Improving Child Speech Disorder Assessment By Incorporating Out-Of-Domain Adult Speech
18TH ANNUAL CONFERENCE OF THE INTERNATIONAL SPEECH COMMUNICATION ASSOCIATION (INTERSPEECH 2017), VOLS 1-6: SITUATED INTERACTION(2017)
摘要
This paper describes the continued development of a system to provide early assessment of speech development issues in children and better triaging to professional services. Whilst corpora of children's speech are increasingly available, recognition of disordered children's speech is still a data-scarce task. Transfer learning methods have been shown to be effective at leveraging out-of-domain data to improve ASR performance in similar data-scarce applications. This paper combines transfer learning, with previously developed methods for constrained decoding based on expert speech pathology knowledge and knowledge of the target text. Results of this study show that transfer learning with out-of-domain adult speech can improve phoneme recognition for disordered children's speech. Specifically. a Deep Neural Network (DNN) trained on adult speech and finetuned on a corpus of disordered children's speech reduced the phoneme error rate (PER) of a DNN trained on a children's corpus from 16.3% to 14.2%. Furthermore, this fine-tuned DNN also improved the performance of a Hierarchal Neural Network based acoustic model previously used by the system with a PER of 19.3%. We close with a discussion of our planned future developments of the system.
更多查看译文
关键词
Automated Speech Recognition, Speech Therapy, Speech Assessment Tools
AI 理解论文
溯源树
样例
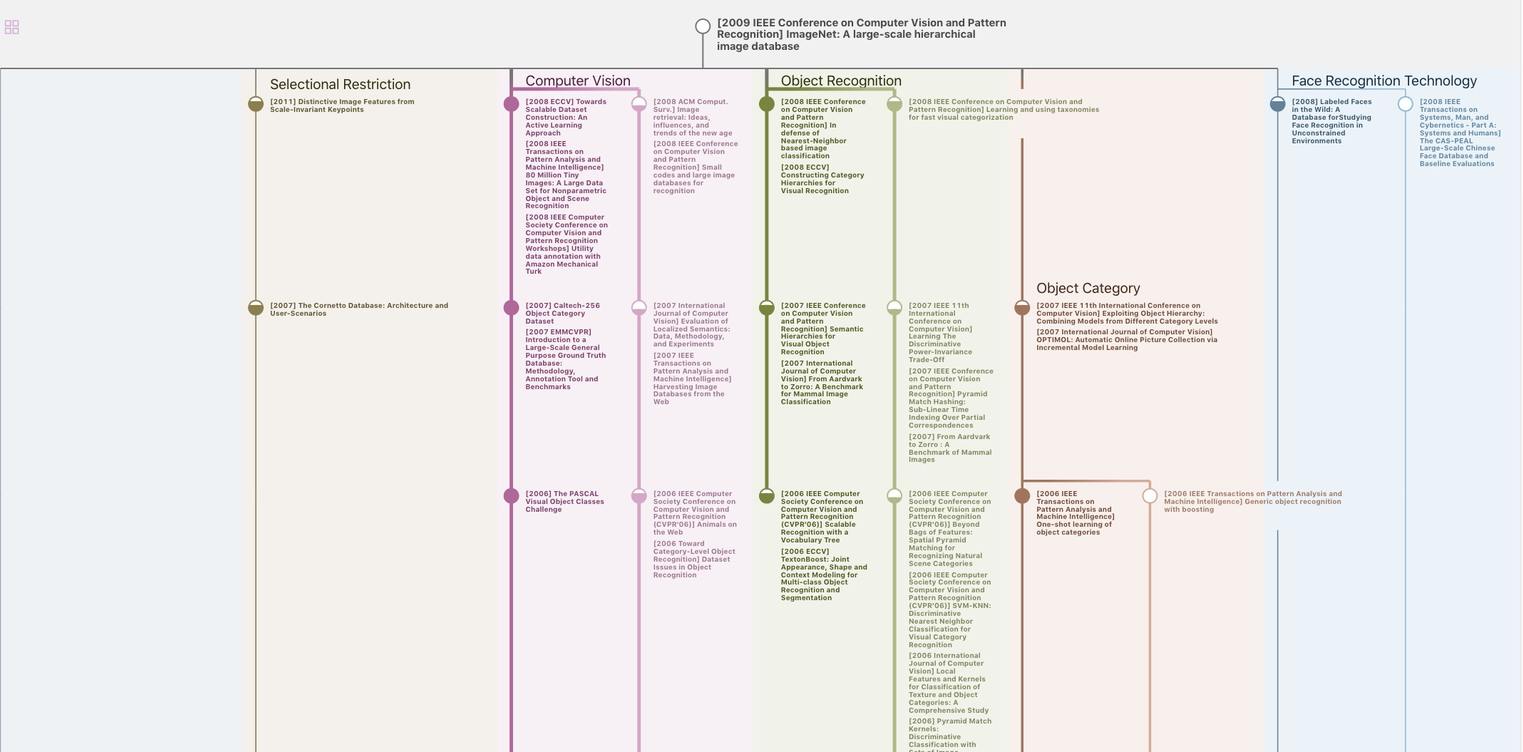
生成溯源树,研究论文发展脉络
Chat Paper
正在生成论文摘要