A representer theorem for deep kernel learning.
Journal of Machine Learning Research(2019)
Abstract
In this paper we provide a representer theorem for the concatenation of (linear combinations of) kernel functions of reproducing kernel Hilbert spaces. This result serves as mathematical foundation for the analysis of machine learning algorithms based on compositions of functions. As a direct consequence, the corresponding infinite-dimensional minimization problems can be recast into (nonlinear) finite-dimensional minimization problems, which can be tackled with nonlinear optimization algorithms. Moreover, we show how concatenated machine learning problems can be reformulated as neural networks and how our representer theorem applies to a broad class of state-of-the-art deep learning methods.
MoreTranslated text
Key words
deep kernel learning,representer theorem,artificial neural networks,multi-layer kernel,regularized least-squares regression
AI Read Science
Must-Reading Tree
Example
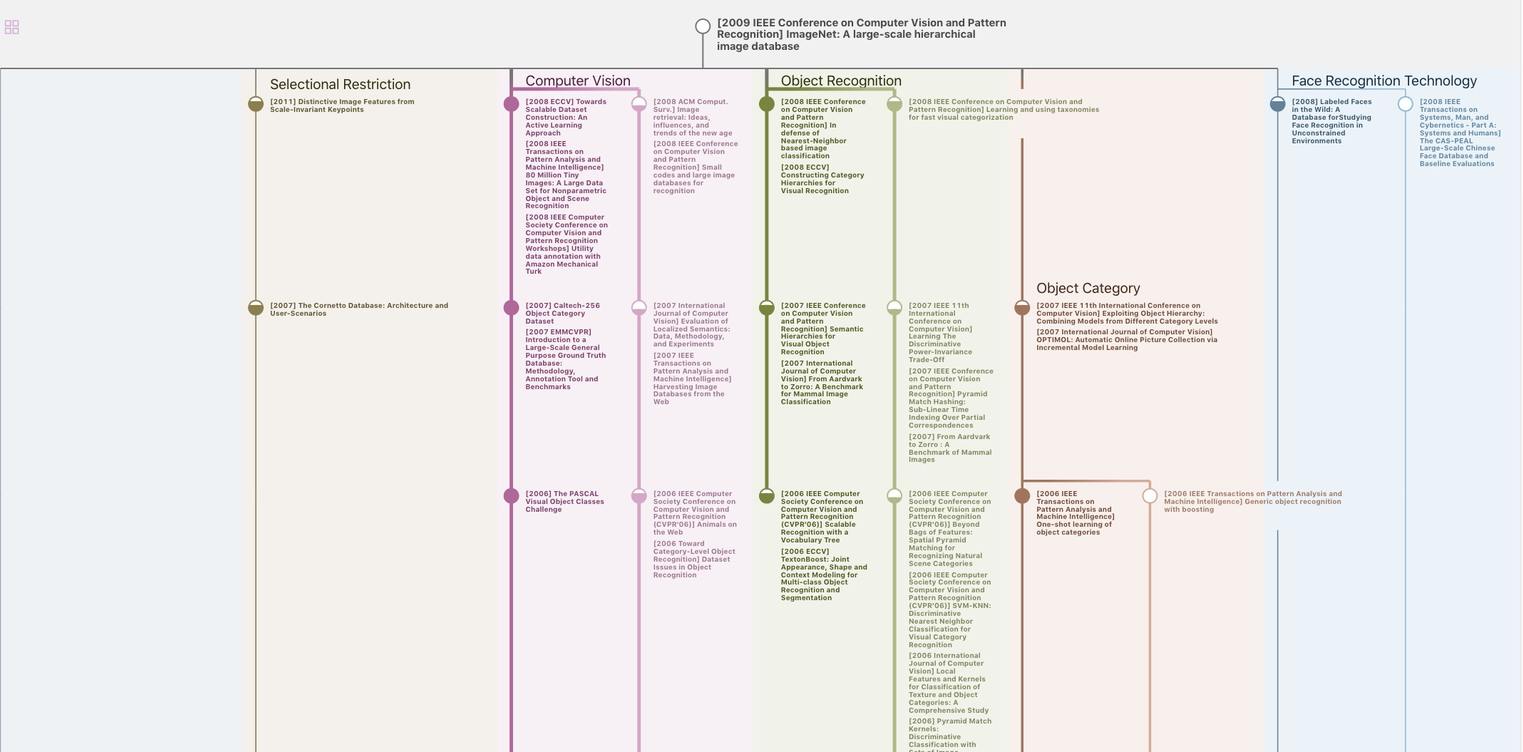
Generate MRT to find the research sequence of this paper
Chat Paper
Summary is being generated by the instructions you defined