A Scalable Multi-Granular Data Model For Data Parallel Workflows
PROCEEDINGS OF THE INTERNATIONAL CONFERENCE ON HIGH PERFORMANCE COMPUTING IN ASIA-PACIFIC REGION (HPC ASIA 2018)(2018)
摘要
Scientific applications consist of many tasks and each task has different requirements for the degree of parallelism and data access pattern. To satisfy these requirements, a task scheduling has to assign required number of processes to each task and task's input has to be decomposed and arranged to these processes by considering data access pattern to exploit data locality. However, hand-writing these code is a troublesome and error-prone work. We propose a multi-view data model where users can specify rules of data decomposition for multi-dimensional data to change data layout on top of processes and define unit of parallel processing by simple directives. Our framework conducts data arrangement and affinity-aware task scheduling transparently from users by following the specified rules. Through a case study of a lattice QCD simulation program, we confirmed that our proposal reduced programming efforts against hand-written MPI code with performance penalties up to 17%.
更多查看译文
AI 理解论文
溯源树
样例
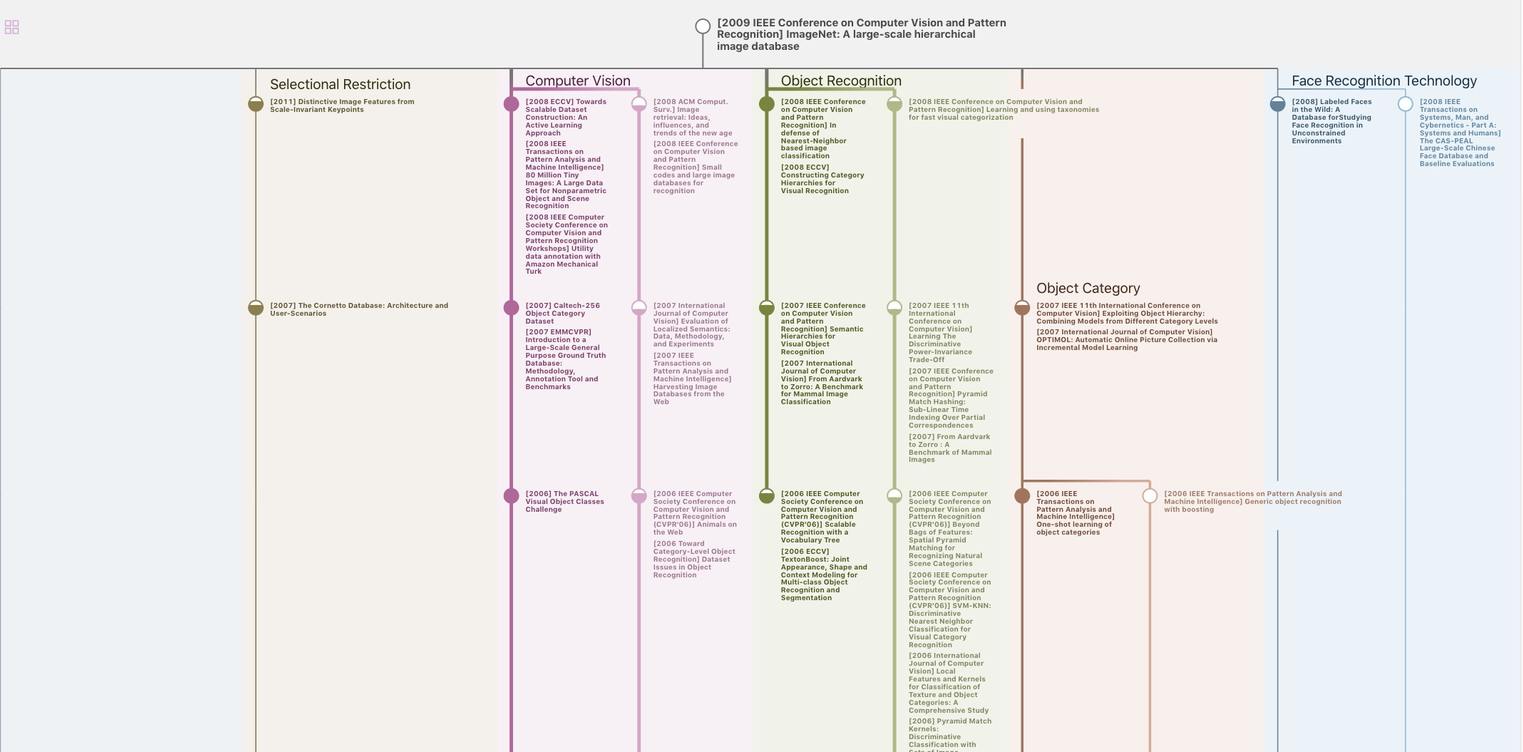
生成溯源树,研究论文发展脉络
Chat Paper
正在生成论文摘要