Bsmboost For Imbalanced Pattern Classification Problems
2017 IEEE INTERNATIONAL CONFERENCE ON SYSTEMS, MAN, AND CYBERNETICS (SMC)(2017)
摘要
Numbers of samples in different classes are in nature imbalanced in many machine learning problems. Single classifier-based methods are subject to high variance. Therefore, ensemble-based methods are more suitable for dealing with imbalanced pattern classification problems. In this work, we propose a boosting-based method: BSMBoost which creates an ensemble of classifiers using samples selected by both the stochastic sensitivity measure (SSM) and the AdaBoost algorithm to yield higher and more robust performances. Experimental results show that the BSMBoost yields better and more robust performances in comparison to other state-of-the-art boosting-based imbalanced classification methods.
更多查看译文
关键词
Imbalanced Classification Problem, Boosting, Stochastic Sensitivity Measure, Undersampling
AI 理解论文
溯源树
样例
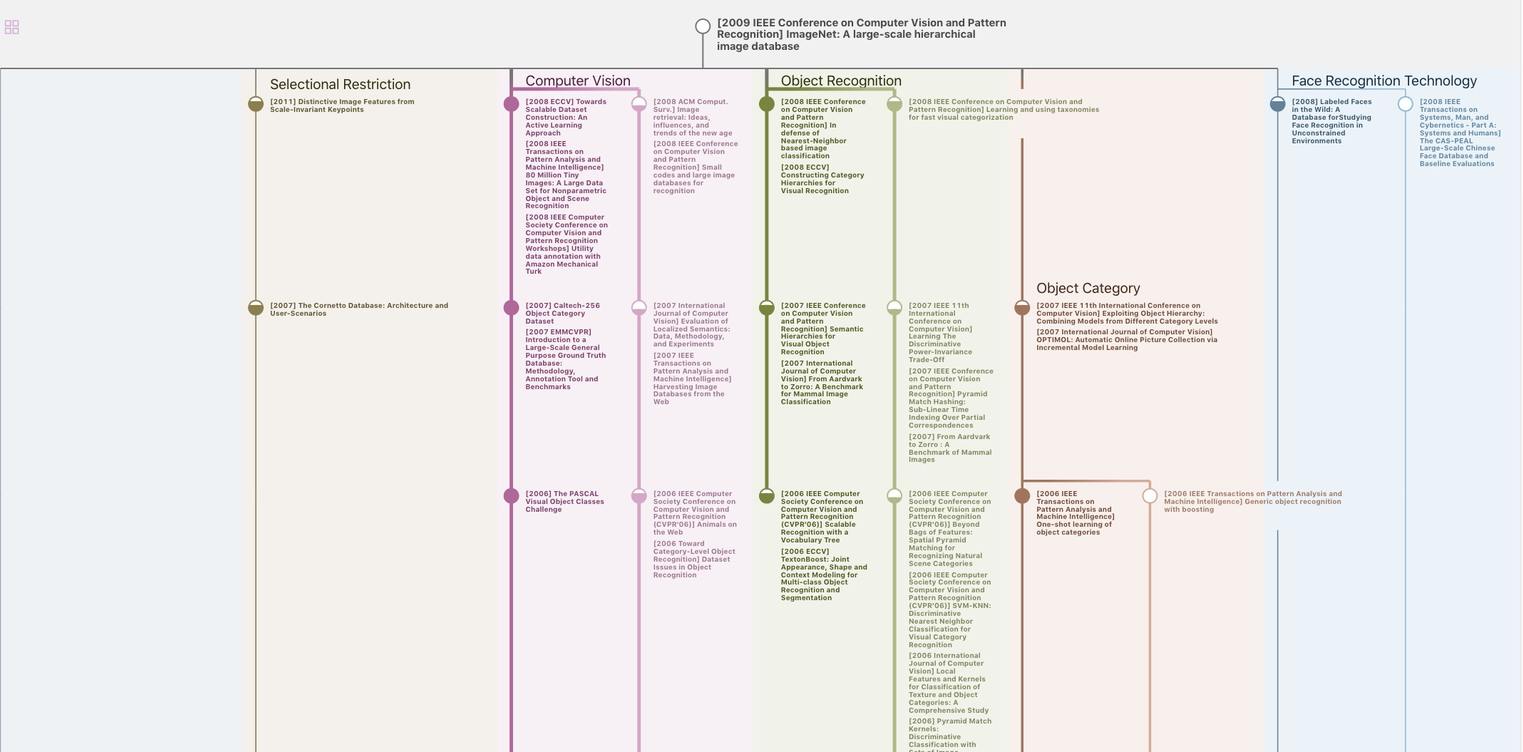
生成溯源树,研究论文发展脉络
Chat Paper
正在生成论文摘要