Data-Driven Anomaly Detection for Power System Generation Control
2017 17TH IEEE INTERNATIONAL CONFERENCE ON DATA MINING WORKSHOPS (ICDMW 2017)(2017)
摘要
In this paper, we propose an online spatiotemporal data-driven methodology to detect malicious cyber attacks that target power system balancing and frequency control. The anomaly detection, which spots abnormal generator behavioral patterns in real time, is achieved locally at a power plant with peer to peer communication capability. We mainly consider the data integrity attack targeting Automatic Generation Control (AGC) and the malicious manipulation of generator set points via cyber intrusions. Behavior conformity metrics are defined as instance features for generators within the same balancing authority (BA) to extract information out of raw telemetry data. We adopt semi-supervised K-means clustering to group each behavioral pattern instances offline, and anomaly detection carries out multi-class classification cyclically during online application based on the model attained. In general, the number of conformity metrics is much smaller compared to that of raw data features, which highly improves the timing performance of anomaly detection. Our experimental studies with the standard IEEE 39 bus system shows that the proposed methodology provides satisfactory accuracy and efficiency by leveraging the domain-specific knowledge in anomaly detection.
更多查看译文
关键词
Balancing and frequency control,generator behavior pattern,conformity metric,attack detection,cyber-physical security
AI 理解论文
溯源树
样例
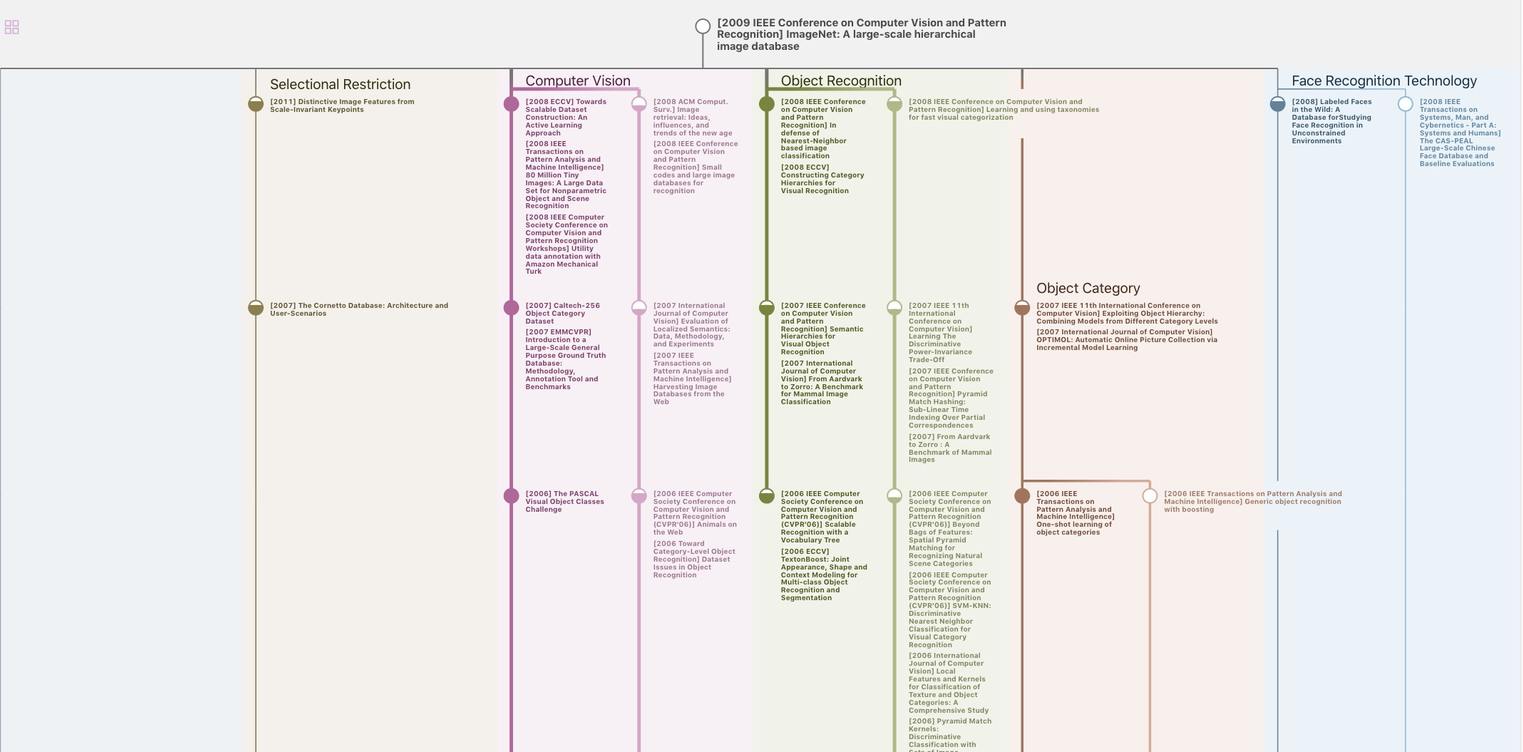
生成溯源树,研究论文发展脉络
Chat Paper
正在生成论文摘要