RESTRAC: REference Sequence Based Space TRAnsformation for Clustering
2017 IEEE International Conference on Data Mining Workshops (ICDMW)(2017)
摘要
Effective mining of large amount of DNA and RNA fragments obtained from next generation sequencing technologies, depends on the availability of efficient analytical tools to process them. One of the important aspects of this analysis, dealing with huge number of fragments, is partitioning them based on their level of similarities. In this paper we propose a space transformation based clustering approach to achieve this partitioning. In this approach, we transform each sequence by a set of reference sequences into a point in a multidimensional vector space and do the clustering in this vector space. We show through extensive analysis that the proposed transformation very closely preserve the clustering properties of the sequences using edit distance. Time for this transformation is linear with the number of sequences. The amount of time saving for this clustering is significant because in this approach edit distance calculations between two sequences are replaced by vector distance calculations between two corresponding feature vectors. We used agglomerative hierarchical clustering using single and average linkage because they are frequently used by the bioinformatics community. Agglomerative hierarchical clustering runs in quadratic time with the number of sequences and clustering time for this approach in the edit space can be prohibitive for large number of sequences. There exists greedy heuristic methods that perform clustering much faster but at the cost of significantly reduced cluster quality. We have applied our method to 16S rRNA fragment datasets obtained from different environmental samples. In these experiments, RESTRAC achieves up to five hundred times speed-up for single linkage and up to five times speed-up for average linkage while preserving good cluster quality.
更多查看译文
关键词
Space Transformation,OTU clustering
AI 理解论文
溯源树
样例
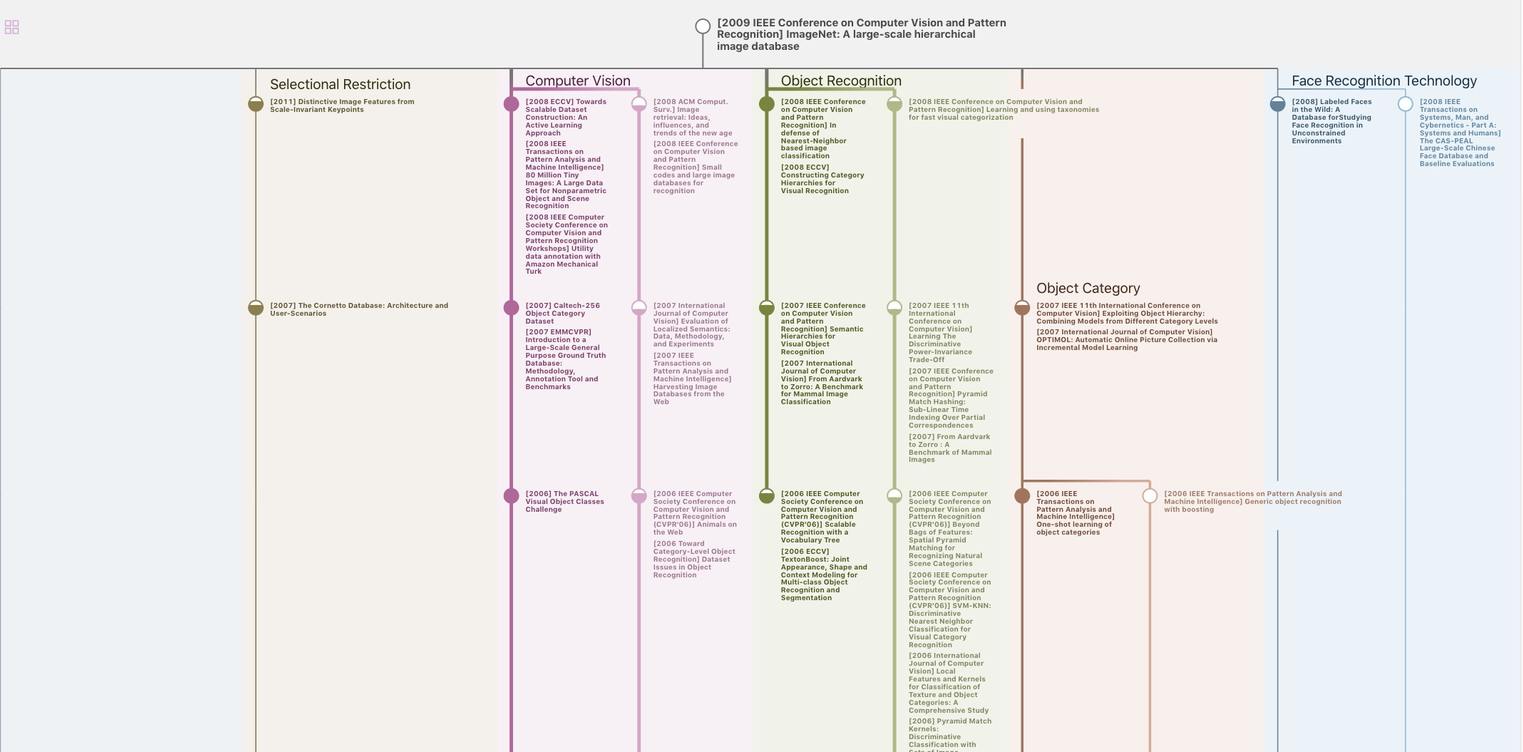
生成溯源树,研究论文发展脉络
Chat Paper
正在生成论文摘要