Color Representation In Cnns: Parallelisms With Biological Vision
2017 IEEE INTERNATIONAL CONFERENCE ON COMPUTER VISION WORKSHOPS (ICCVW 2017)(2017)
摘要
Convolutional Neural Networks (CNNs) trained for object recognition tasks present representational capabilities approaching to primate visual systems [1]. This provides a computational framework to explore how image features are efficiently represented. Here, we dissect a trained CNN [2] to study how color is represented. We use a classical methodology used in physiology that is measuring index of selectivity of individual neurons to specific features. We use ImageNet Dataset [20] images and synthetic versions of them to quantify color tuning properties of artificial neurons to provide a classification of the network population. We conclude three main levels of color representation showing some parallelisms with biological visual systems: (a) a decomposition in a circular hue space to represent single color regions with a wider hue sampling beyond the first layer (V2), (b) the emergence of opponent low-dimensional spaces in early stages to represent color edges (V1); and (c) a strong entanglement between color and shape patterns representing object-parts (e.g. wheel of a car), object-shapes (e.g. faces) or object-surrounds configurations (e.g. blue sky surrounding an object) in deeper layers (V4 or IT).
更多查看译文
关键词
biological vision,image features,artificial neurons,biological visual systems,circular hue space,single color regions,wider hue sampling,opponent low-dimensional spaces,object-surrounds configurations,CNN,convolutional neural networks,object recognition tasks,primate visual systems,ImageNet dataset images,color tuning properties,network population classification,object-parts representation,color edge representation
AI 理解论文
溯源树
样例
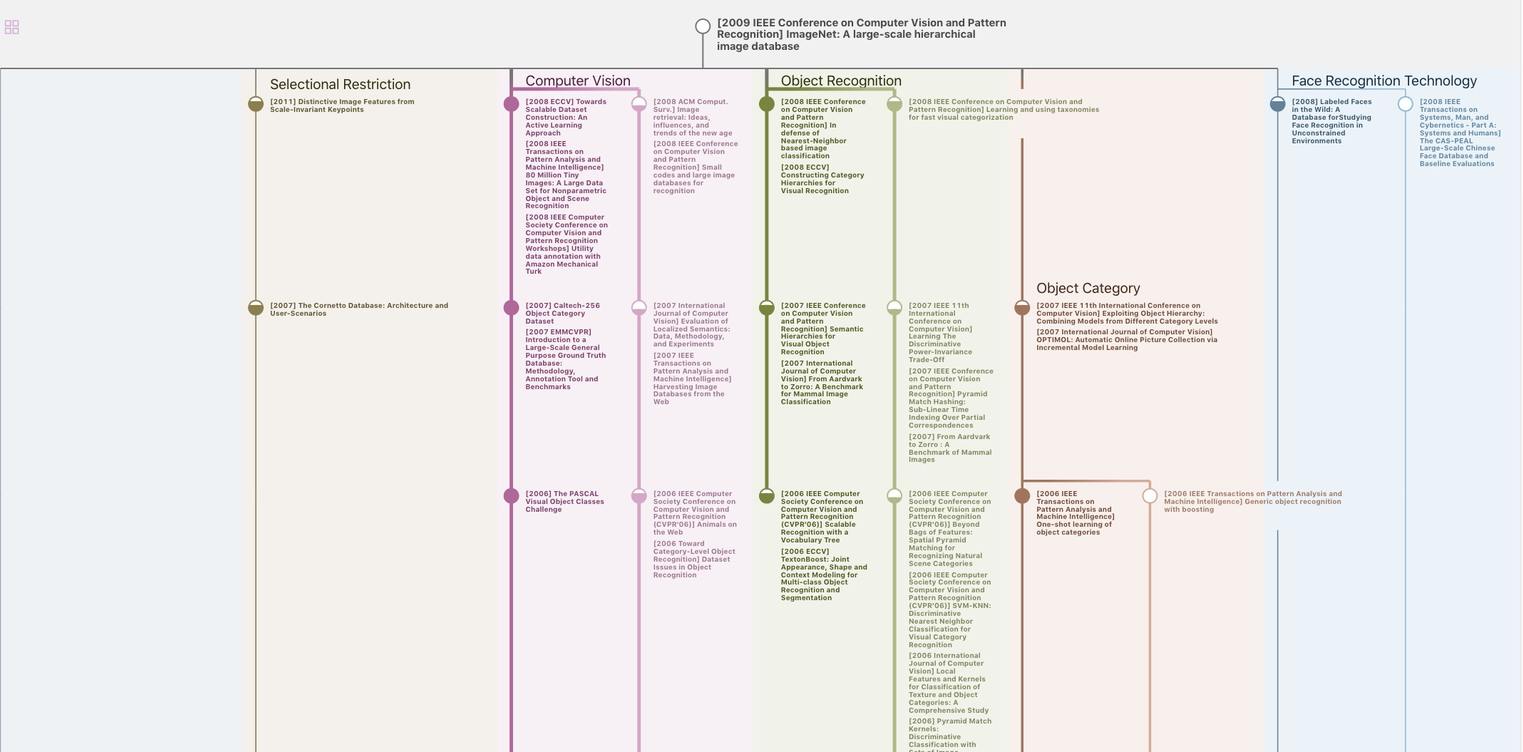
生成溯源树,研究论文发展脉络
Chat Paper
正在生成论文摘要