Classification Of Motor Imagery Based Eeg Signals Using Sparsity Approach
INTELLIGENT HUMAN COMPUTER INTERACTION, IHCI 2017(2017)
摘要
The advancement in brain-computer interface systems (BCIs) gives a new hope to people with special needs in restoring their independence. Since, BCIs using motor imagery (MI) rhythms provides high degree of freedom, it is been used for many real-time applications, especially for locked-in people. The available BCIs using MI-based EEG signals usually makes use of spatial filtering and powerful classification methods to attain better accuracy and performance. Inter-subject variability and speed of the classifier is still a issue in MI-based BCIs. To address the aforementioned issues, in this work, we propose a new classification method, spatial filtering based sparsity (SFS) approach for MI-based BCIs. The proposed method makes use of common spatial pattern (CSP) to spatially filter the MI signals. Then frequency bandpower and wavelet features from the spatially filtered signals are used to bulid two different over-complete dictionary matrix. This dictionary matrix helps to overcome the issue of inter-subject variability. Later, sparse representation based classification is carried out to classify the two-class MI signals. We analysed the performance of the proposed approach using publicly available MI dataset IVa from BCI competition III. The proposed SFS method provides better classification accuracy and runtime than the well-known support vector machine (SVM) and logistic regression (LR) classification methods. This SFS method can be further used to develop a real-time application for people with special needs.
更多查看译文
关键词
Electroencephalography (EEG),Brain computer interface (BCI),Motor imagery (MI),Sparisty based classification,BCI for motor impaired users
AI 理解论文
溯源树
样例
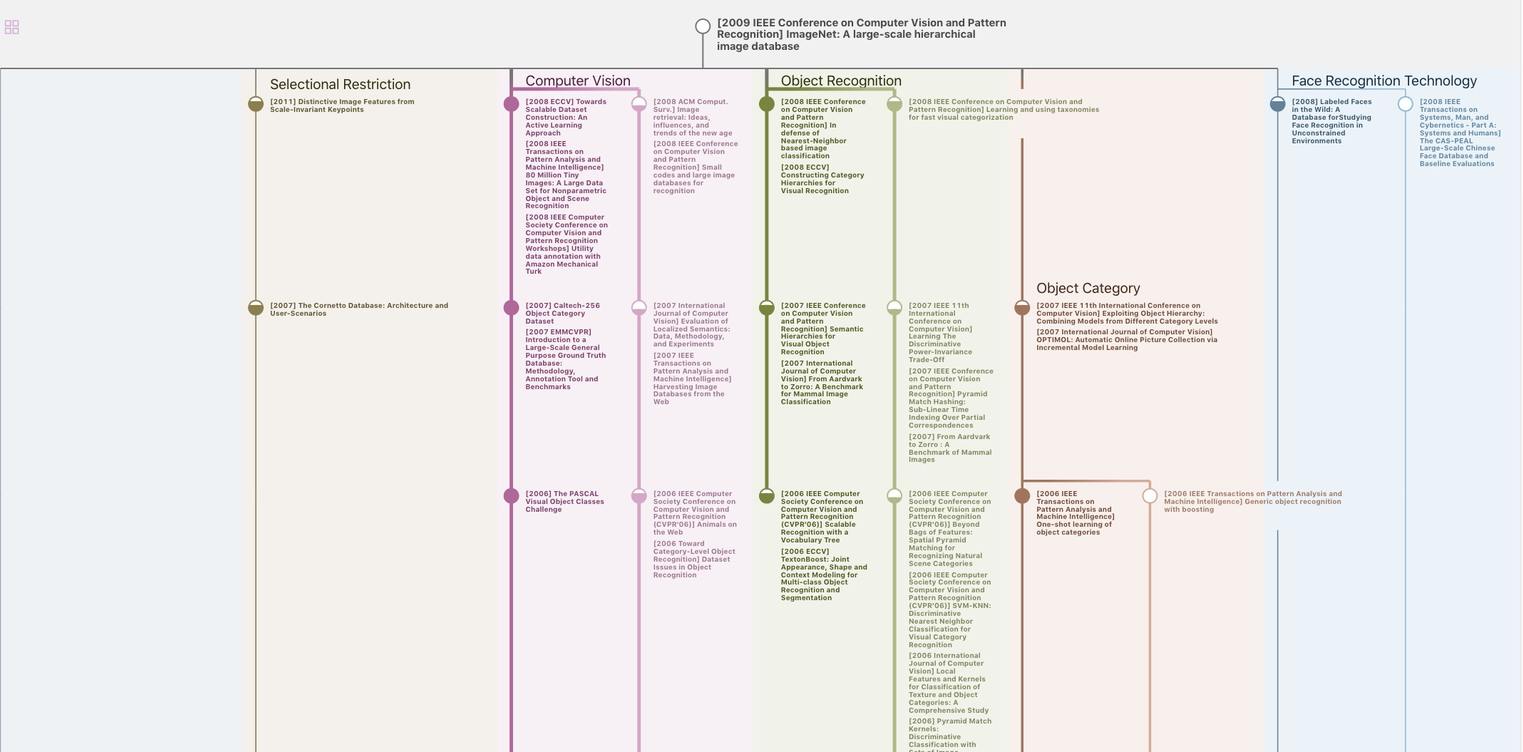
生成溯源树,研究论文发展脉络
Chat Paper
正在生成论文摘要