Two-stream Collaborative Learning with Spatial-Temporal Attention for Video Classification.
IEEE Transactions on Circuits and Systems for Video Technology(2019)
摘要
Video classification is highly important and has widespread applications, such as video search and intelligent surveillance. Video naturally contains both static and motion information, which can be represented by frames and optical flow, respectively. Recently, researchers have generally adopted deep networks to capture the static and motion information
separately
, which has two main limitations. First, the coexistence relationship between spatial and temporal attention is ignored, although they should be jointly modeled as the spatial and temporal evolutions of video to learn discriminative video features. Second, the strong complementarity between static and motion information is ignored, although they should be collaboratively learned to enhance each other. To address the above two limitations, this paper proposes the two-stream collaborative learning with spatial-temporal attention (TCLSTA) approach, which consists of two models. First, for the
spatial-temporal attention model
, the spatial-level attention emphasizes the salient regions in a frame, and the temporal-level attention exploits the discriminative frames in a video. They are mutually enhanced to jointly learn the discriminative static and motion features for better classification performance. Second, for the
static-motion collaborative model
, it not only achieves mutual guidance between static and motion information to enhance the feature learning but also adaptively learns the fusion weights of static and motion streams, thus exploiting the strong complementarity between static and motion information to improve video classification. Experiments on four widely used data sets show that our TCLSTA approach achieves the best performance compared with more than 10 state-of-the-art methods.
更多查看译文
关键词
Feature extraction,Adaptation models,Video sequences,Collaboration,Semantics,Collaborative work,Weapons
AI 理解论文
溯源树
样例
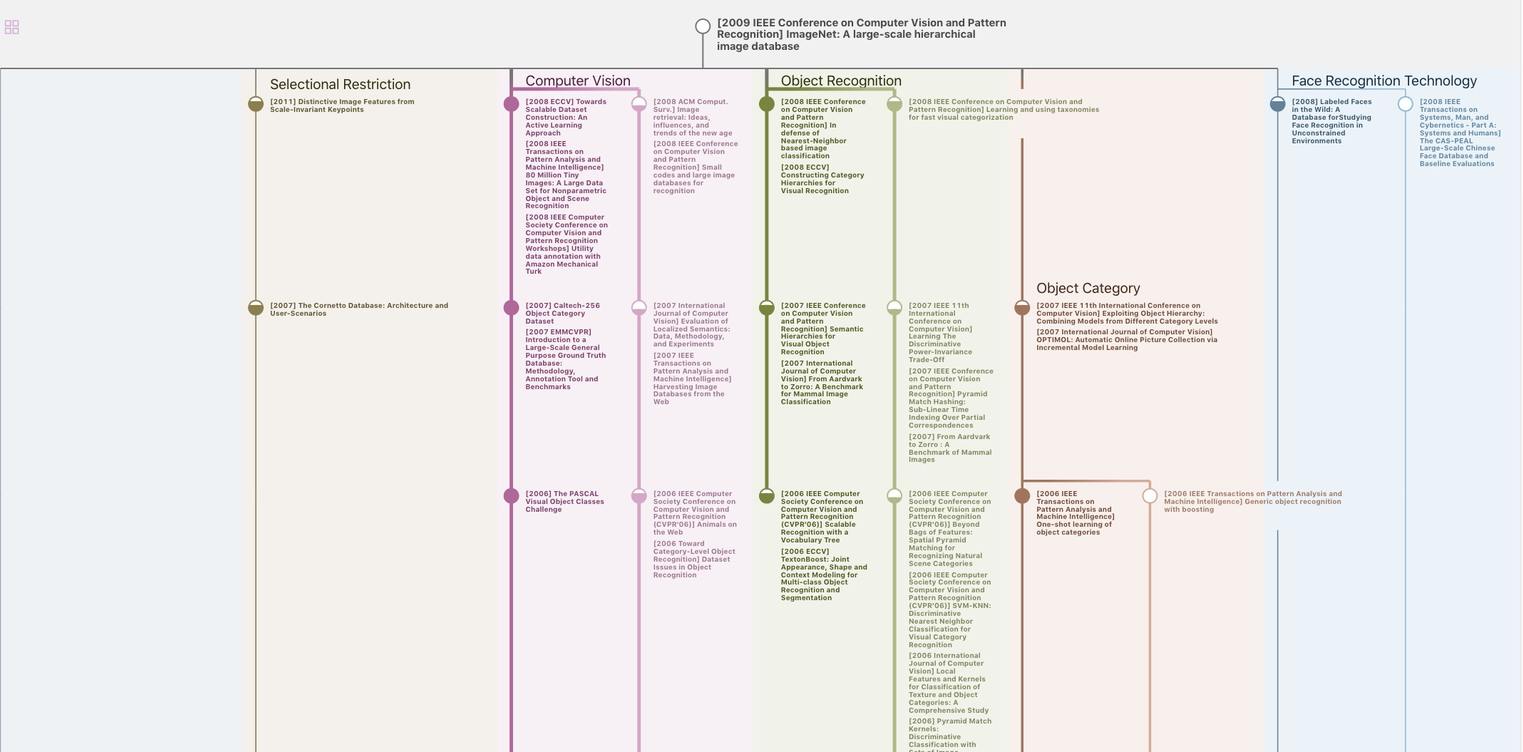
生成溯源树,研究论文发展脉络
Chat Paper
正在生成论文摘要