Situation Aware Multi-task Learning for Traffic Prediction
2017 IEEE International Conference on Data Mining (ICDM)(2017)
摘要
Due to the recent vast availability of transportation traffic data, major research efforts have been devoted to traffic prediction, which is useful in many applications such as urban planning, traffic management and navigations systems. Current prediction methods that independently train a model per traffic sensor cannot accurately predict traffic in every situation (e.g., rush hours, constructions and accidents) because there may not exist sufficient training samples per sensor for all situations. To address this shortcoming, our core idea is to explore the commonalities of prediction tasks across multiple sensors who behave similarly in a specific traffic situation. Instead of building a model independently per sensor, we propose a Multi-Task Learning (MTL) framework that aims to first automatically identify the traffic situations and then simultaneously build one forecasting model for similar-behaving sensors per traffic situation. The key innovation here is that instead of the straightforward application of MTL where each "task" corresponds to a sensor, we relate each MTL's "task" to a traffic situation. Specifically, we first identify these traffic situations by running clustering algorithms on all sensors' data. Subsequently, to enforce the commonalities under each identified situation, we use the group Lasso regularization in MTL to select a common set of features for the prediction tasks, and we adapt efficient FISTA algorithm with guaranteed convergence rate. We evaluated our methods with a large volume of real-world traffic sensor data; our results show that by incorporating traffic situations, our proposed MTL framework performs consistently better than naively applying MTL per sensor. Moreover, our holistic approach, under different traffic situations, outperforms all the best traffic prediction approaches for a given situation by up to 18% and 30% in short and long term predictions, respectively.
更多查看译文
关键词
traffic prediction,traffic situation clustering,situation-aware multi-task learning,long term traffic forecasting
AI 理解论文
溯源树
样例
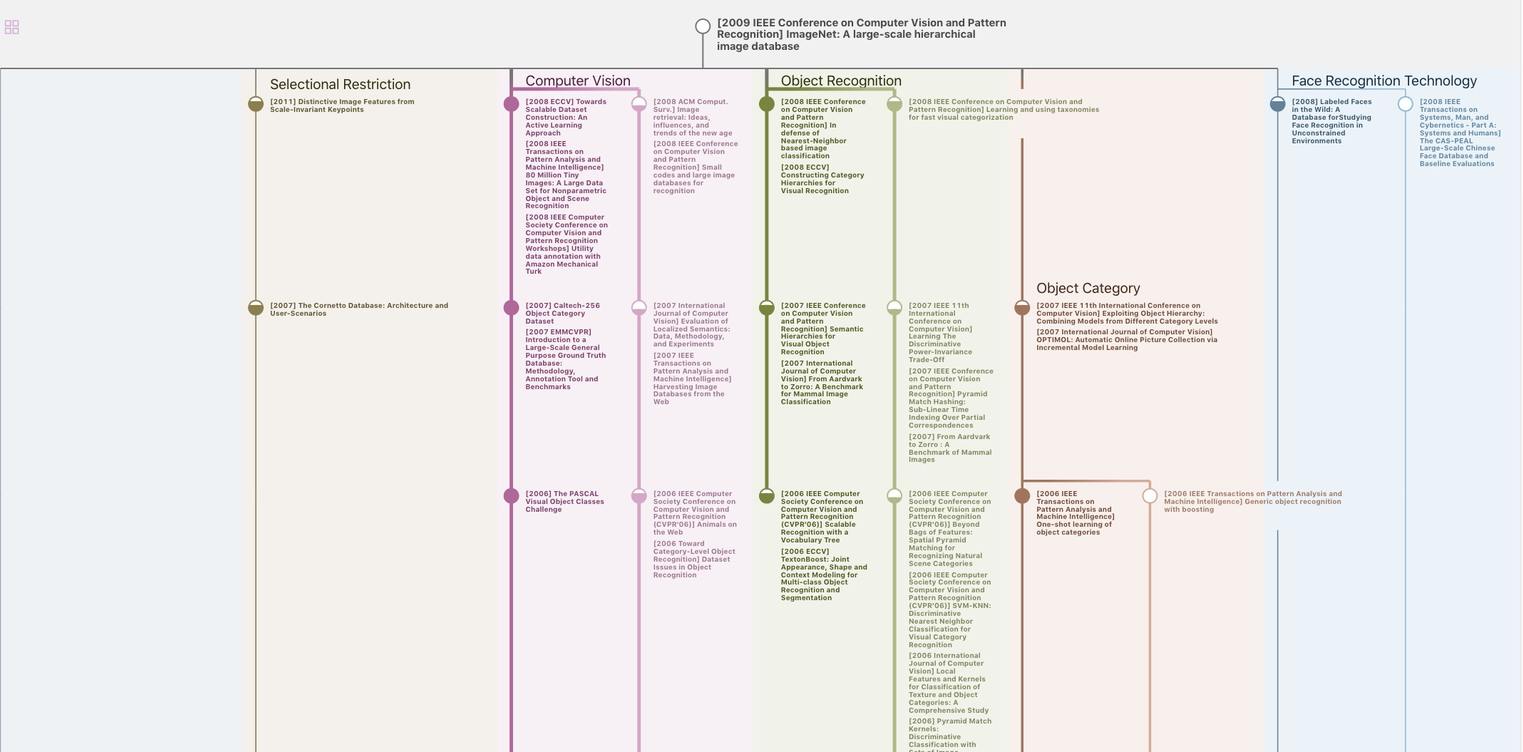
生成溯源树,研究论文发展脉络
Chat Paper
正在生成论文摘要