Language-Based Image Editing with Recurrent Attentive Models
2018 IEEE/CVF Conference on Computer Vision and Pattern Recognition(2018)
摘要
We investigate the problem of Language-Based Image Editing (LBIE). Given a source image and a natural language description, we want to generate a target image by editing the source image based on the description. We propose a generic modeling framework for two sub-tasks of LBIE: language-based image segmentation and image colorization. The framework uses recurrent attentive models to fuse image and language features. Instead of using a fixed step size, we introduce for each region of the image a termination gate to dynamically determine after each inference step whether to continue extrapolating additional information from the textual description. The effectiveness of the framework is validated on three datasets. First, we introduce a synthetic dataset, called CoSaL, to evaluate the end-to-end performance of our LBIE system. Second, we show that the framework leads to state-of-the-art performance on image segmentation on the ReferIt dataset. Third, we present the first language-based colorization result on the Oxford-102 Flowers dataset.
更多查看译文
关键词
image colorization,recurrent attentive models,language features,language-based colorization result,source image,natural language description,target image,generic modeling framework,language-based image segmentation,language-based image editing,termination gate,CoSaL,LBIE system,ReferIt dataset,Oxford-102 Flowers dataset
AI 理解论文
溯源树
样例
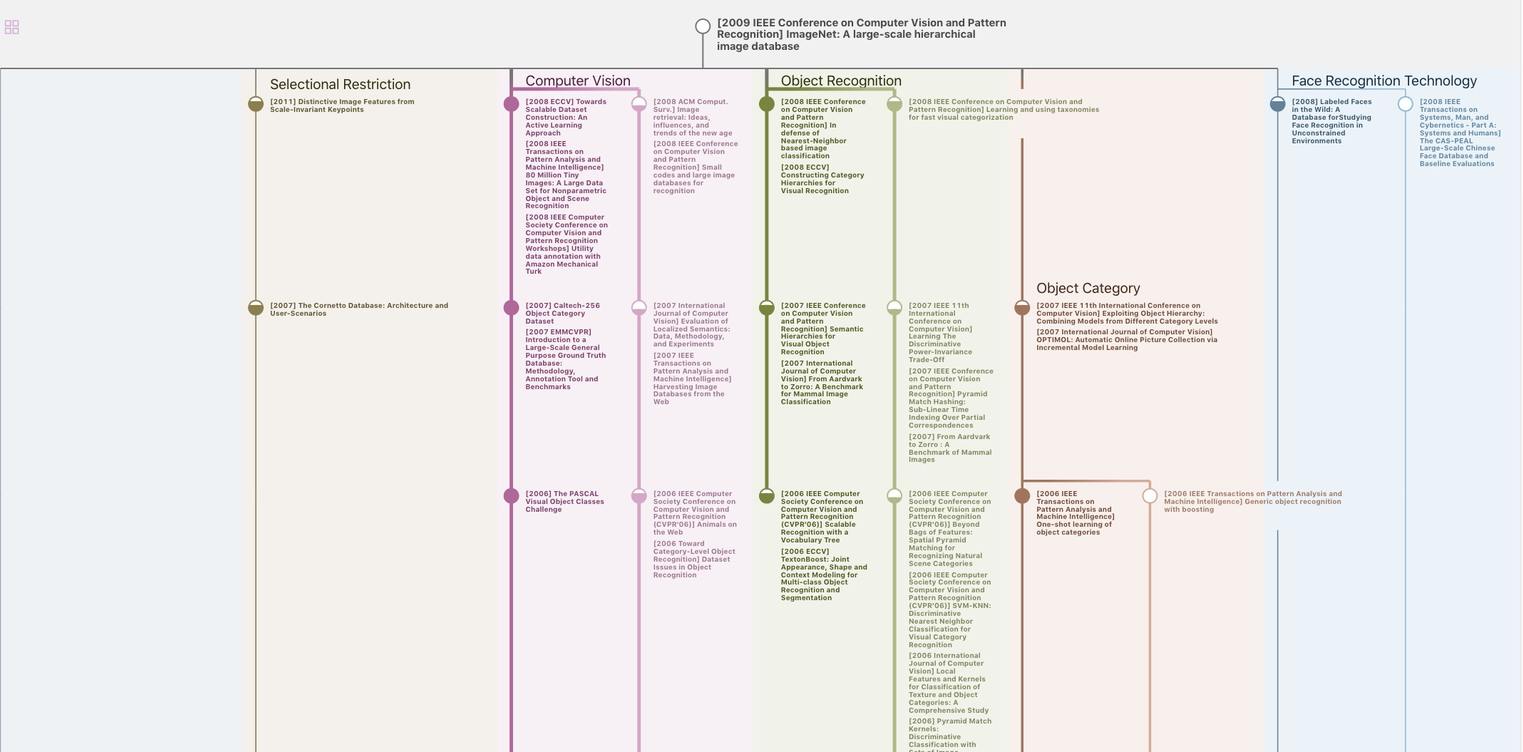
生成溯源树,研究论文发展脉络
Chat Paper
正在生成论文摘要