Spectral-Spatial Subspace Clustering For Hyperspectral Images Via Modulated Low-Rank Representation
2017 IEEE INTERNATIONAL GEOSCIENCE AND REMOTE SENSING SYMPOSIUM (IGARSS)(2017)
摘要
In this paper, a novel spectral-spatial low-rank subspace clustering (SS-LRSC) algorithm is presented for clustering of hyperspectral images (HSI). Generally, employing the traditional LRSC framework directly cannot fully exploit the sample correlations in original spatial domain. Therefore, the proposed method utilizes a novel modulation strategy to modify the low rank representation matrix, which largely exploits the structure correlations. Specifically, a spectral and representation similarity weighted matrix is first applied to modulate the representation matrix; another local spatial bilateral filtering based modulation is further incorporated. Finally, the modulation method is integrated into the LRSC framework. Benefitting from the ability of the modulation method, SS-LRSC can capture both the structure correlations and inherent feature information of the data, which provides a competitive subspace clustering for HSI. Several experiments were conducted to illustrate the performance of the proposed algorithm.
更多查看译文
关键词
hyperspectral image, low-rank representation, spectral clustering, subspace clustering, spatial information
AI 理解论文
溯源树
样例
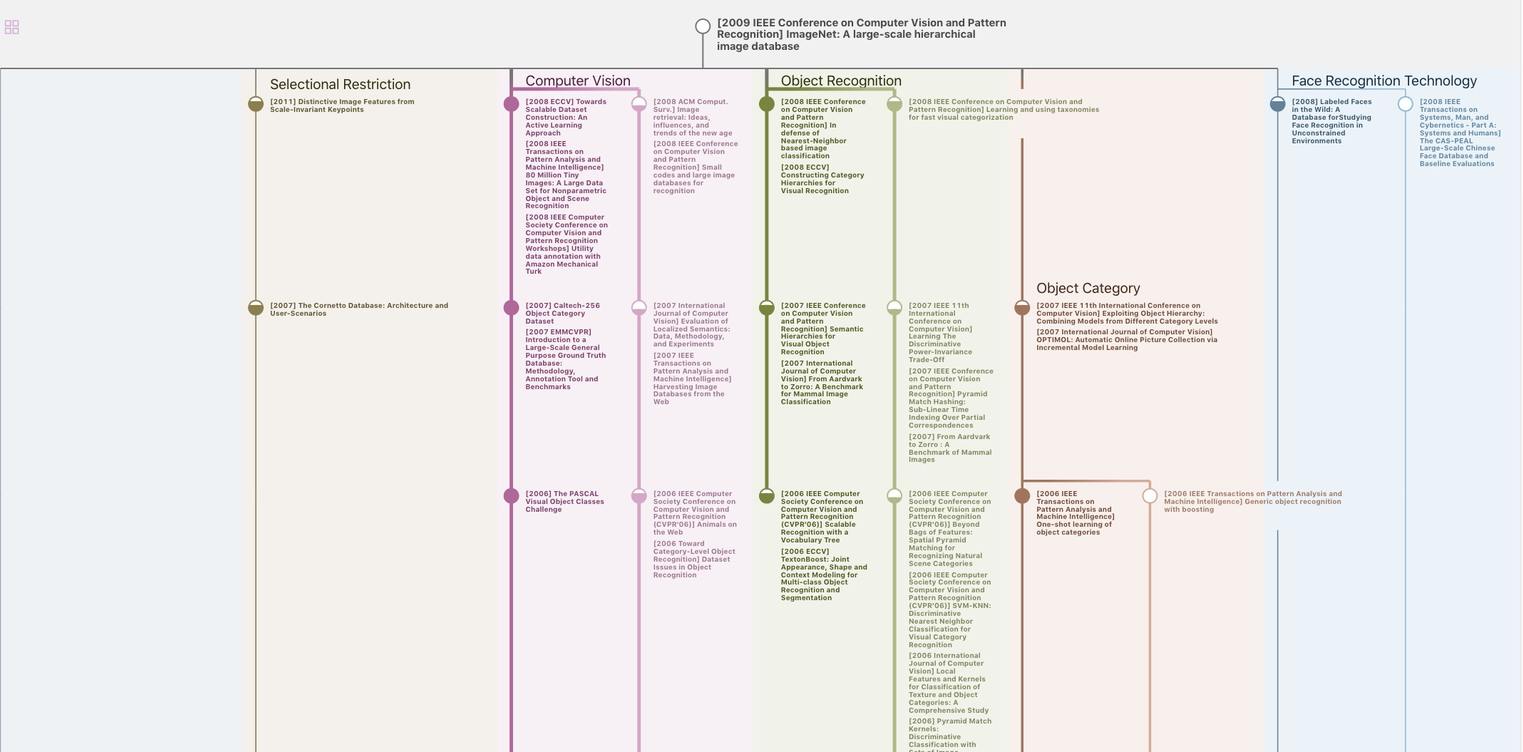
生成溯源树,研究论文发展脉络
Chat Paper
正在生成论文摘要