Small-sample classification of hyperspectral data in a graph-based semi-supervision framwork
2017 IEEE INTERNATIONAL GEOSCIENCE AND REMOTE SENSING SYMPOSIUM (IGARSS)(2017)
摘要
Using spectra of hyperspectral remote sensing imagery to identify and classify land cover has been a hot topic thanks for its high resolution spectrum. However, when the quantity of labeled samples is too small, the classification accuracy of hyperspectral data will be reduced greatly. Most classification algorithms take dimensional reduction strategy and require plentiful labeled samples in order to learn the classifier that could then recognize a specific material. But in most remote sensing situations labeling samples is a costly task and the valued information would be lost with dimensional reduction. Sparse representation as a fast and effective algorithm has the advantages that it can perform quite well with small labeled samples without dimensional reduction, Therefore, we proposed a new framework to construct a graph-based semi-supervised model to solve paucity problem of labeled samples and combine the k nearest neighbor (knn) graph to take the advantage of space features. In this new model, sparse representation is used to build the probability matrix by estimating if a pairwise pixels belonging to the same class, and this probability matrix is integrated into l(1) norm graph to form a more discriminating graph called dl(1) graph. Then we combine this dl(1) graph with knn graph in proportion. The new graph can employ both spectral values and space information of hyperspectral data. We demonstrate the effectiveness of our proposal on the Indiana Pines hyperspectral data set and the results outperform state of the art.
更多查看译文
关键词
Hyperspectral data classification,sparse representation,small sample analysis,graph-based,semi-supervision learning
AI 理解论文
溯源树
样例
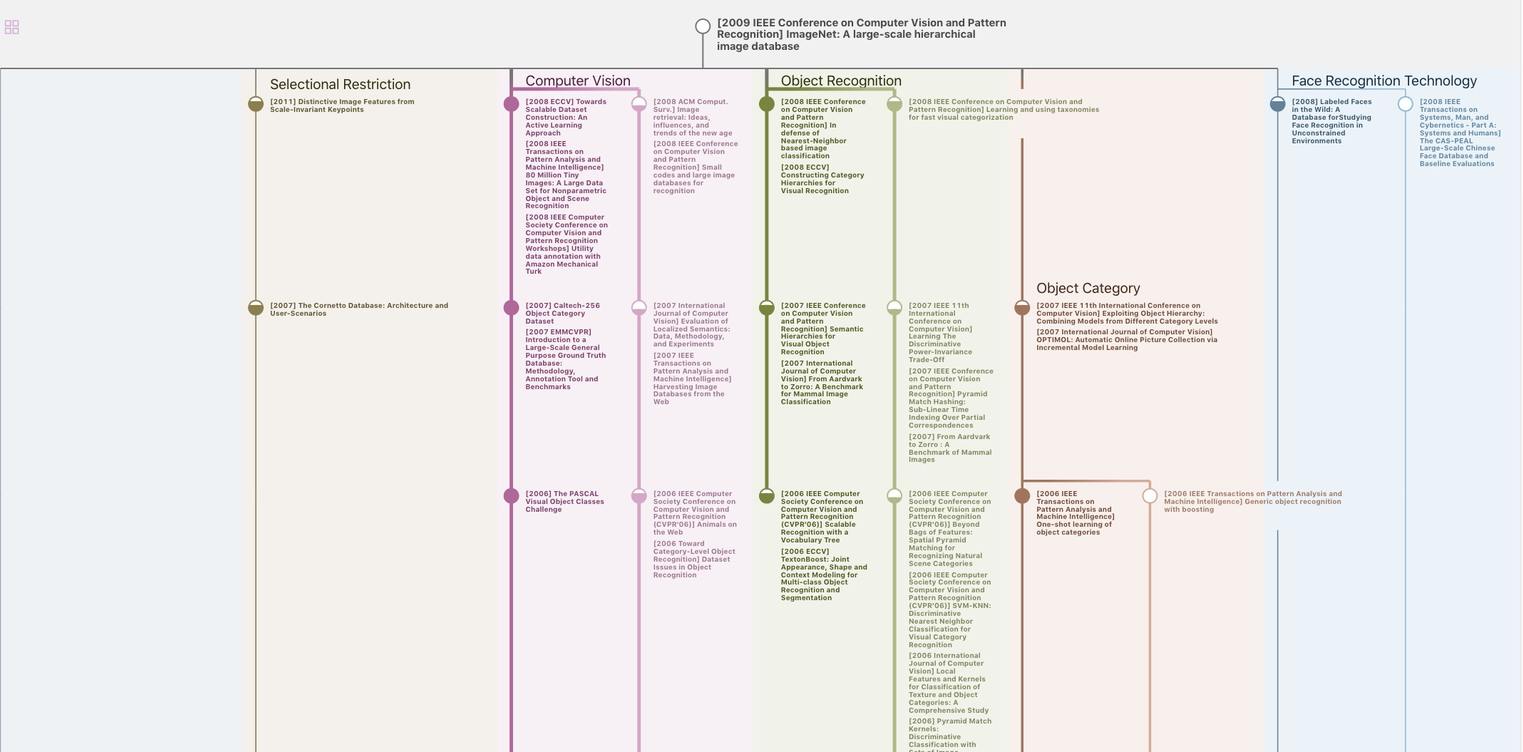
生成溯源树,研究论文发展脉络
Chat Paper
正在生成论文摘要