Low-Shot Visual Recognition By Shrinking And Hallucinating Features
2017 IEEE INTERNATIONAL CONFERENCE ON COMPUTER VISION (ICCV)(2017)
摘要
Low-shot visual learning-the ability to recognize novel object categories from very few examples-is a hallmark of human visual intelligence. Existing machine learning approaches fail to generalize in the same way. To make progress on this foundational problem, we present a lowshot learning benchmark on complex images that mimics challenges faced by recognition systems in the wild. We then propose (1) representation regularization techniques, and (2) techniques to hallucinate additional training examples for data-starved classes. Together, our methods improve the effectiveness of convolutional networks in low-shot learning, improving the one-shot accuracy on novel classes by 2.3x on the challenging ImageNet dataset.
更多查看译文
关键词
low-shot visual recognition,object categories,human visual intelligence,recognition systems,one-shot accuracy,machine learning,low-shot visual learning,representation regularization techniques,feature hallucination,feature shrinking,low-shot learning
AI 理解论文
溯源树
样例
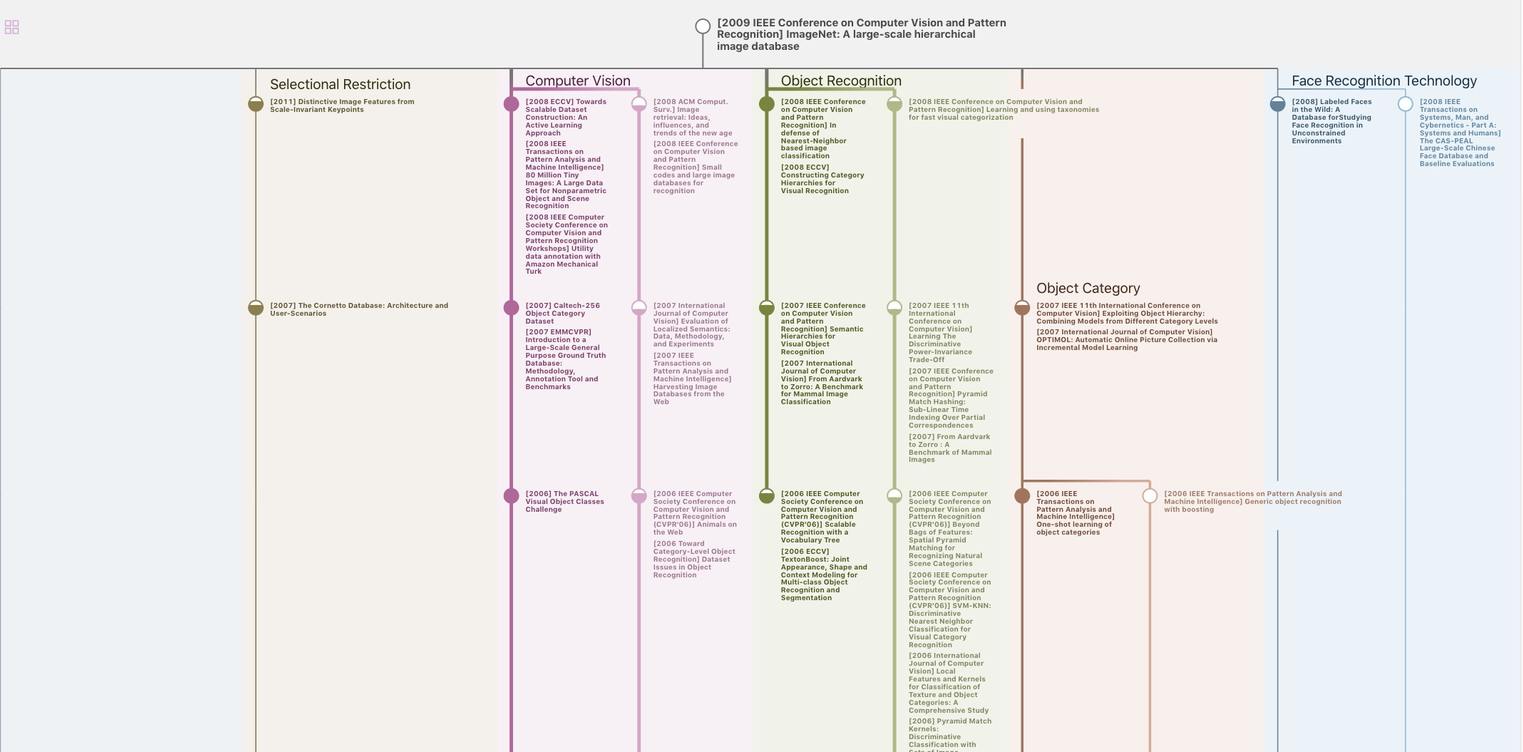
生成溯源树,研究论文发展脉络
Chat Paper
正在生成论文摘要