Learning Sensor Feedback Models from Demonstrations via Phase-Modulated Neural Networks
2018 IEEE International Conference on Robotics and Automation (ICRA)(2018)
摘要
In order to robustly execute a task under environmental uncertainty, a robot needs to be able to reactively adapt to changes arising in its environment. The environment changes are usually reflected in deviation from expected sensory traces. These deviations in sensory traces can be used to drive the motion adaptation, and for this purpose, a feedback model is required. The feedback model maps the deviations in sensory traces to the motion plan adaptation. In this paper, we develop a general data-driven framework for learning a feedback model from demonstrations. We utilize a variant of a radial basis function network structure --with movement phases as kernel centers-- which can generally be applied to represent any feedback models for movement primitives. To demonstrate the effectiveness of our framework, we test it on the task of scraping on a tilt board. In this task, we are learning a reactive policy in the form of orientation adaptation, based on deviations of tactile sensor traces. As a proof of concept of our method, we provide evaluations on an anthropomorphic robot. A video demonstrating our approach and its results can be seen in https://youtu.be/7Dx5imy1Kcw
更多查看译文
关键词
phase-modulated neural networks,feedback model maps,motion plan adaptation,radial basis function network structure,tactile sensor traces,data-driven framework,anthropomorphic robot
AI 理解论文
溯源树
样例
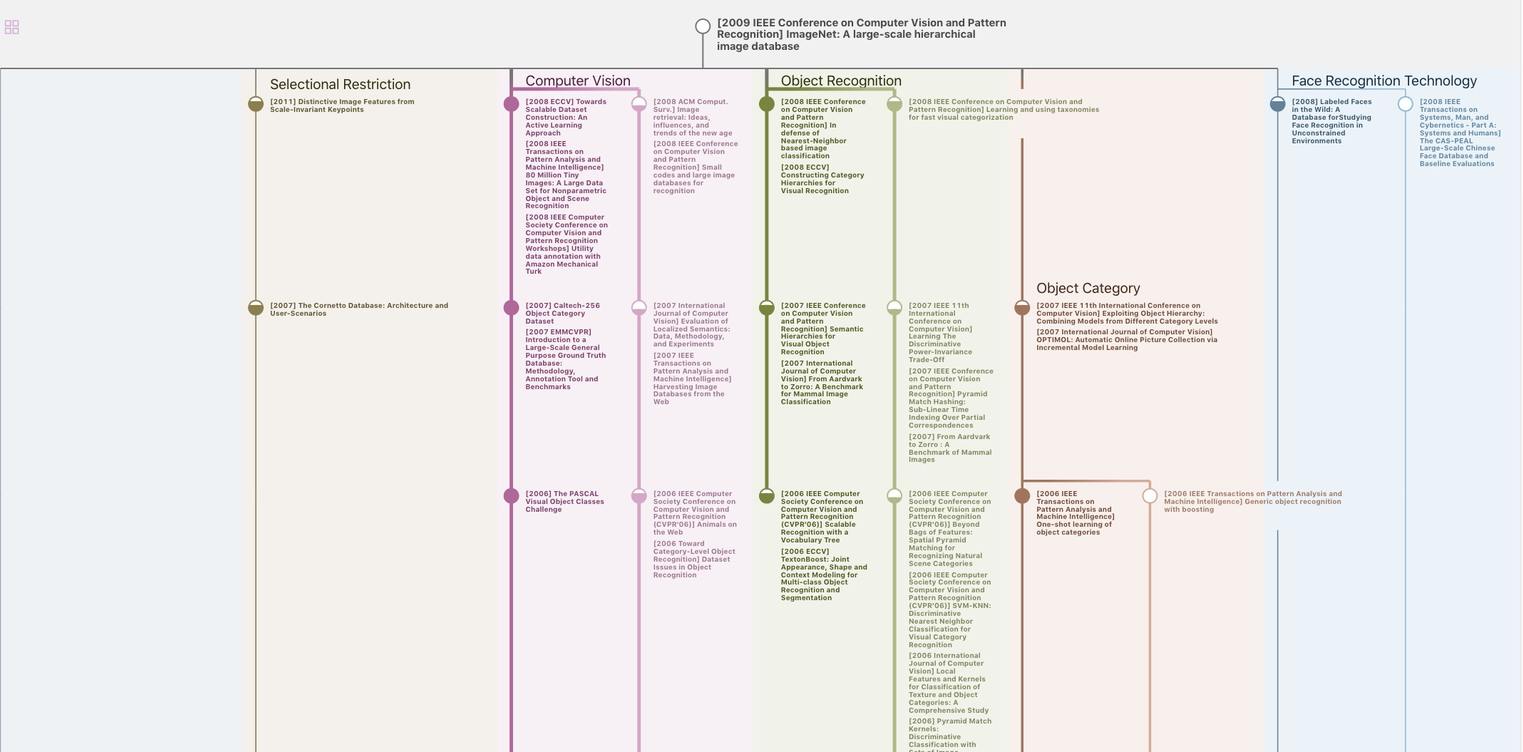
生成溯源树,研究论文发展脉络
Chat Paper
正在生成论文摘要