Including Multi-feature Interactions and Redundancy for Feature Ranking in Mixed Datasets.
Lecture Notes in Artificial Intelligence(2017)
摘要
Feature ranking is beneficial to gain knowledge and to identify the relevant features from a high-dimensional dataset. However, in several datasets, few features by itself might have small correlation with the target classes, but by combining these features with some other features, they can be strongly correlated with the target. This means that multiple features exhibit interactions among themselves. It is necessary to rank the features based on these interactions for better analysis and classifier performance. However, evaluating these interactions on large datasets is computationally challenging. Furthermore, datasets often have features with redundant information. Using such redundant features hinders both efficiency and generalization capability of the classifier. The major challenge is to efficiently rank the features based on relevance and redundance on mixed datasets. In this work, we propose a filter-based framework based on Relevance and Redundancy (RaR), RaR computes a single score that quantifies the feature relevance by considering interactions between features and redundancy. The top ranked features of RaR are characterized by maximum relevance and non-redundance. The evaluation on synthetic and real world datasets demonstrates that our approach outperforms several state-of-the-art feature selection techniques. Code and data related to this chapter are available at: https://doi.org/10.6084/m9.figshare.5418706.
更多查看译文
AI 理解论文
溯源树
样例
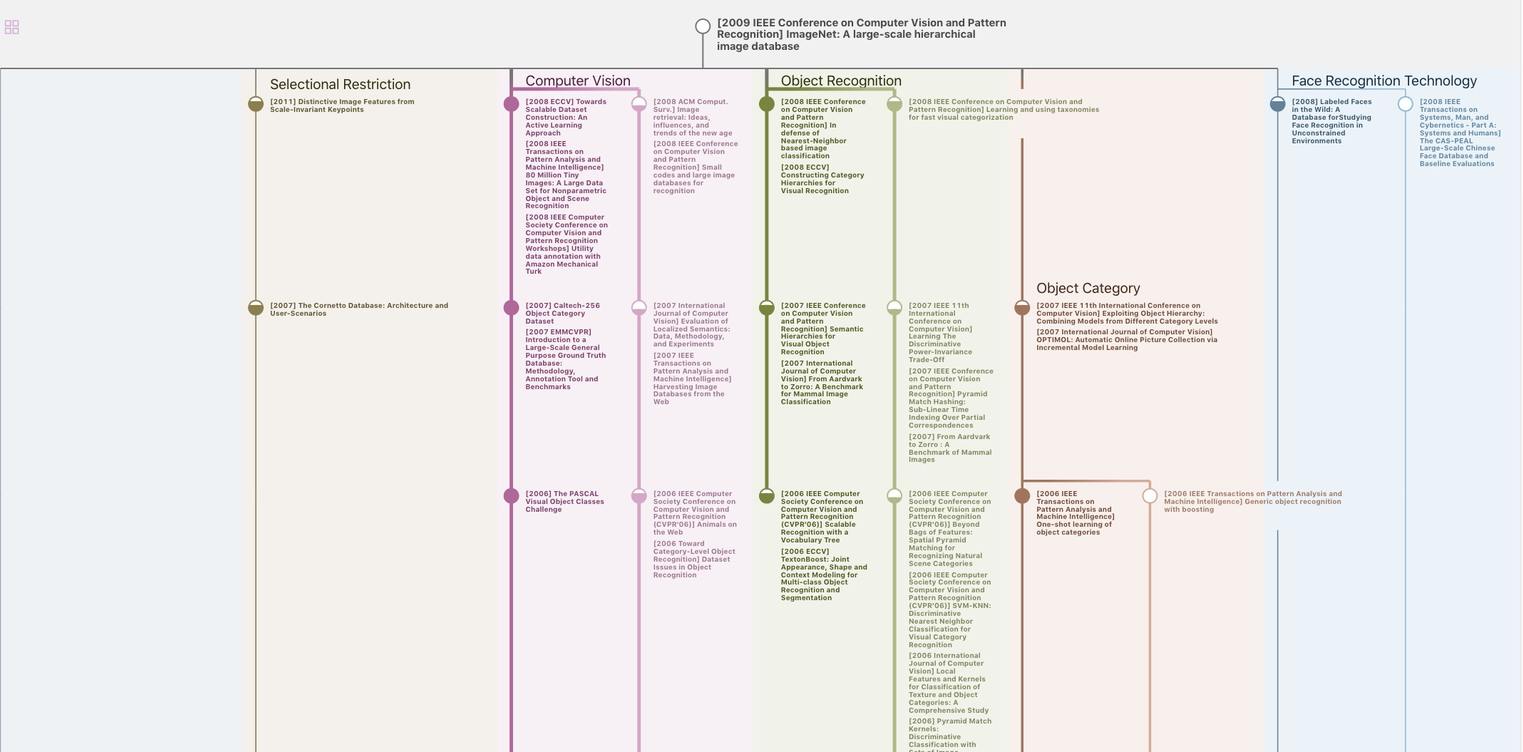
生成溯源树,研究论文发展脉络
Chat Paper
正在生成论文摘要