A Short-Term Rainfall Prediction Model Using Multi-task Convolutional Neural Networks
2017 IEEE International Conference on Data Mining (ICDM)(2017)
摘要
Precipitation prediction, such as short-term rainfall prediction, is a very important problem in the field of meteorological service. In practice, most of recent studies focus on leveraging radar data or satellite images to make predictions. However, there is another scenario where a set of weather features are collected by various sensors at multiple observation sites. The observations of a site are sometimes incomplete but provide important clues for weather prediction at nearby sites, which are not fully exploited in existing work yet. To solve this problem, we propose a multi-task convolutional neural network model to automatically extract features from the time series measured at observation sites and leverage the correlation between the multiple sites for weather prediction via multi-tasking. To the best of our knowledge, this is the first attempt to use multi-task learning and deep learning techniques to predict short-term rainfall amount based on multi-site features. Specifically, we formulate the learning task as an end-to-end multi-site neural network model which allows to leverage the learned knowledge from one site to other correlated sites, and model the correlations between different sites. Extensive experiments show that the learned site correlations are insightful and the proposed model significantly outperforms a broad set of baseline models including the European Centre for Medium-range Weather Forecasts system (ECMWF).
更多查看译文
关键词
Multi-Task Convolutional Neural Networks,Rainfall Prediction,Multi-site Features
AI 理解论文
溯源树
样例
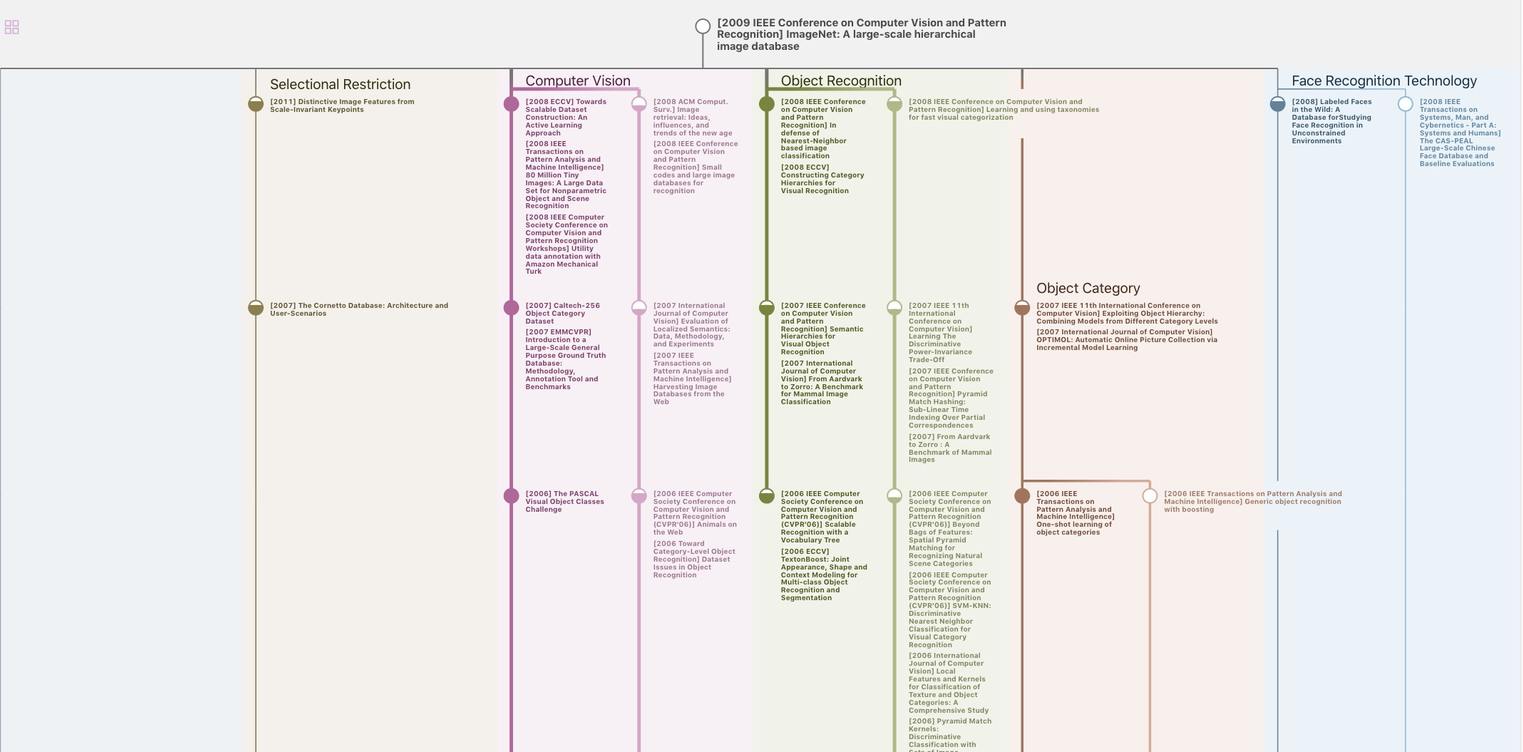
生成溯源树,研究论文发展脉络
Chat Paper
正在生成论文摘要