Quantization For Low-Rank Matrix Recovery
INFORMATION AND INFERENCE-A JOURNAL OF THE IMA(2019)
摘要
We study Sigma-Delta quantization methods coupled with appropriate reconstruction algorithms for digitizing randomly sampled low-rank matrices. We show that the reconstruction error associated with our methods decays polynomially with the oversampling factor, and we leverage our results to obtain root-exponential accuracy by optimizing over the choice of quantization scheme. Additionally, we show that a random encoding scheme, applied to the quantized measurements, yields a near-optimal exponential bit rate. As an added benefit, our schemes are robust both to noise and to deviations from the low-rank assumption. In short, we provide a full generalization of analogous results, obtained in the classical setup of band-limited function acquisition, and more recently, in the finite frame and compressed sensing setups to the case of low-rank matrices sampled with sub-Gaussian linear operators. Finally, we believe our techniques for generalizing results from the compressed sensing setup to the analogous low-rank matrix setup is applicable to other quantization schemes.
更多查看译文
关键词
compressed sensing, quantization, exponential accuracy, rate-distortion, low-rank, 1-bit
AI 理解论文
溯源树
样例
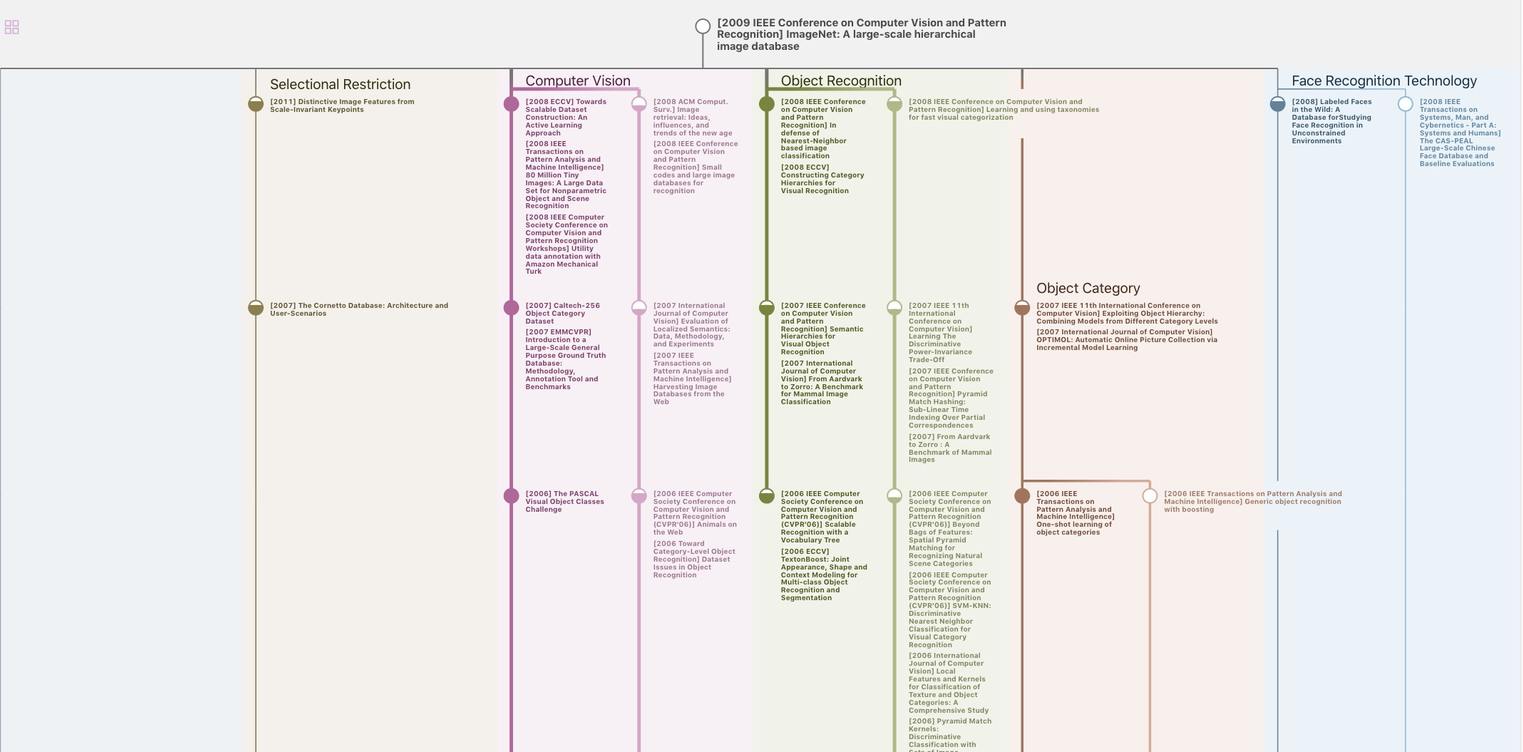
生成溯源树,研究论文发展脉络
Chat Paper
正在生成论文摘要