Hardening Quantum Machine Learning Against Adversaries.
NEW JOURNAL OF PHYSICS(2018)
摘要
Security of machine learning has begun to become a serious issue for present day applications. An important question remaining is whether emerging quantum technologies will help or hinder the security of machine learning. Here we discuss a number of ways that quantum information can be used to help make quantum classifiers more secure or private. In particular, we demonstrate a form of robust principal component analysis that, under some circumstances, can provide an exponential speedup relative to robust methods used at present. To demonstrate this approach we introduce a linear combinations of unitaries Hamiltonian simulation method that we show functions when given an imprecise Hamiltonian oracle, which maybe of independent interest. We also introduce a new quantum approach for bagging and boosting that can use quantum superposition over the classifiers or splits of the training set to aggragate over many more models than would be possible classically. Finally, we provide a private form of k-means clustering that can be used to prevent an all powerful adversary from learning more than a small fraction of a bit from any user. These examples show the role that quantum technologies can play in the security of ML and vice versa. This illustrates that quantum computing can provide useful advantages to machine learning apart from speedups.
更多查看译文
关键词
quantum computing,quantum machine learning,quantum algorithms
AI 理解论文
溯源树
样例
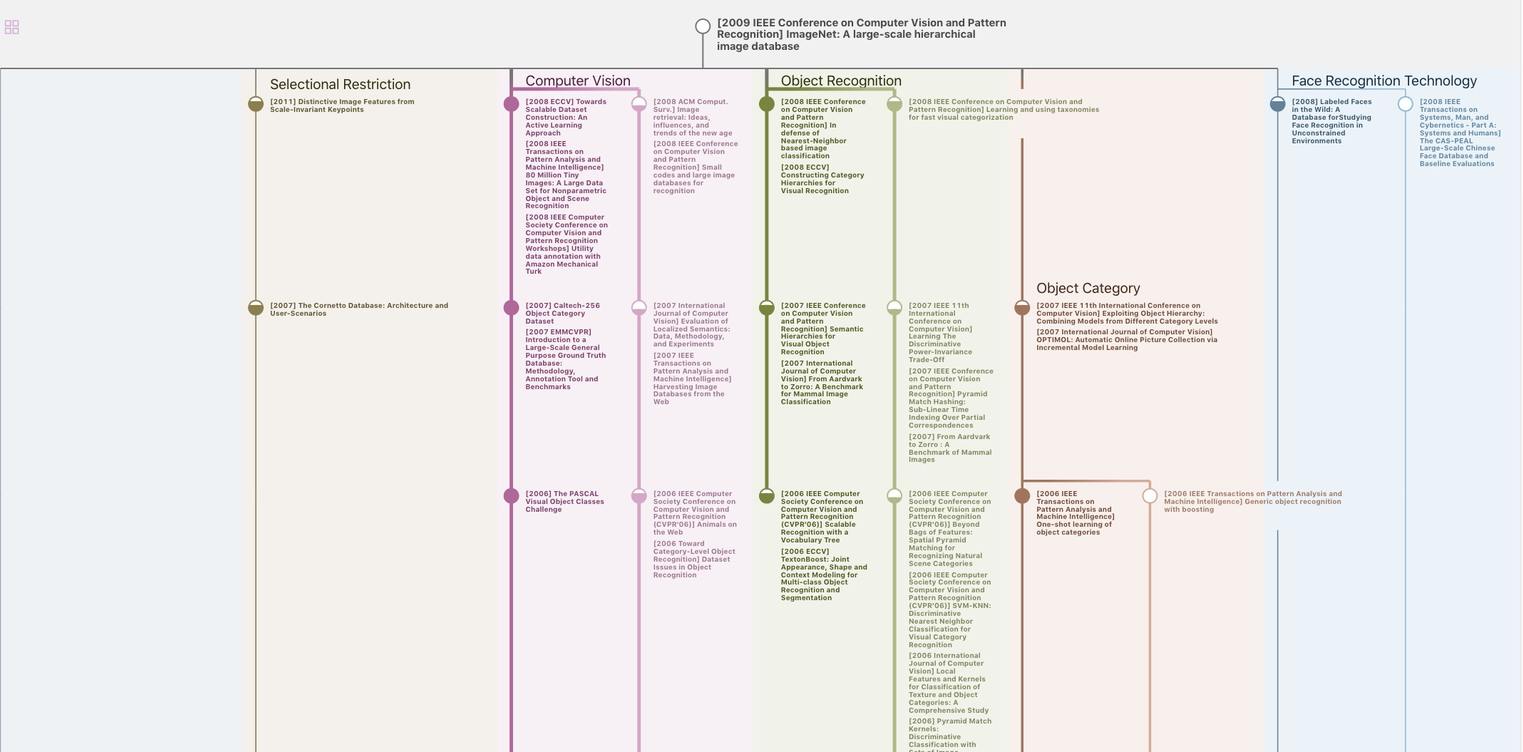
生成溯源树,研究论文发展脉络
Chat Paper
正在生成论文摘要