GANs for Biological Image Synthesis
2017 IEEE International Conference on Computer Vision (ICCV)(2017)
摘要
In this paper, we propose a novel application of Generative Adversarial Networks (GAN) to the synthesis of cells imaged by fluorescence microscopy. Compared to natural images, cells tend to have a simpler and more geometric global structure that facilitates image generation. However, the correlation between the spatial pattern of different fluorescent proteins reflects important biological functions, and synthesized images have to capture these relationships to be relevant for biological applications. We adapt GANs to the task at hand and propose new models with casual dependencies between image channels that can generate multi-channel images, which would be impossible to obtain experimentally. We evaluate our approach using two independent techniques and compare it against sensible baselines. Finally, we demonstrate that by interpolating across the latent space we can mimic the known changes in protein localization that occur through time during the cell cycle, allowing us to predict temporal evolution from static images.
更多查看译文
关键词
GAN,image channels,multichannel images,cell cycle,static images,biological image synthesis,Generative Adversarial Networks,fluorescence microscopy,natural images,image generation,spatial pattern,synthesized images,biological applications,fluorescent proteins,biological functions,geometric global structure
AI 理解论文
溯源树
样例
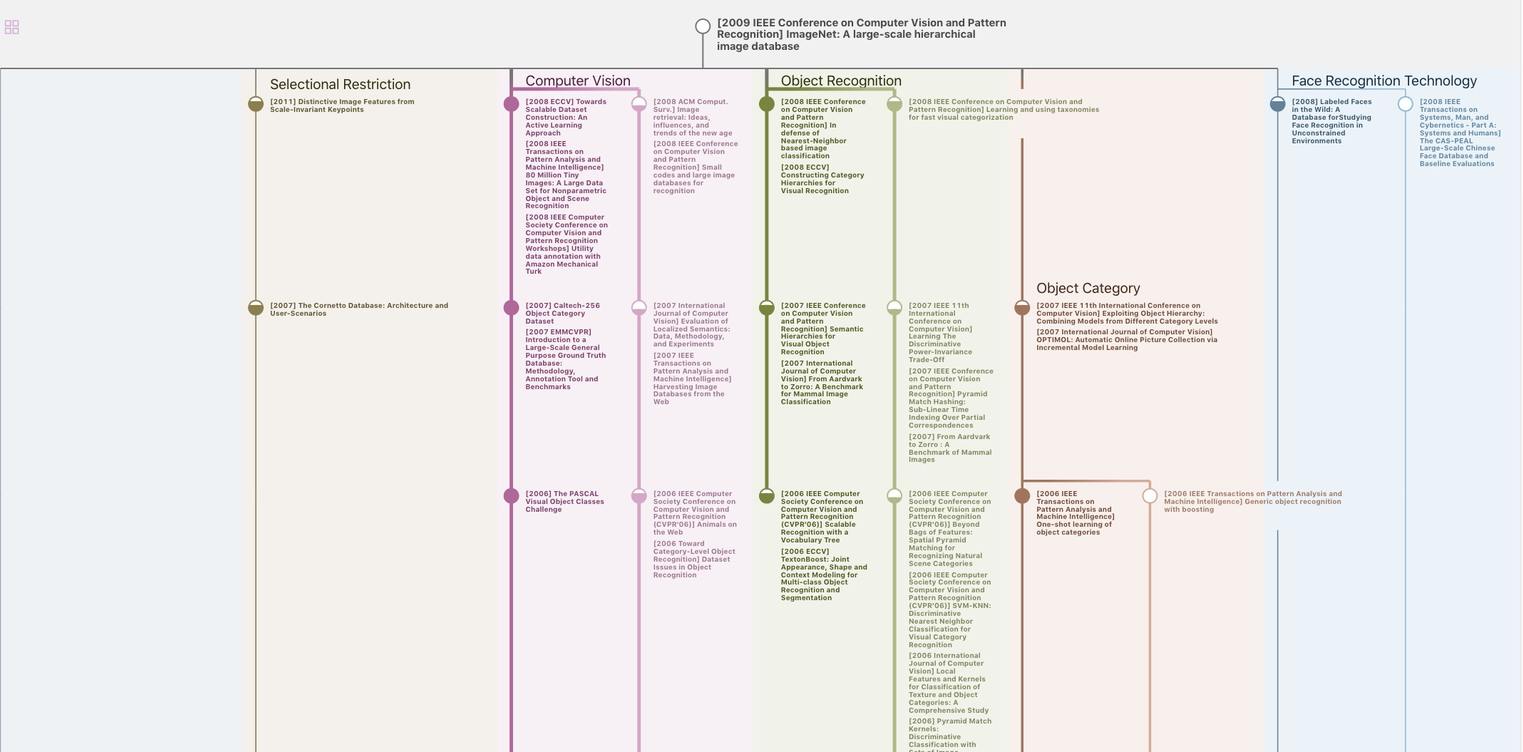
生成溯源树,研究论文发展脉络
Chat Paper
正在生成论文摘要