Robust real-time visual tracking using dual-frame deep comparison network integrated with correlation filters
2017 IEEE/RSJ INTERNATIONAL CONFERENCE ON INTELLIGENT ROBOTS AND SYSTEMS (IROS)(2017)
摘要
In recent years, applications of visual tracking algorithms has seen a substantial growth with deployments in intelligent robots such as drones for human tracking. The algorithms for such tasks has to be efficient in terms of computational cost while been robust, accurate and fast. Object tracking algorithms based on handcrafted heuristics and constraints are widely used in uav applications. The handcrafted heuristics are mostly implemented for task-oriented applications which limits the extensions in uav's capability beyond the predefined functions. This paper considers the challenges of tracking and landing an autonomous uav on a speed high moving target, and presents a visual tracking algorithm that integrates correlation filters with deep comparison network for real-time tracking with state-of-the-art accuracy. The method first tracks the target upto translation using an online learnt model via local search technique. The changes in scale is estimated by a deep comparison network (DCN) instead of the commonly used pyramidal approach. In a single network evaluation, DCN can estimate the changes in scale as well as compensate the drifting of the tracker by refining the object region estimated by the correlation filters. The network is end-to-end trained which attempts to learn a powerful matching function for object localization using a known template. Generally, the integrated framework can be viewed as coarse-to-fine level motion estimation. Moreover, the framework can redetect the lost target without a need for a separate detector.
更多查看译文
关键词
Kernalized correlation filters (KCF),deep comparison network (DCN),object tracking
AI 理解论文
溯源树
样例
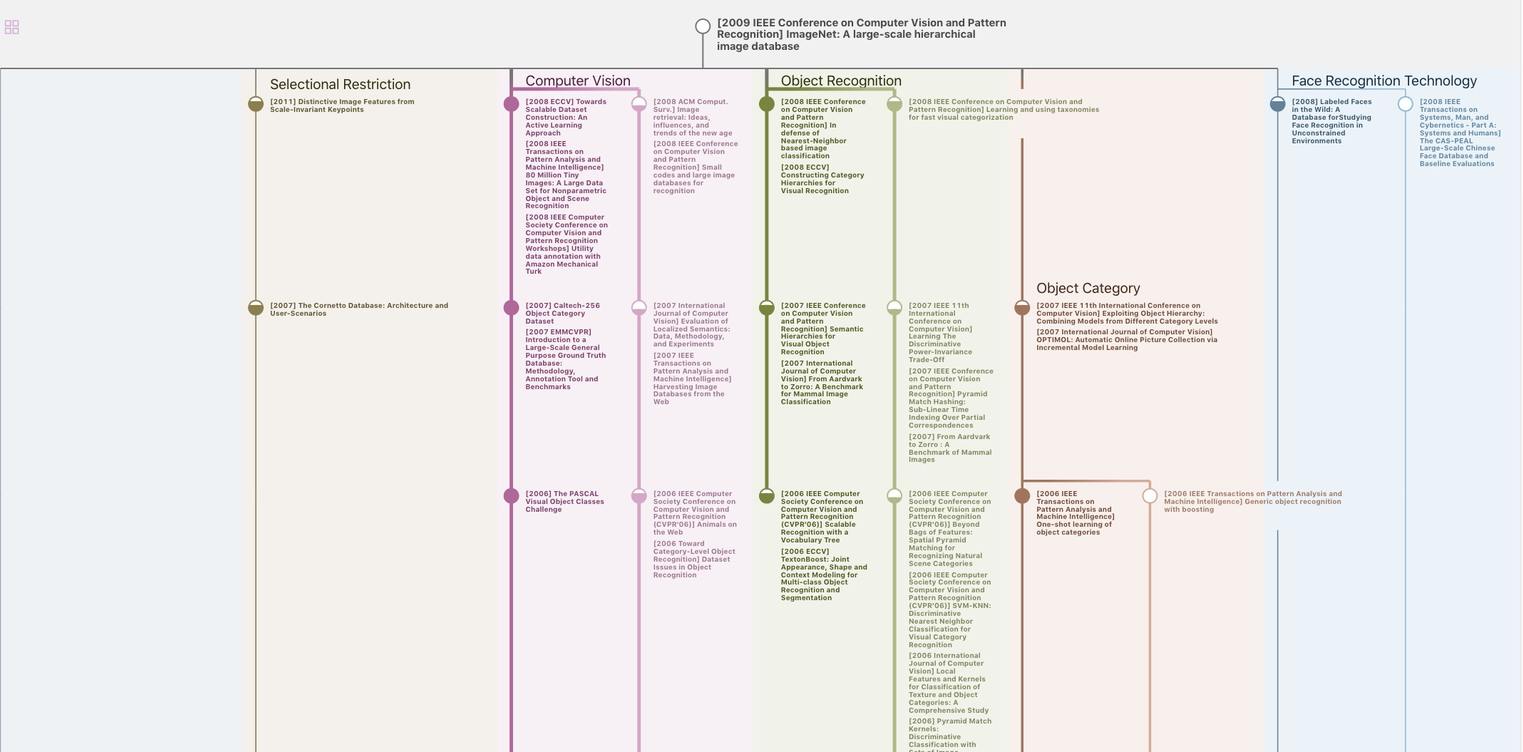
生成溯源树,研究论文发展脉络
Chat Paper
正在生成论文摘要