Punda: Probabilistic Unsupervised Domain Adaptation For Knowledge Transfer Across Visual Categories
2017 IEEE INTERNATIONAL CONFERENCE ON COMPUTER VISION (ICCV)(2017)
摘要
This paper introduces a probabilistic latent variable model to address unsupervised domain adaptation problems. Specifically, we tackle the task of categorization of visual input from different domains by learning projections from each domain to a latent (shared) space jointly with the classifier in the latent space, which simultaneously minimizes the domain disparity while maximizing the classifier's discriminative power. Furthermore, the non-parametric nature of our adaptation model makes it possible to infer the latent space dimension automatically from data. We also develop a novel regularized Variational Bayes (VB) algorithm for efficient estimation of the model parameters. We compare the proposed model with the state-of-the-art methods for the tasks of visual domain adaptation using both handcrafted and deep-net features. Our experiments show that even with a simple softmax classifier, our model outperforms several state-of-the-art methods that take advantage of more sophisticated classification schemes.
更多查看译文
关键词
probabilistic unsupervised domain adaptation,knowledge transfer,visual categories,probabilistic latent variable model,unsupervised domain adaptation problems,domain disparity,nonparametric nature,latent space dimension,state-of-the-art methods,visual domain adaptation,simple softmax classifier,PUnDA,visual input categorization task,classifier discriminative power,variational Bayes algorithm,handcrafted deep-net features
AI 理解论文
溯源树
样例
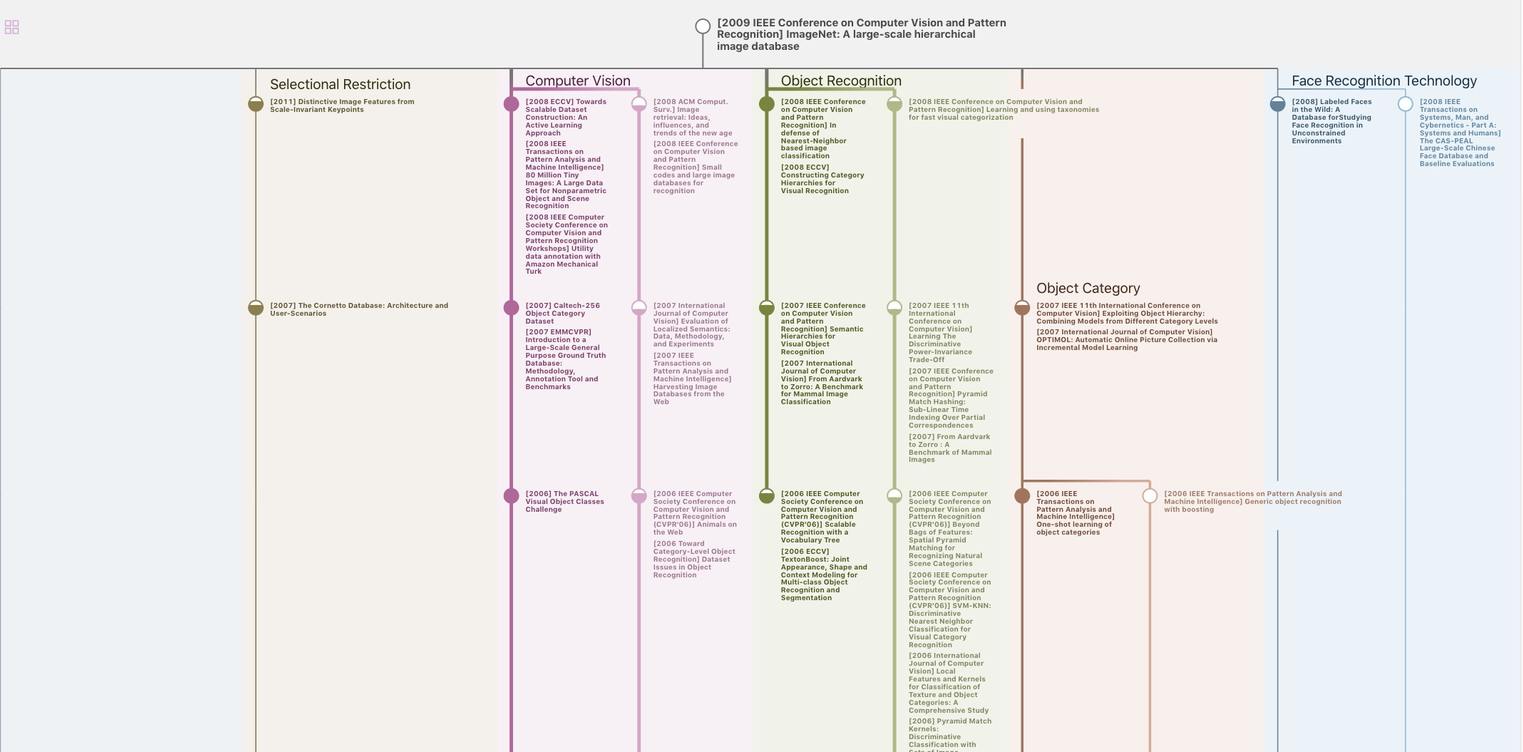
生成溯源树,研究论文发展脉络
Chat Paper
正在生成论文摘要