Fast Vehicle Detector for Autonomous Driving
2017 IEEE International Conference on Computer Vision Workshops (ICCVW)(2017)
Abstract
This paper presents a fast vehicle detector which can be deployed on NVIDIA DrivePX2 under real-time constraints. The network predicts bounding boxes with different aspect ratio and scale priors from the specifically-designed prediction module given concatenated multi-scale feature map. A new data augmentation strategy is proposed to systematically generate a lot of vehicle training images whose appearance is randomly truncated so our detector could detect occluded vehicles better. Besides, we propose a non-region-based online hard example mining framework which performs fine-tuning by picking (1) hard examples and (2) detection results with insufficient IOU. Compared to other classical object detectors, this work achieves very competitive result in terms of average precision (AP) and computational speed. For the newly-defined vehicle class (car+bus) on VOC2007 test, our detector achieves 85.32 AP and runs at 48 FPS and 30 FPS on NVIDIA Titan X & GP106 (DrivePX2), respectively.
MoreTranslated text
Key words
classical object detectors,vehicle class,fast vehicle detector,autonomous driving,NVIDIA DrivePX2,real-time constraints,scale priors,prediction module,concatenated multiscale feature map,data augmentation strategy,bounding boxes prediction,aspect ratio,vehicle training images,nonregion-based online hard example mining framework,VOC2007 test,NVIDIA Titan X,NVIDIA Titan GP106,FPS,VOC2007
AI Read Science
Must-Reading Tree
Example
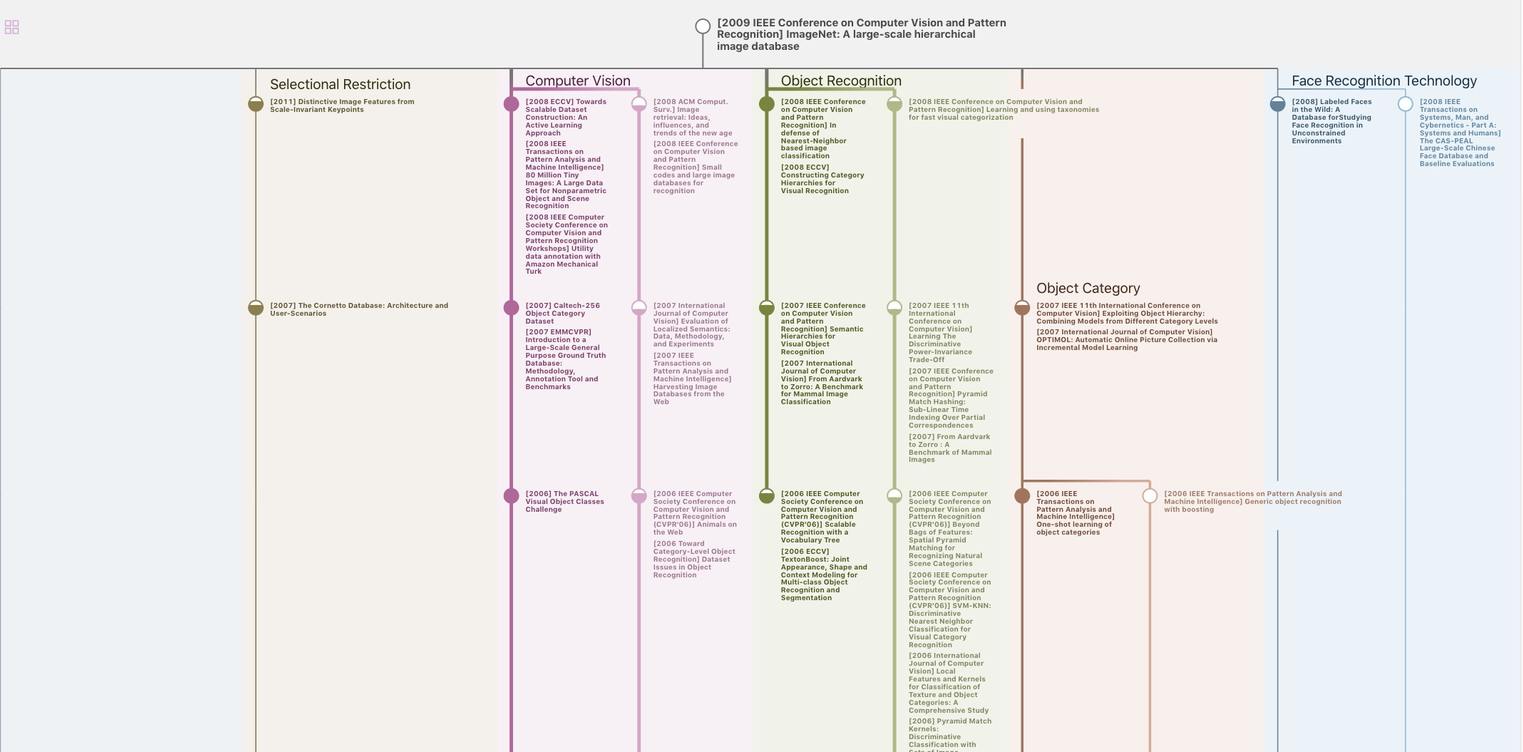
Generate MRT to find the research sequence of this paper
Chat Paper
Summary is being generated by the instructions you defined