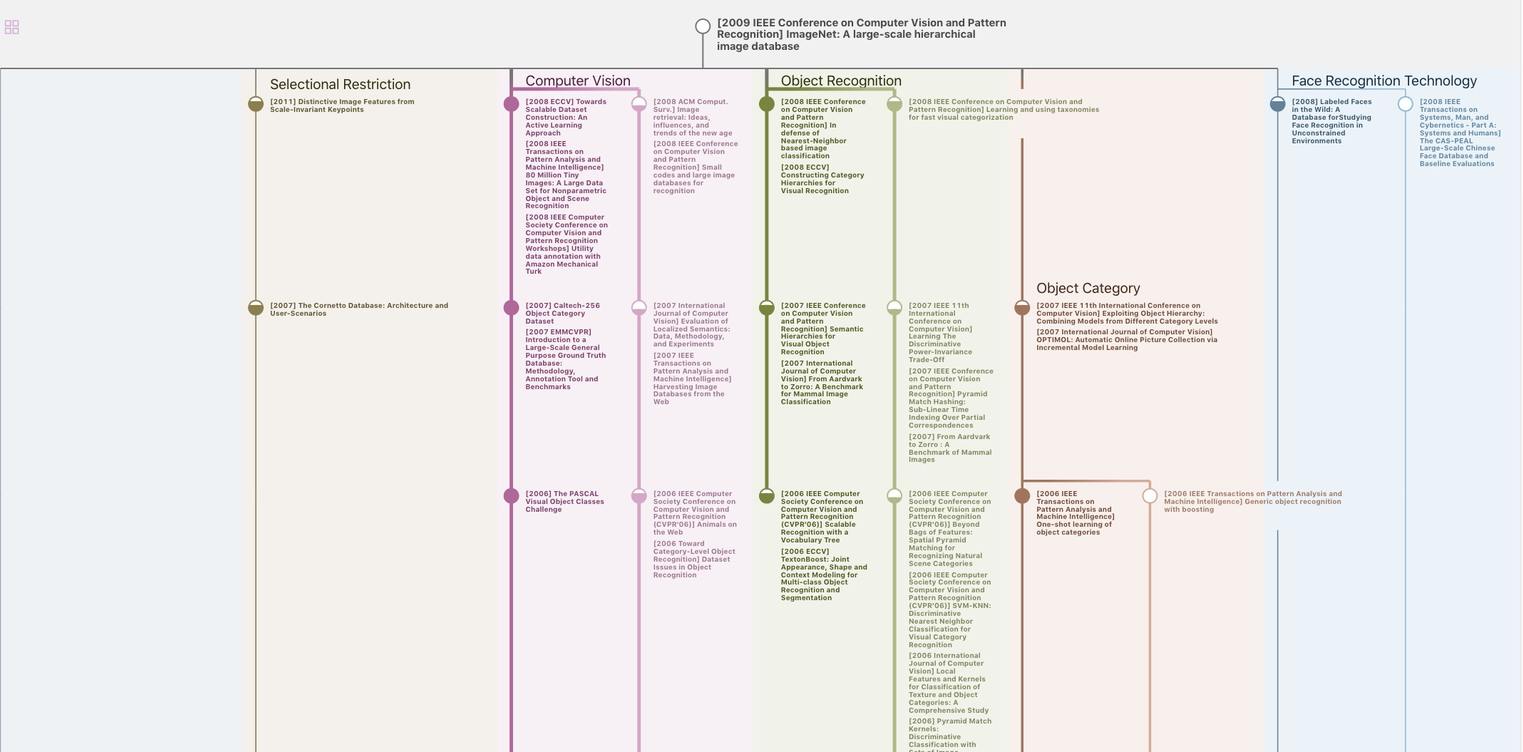
生成溯源树,研究论文发展脉络
Chat Paper
正在生成论文摘要