Leveraging Deep Neural Networks With Nonnegative Representations For Improved Environmental Sound Classification
2017 IEEE 27TH INTERNATIONAL WORKSHOP ON MACHINE LEARNING FOR SIGNAL PROCESSING(2017)
摘要
This paper introduces the use of representations based on non-negative matrix factorization (NMF) to train deep neural networks with applications to environmental sound classification. Deep learning systems for sound classification usually rely on the network to learn meaningful representations from spectrograms or hand-crafted features. Instead, we introduce a NMF-based feature learning stage before training deep networks, whose usefulness is highlighted in this paper, especially for multi-source acoustic environments such as sound scenes. We rely on two established unsupervised and supervised NMF techniques to learn better input representations for deep neural networks. This will allow us, with simple architectures, to reach competitive performance with more complex systems such as convolutional networks for acoustic scene classification. The proposed systems outperform neural networks trained on time-frequency representations on two acoustic scene classification datasets as well as the best systems from the 2016 DCASE challenge.
更多查看译文
关键词
Nonnegative Matrix Factorization, Deep Neural Networks, Sound Classification
AI 理解论文
溯源树
样例
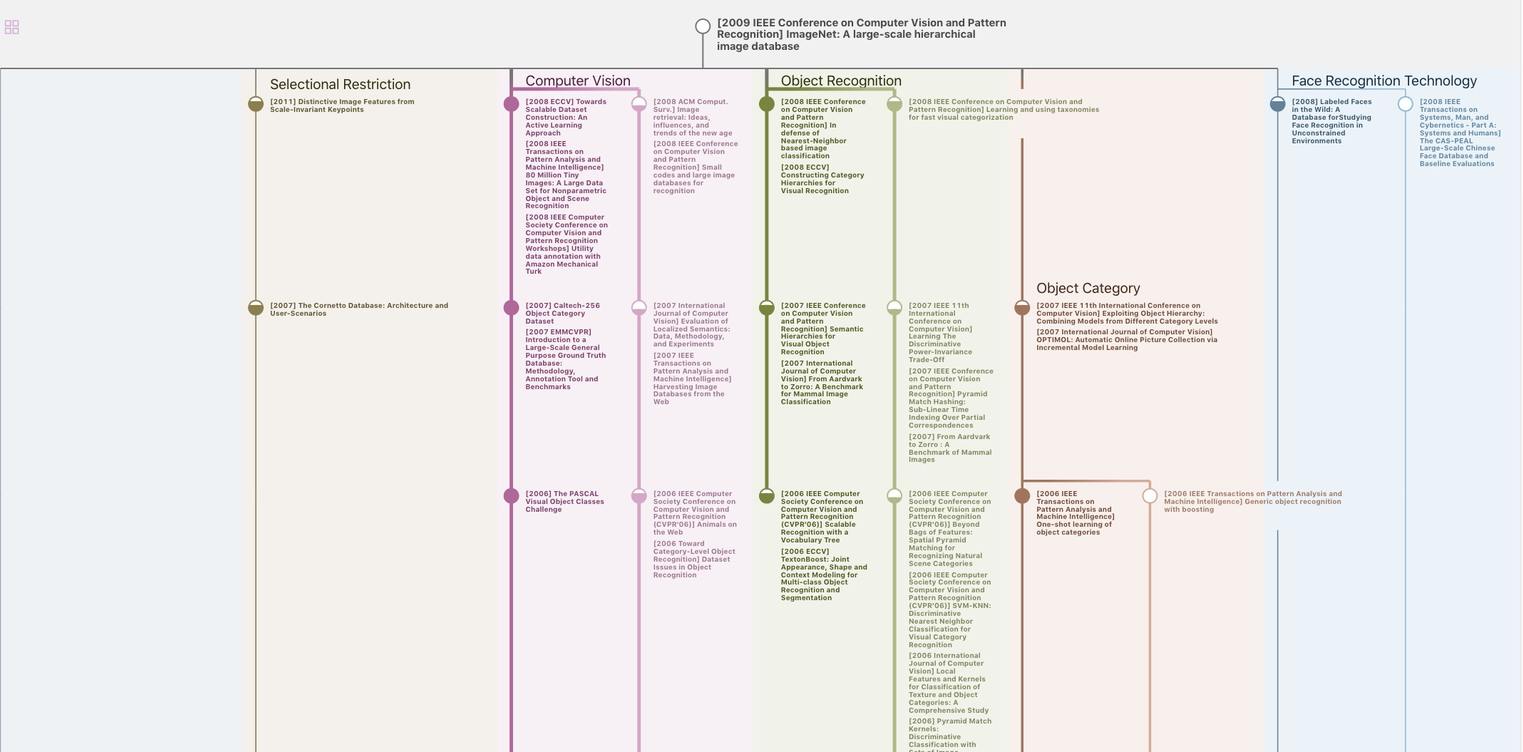
生成溯源树,研究论文发展脉络
Chat Paper
正在生成论文摘要