Probabilistic Structure From Motion With Objects (Psfmo)
2017 IEEE INTERNATIONAL CONFERENCE ON COMPUTER VISION (ICCV)(2017)
摘要
This paper proposes a probabilistic approach to recover affine camera calibration and objects position/occupancy from multi-view images using solely the information from image detections. We show that remarkable object localisation and volumetric occupancy can be recovered by including both geometrical constraints and prior information given by objects CAD models from the ShapeNet dataset. This can be done by recasting the problem in the context of a probabilistic framework based on PPCA that enforces both geometrical constraints and the associated semantic given by the object category extracted by the object detector. We present results on synthetic data and extensive real evaluation on the ScanNet datasets on more than 1200 image sequences to show the validity of our approach in realistic scenarios. In particular, we show that 3D statistical priors are key to obtain reliable reconstruction especially when the input detections are noisy, a likely case in real scenes.
更多查看译文
关键词
PSfMO,probabilistic approach,affine camera calibration,multiview images,image detections,volumetric occupancy,geometrical constraints,prior information,objects CAD models,probabilistic framework,object category,object detector,ScanNet datasets,3D statistical priors,input detections,object localisation,image sequences,probabilistic structure-from-motion-with-objects,objects position,objects occupancy,PPCA,probabilistic principal components analysis
AI 理解论文
溯源树
样例
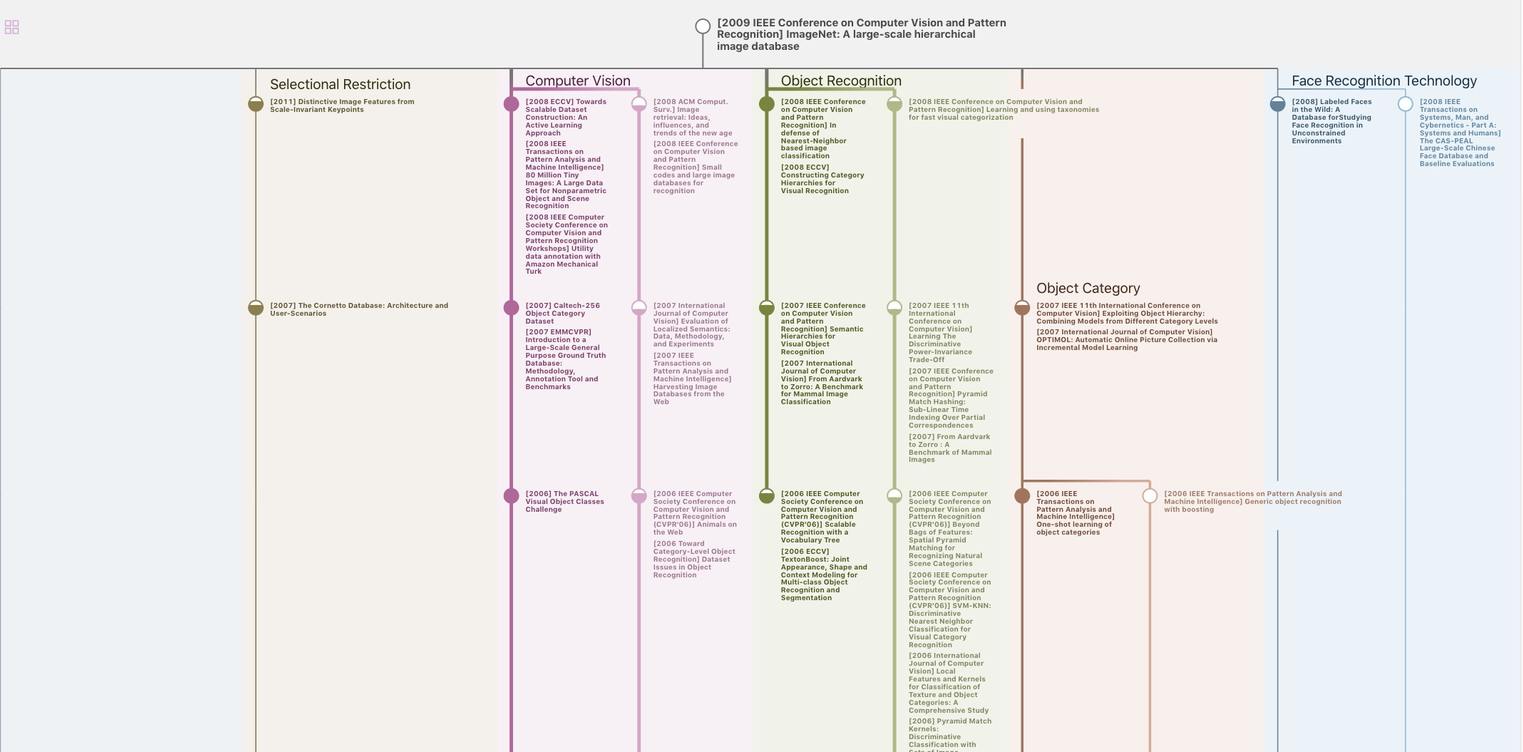
生成溯源树,研究论文发展脉络
Chat Paper
正在生成论文摘要