Acute Coronary Syndrome Risk Prediction Based on GRACE Risk Score.
Studies in Health Technology and Informatics(2017)
Abstract
Clinical risk prediction of acute coronary syndrome (ACS) plays a critical role for clinical decision support, treatment management and quality of care assessment in ACS patients. Admission records contain a wealth of patient information in the early stages of hospitalization, which offers the opportunity to support the ACS risk prediction in a proactive manner. However, ACS patient risks aren't recorded in hospital admission records, thus impeding the construction of supervised risk prediction models. In our study, we propose a novel approach for ACS risk prediction, which employs a wellknown ACS risk prediction model (GRACE) as the benchmark methods to stratify patient risks, and then utilizes a state-of-theart supervised machine learning algorithm to establish our risk prediction models. The experiment was conducted with a collection of 3,643 ACS patient samples from a Chinese hospital. Our best model achieved 0.616 accuracy for risk prediction, which indicates our learned model can achieve a better performance than the benchmark GRACE model and can obtain significant improvement by mixing up patient samples that were manually labeled risks.
MoreTranslated text
Key words
Risk Assessment,Acute Coronary Syndrome,Supervised Machine Learning
AI Read Science
Must-Reading Tree
Example
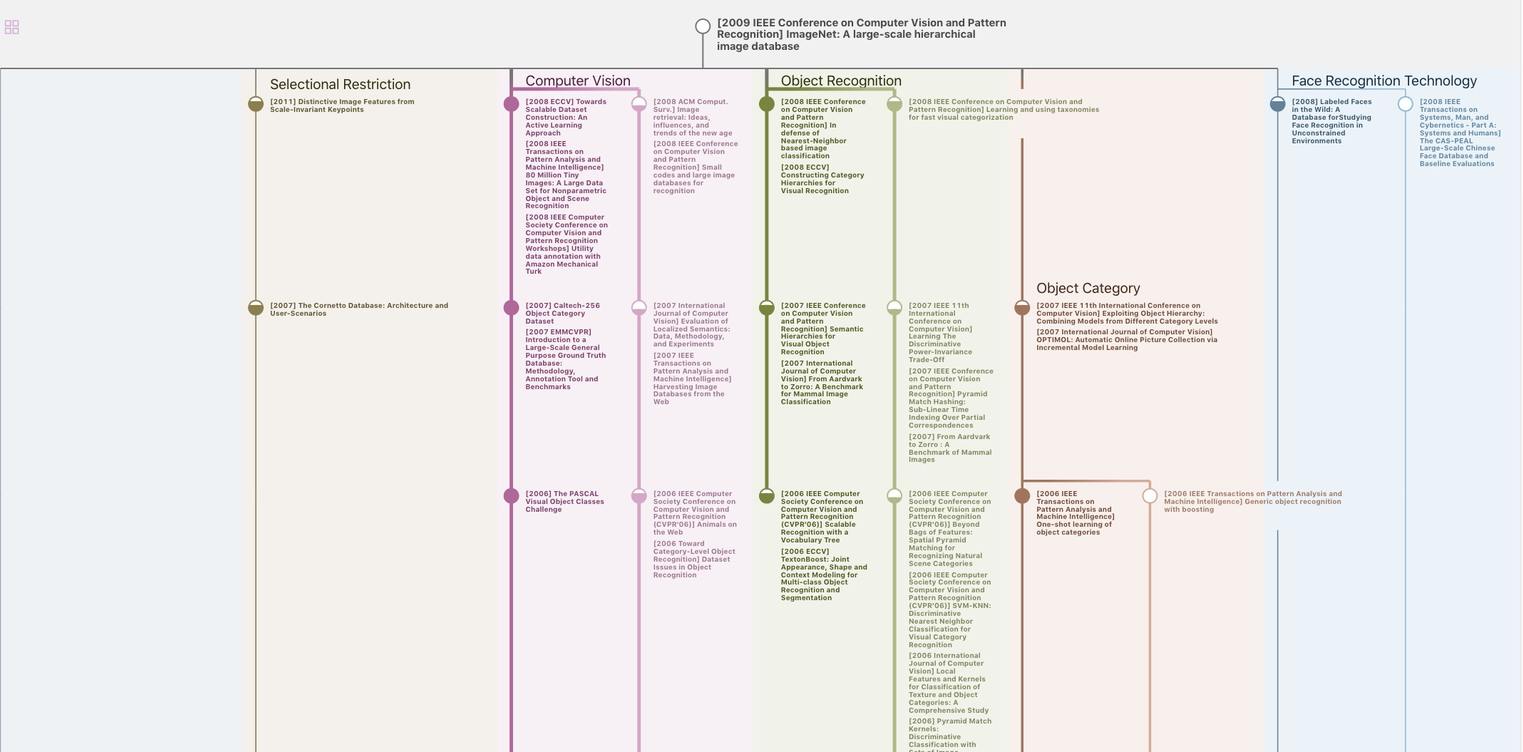
Generate MRT to find the research sequence of this paper
Chat Paper
Summary is being generated by the instructions you defined