Toward Autonomous Mapping And Exploration For Mobile Robots Through Deep Supervised Learning
2017 IEEE/RSJ INTERNATIONAL CONFERENCE ON INTELLIGENT ROBOTS AND SYSTEMS (IROS)(2017)
摘要
We consider an autonomous mapping and exploration problem in which a range-sensing mobile robot is guided by an information-based controller through an a priori unknown environment, choosing to collect its next measurement at the location estimated to yield the maximum information gain within its current field of view. We propose a novel and time-efficient approach to predict the most informative sensing action using a deep neural network. After training the deep neural network on a series of thousands of randomly-generated "dungeon maps", the predicted optimal sensing action can be computed in constant time, with prospects for appealing scalability in the testing phase to higher dimensional systems. We evaluated the performance of deep neural networks on the autonomous exploration of two-dimensional workspaces, comparing several different neural networks that were selected due to their success in recent ImageNet challenges. Our computational results demonstrate that the proposed method provides high efficiency as well as accuracy in selecting informative sensing actions that support autonomous mobile robot exploration.
更多查看译文
关键词
deep neural network,autonomous exploration,informative sensing action,autonomous mobile robot exploration,deep supervised learning,autonomous mapping,range-sensing mobile robot,maximum information gain,time-efficient approach,dungeon maps,predicted optimal sensing action
AI 理解论文
溯源树
样例
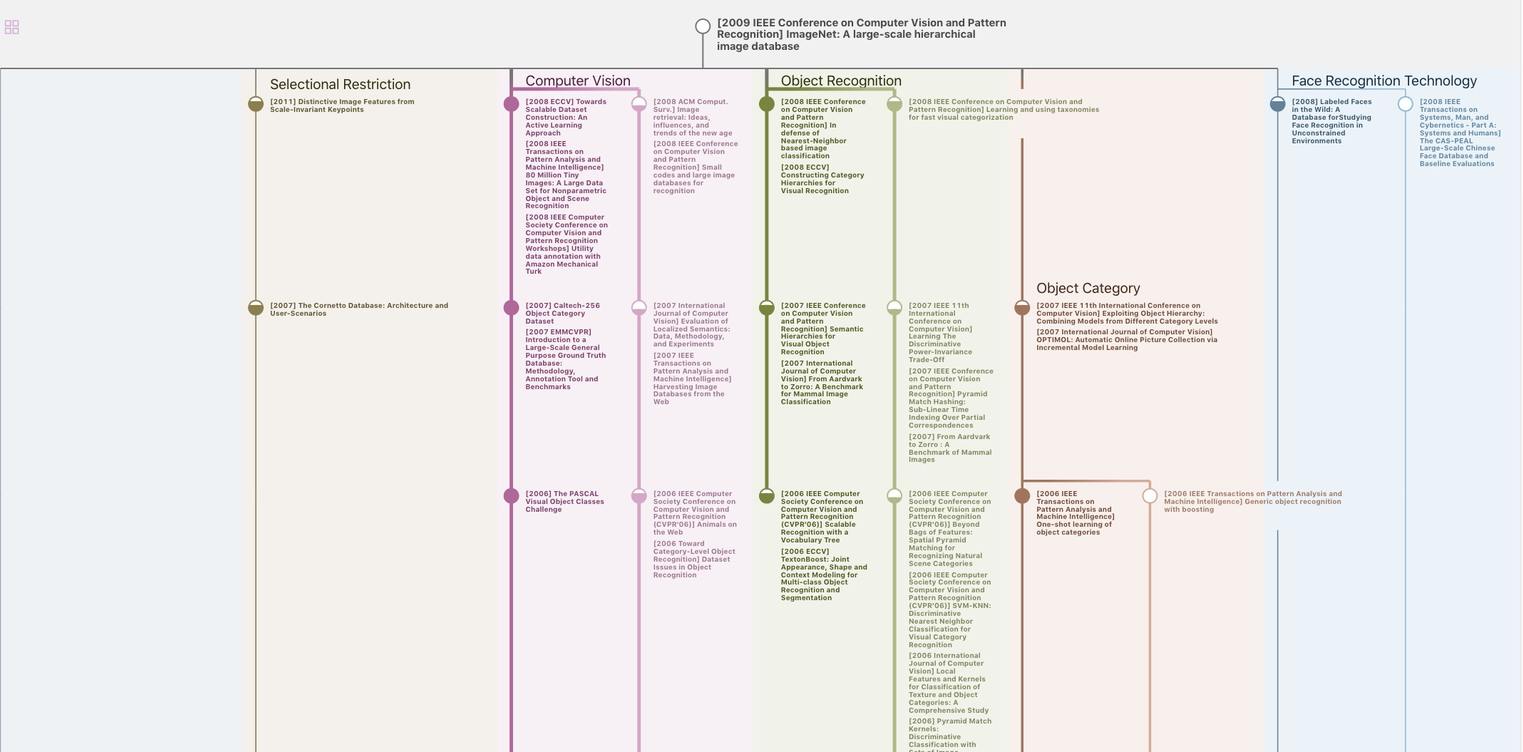
生成溯源树,研究论文发展脉络
Chat Paper
正在生成论文摘要