Image-Based Localization Using LSTMs for Structured Feature Correlation
2017 IEEE International Conference on Computer Vision (ICCV)(2017)
Abstract
In this work we propose a new CNN+LSTM architecture for camera pose regression for indoor and outdoor scenes. CNNs allow us to learn suitable feature representations for localization that are robust against motion blur and illumination changes. We make use of LSTM units on the CNN output, which play the role of a structured dimensionality reduction on the feature vector, leading to drastic improvements in localization performance. We provide extensive quantitative comparison of CNN-based and SIFT-based localization methods, showing the weaknesses and strengths of each. Furthermore, we present a new large-scale indoor dataset with accurate ground truth from a laser scanner. Experimental results on both indoor and outdoor public datasets show our method outperforms existing deep architectures, and can localize images in hard conditions, e.g., in the presence of mostly textureless surfaces, where classic SIFT-based methods fail.
MoreTranslated text
Key words
outdoor scenes,LSTM units,CNN output,structured dimensionality reduction,feature vector,localization performance,large-scale indoor dataset,laser scanner,indoor public datasets,outdoor public datasets,feature representations,image-based localization,camera pose regression,CNN-based localization method,SIFT-based localization method,indoor scenes,CNN+LSTM architecture,structured feature correlation
AI Read Science
Must-Reading Tree
Example
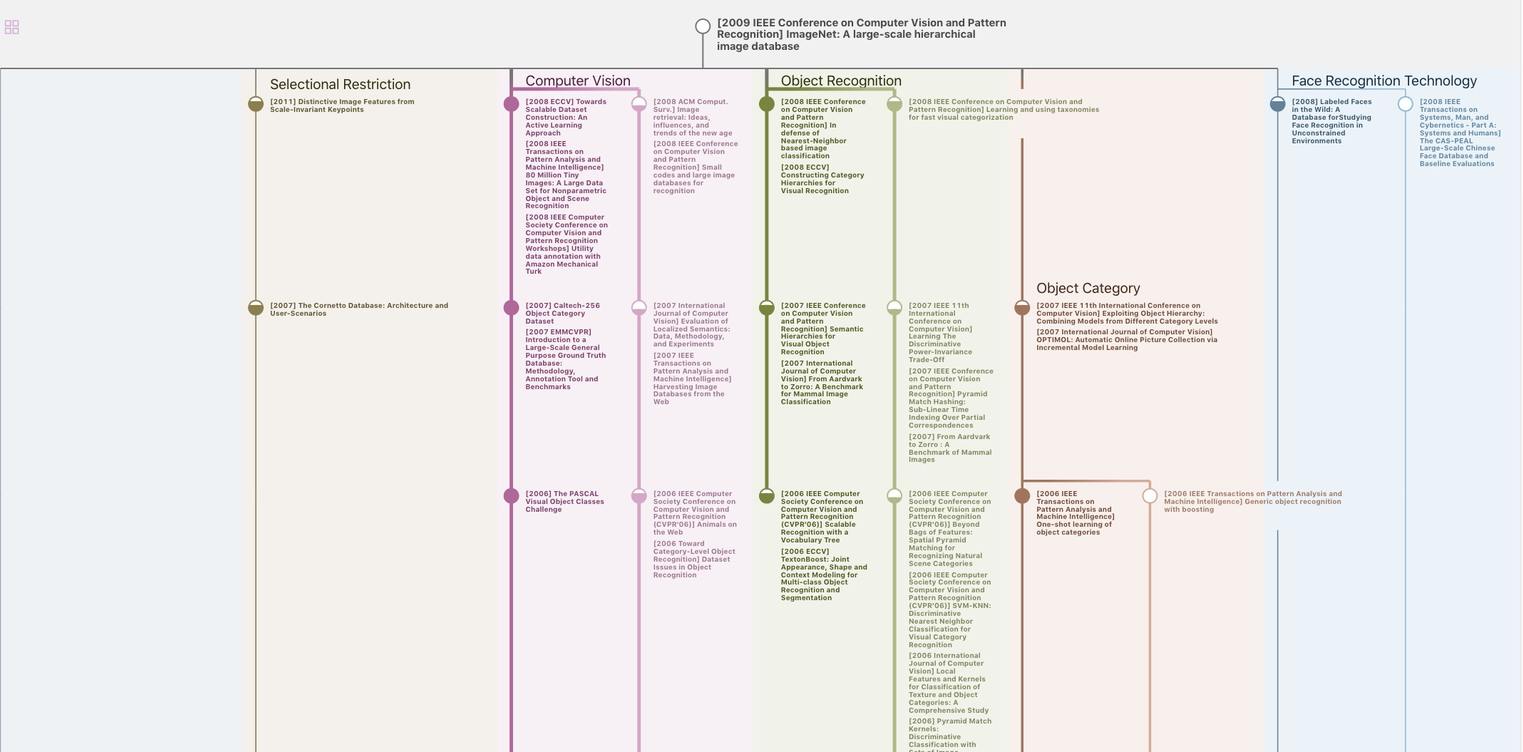
Generate MRT to find the research sequence of this paper
Chat Paper
Summary is being generated by the instructions you defined