Learning Word Representations for Sentiment Analysis
Cognitive Computation(2017)
摘要
Word embedding has been proven to be a useful model for various natural language processing tasks. Traditional word embedding methods merely take into account word distributions independently from any specific tasks. Hence, the resulting representations could be sub-optimal for a given task. In the context of sentiment analysis, there are various types of prior knowledge available, e.g., sentiment labels of documents from available datasets or polarity values of words from sentiment lexicons. We incorporate such prior sentiment information at both word level and document level in order to investigate the influence each word has on the sentiment label of both target word and context words. By evaluating the performance of sentiment analysis in each category, we find the best way of incorporating prior sentiment information. Experimental results on real-world datasets demonstrate that the word representations learnt by DLJT2 can significantly improve the sentiment analysis performance. We prove that incorporating prior sentiment knowledge into the embedding process has the potential to learn better representations for sentiment analysis.
更多查看译文
关键词
Sentiment Analysis,Word Embedding Algorithm,Sentiment Information,Prior Sentiment,Sentiment Lexicon
AI 理解论文
溯源树
样例
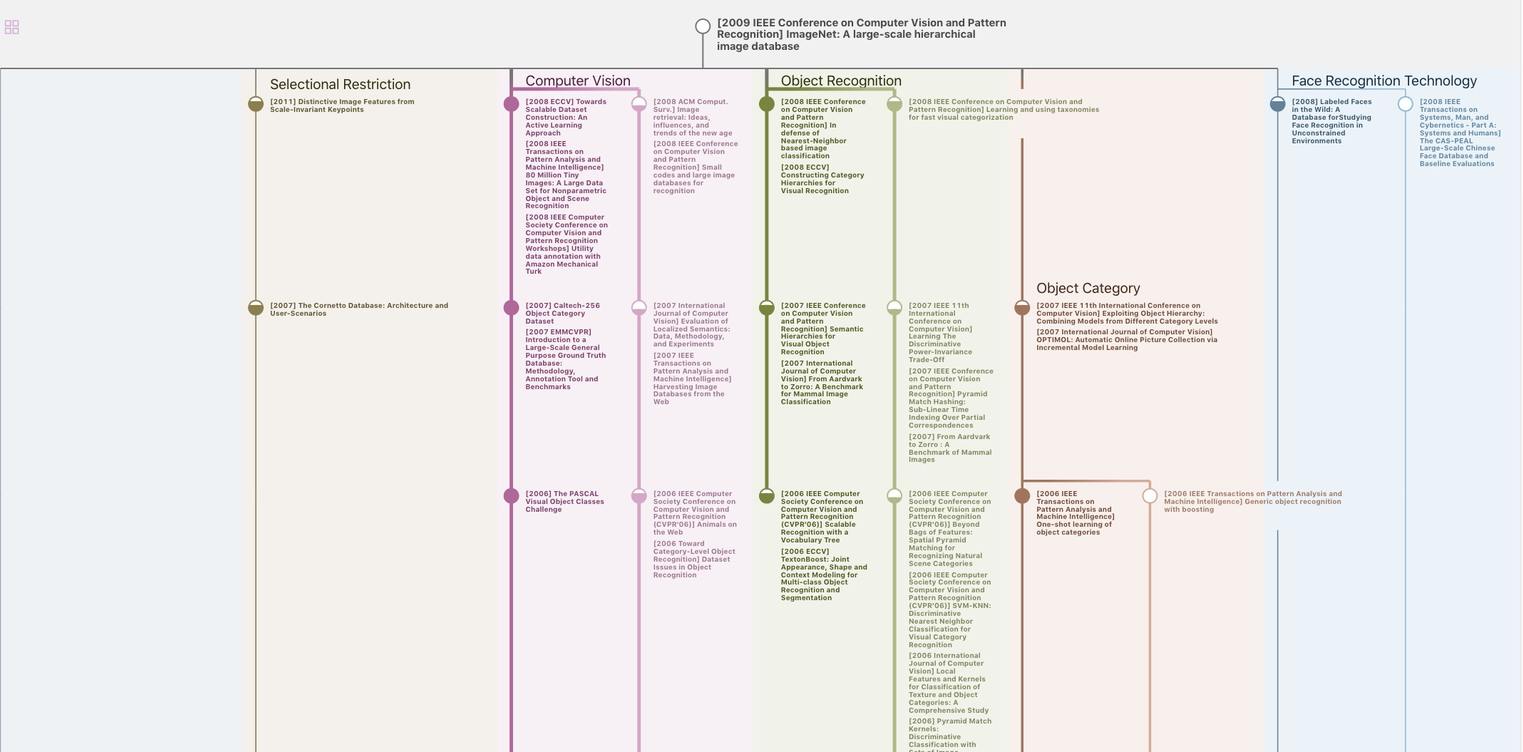
生成溯源树,研究论文发展脉络
Chat Paper
正在生成论文摘要