Energy-Aware Task Scheduling By A True Online Reinforcement Learning In Wireless Sensor Networks
INTERNATIONAL JOURNAL OF SENSOR NETWORKS(2017)
Abstract
Wireless sensor networks (WSNs) are an attractive platform for various pervasive computing applications. A typical WSN application is composed of different tasks. In this paper, an energy-aware task scheduling method is proposed to achieve better energy consumption/performance trade-off. The proposed method exploits a true online reinforcement learning algorithm. This method is compared with the existing approaches exploiting online learning: distributed independent reinforcement learning (DIRL), reinforcement learning (RL), cooperative reinforcement learning (CRL) and exponential weight for exploration and exploitation (Exp3), in terms of tracking quality/energy consumption trade-off. Simulation results show that our proposed method outperforms existing methods for the target tracking application.
MoreTranslated text
Key words
WSNs, wireless sensor networks, task scheduling, energy-awareness, true online reinforcement learning, DIRL, distributed independent reinforcement learning, CRL, cooperative reinforcement learning, adversarial bandit solvers
AI Read Science
Must-Reading Tree
Example
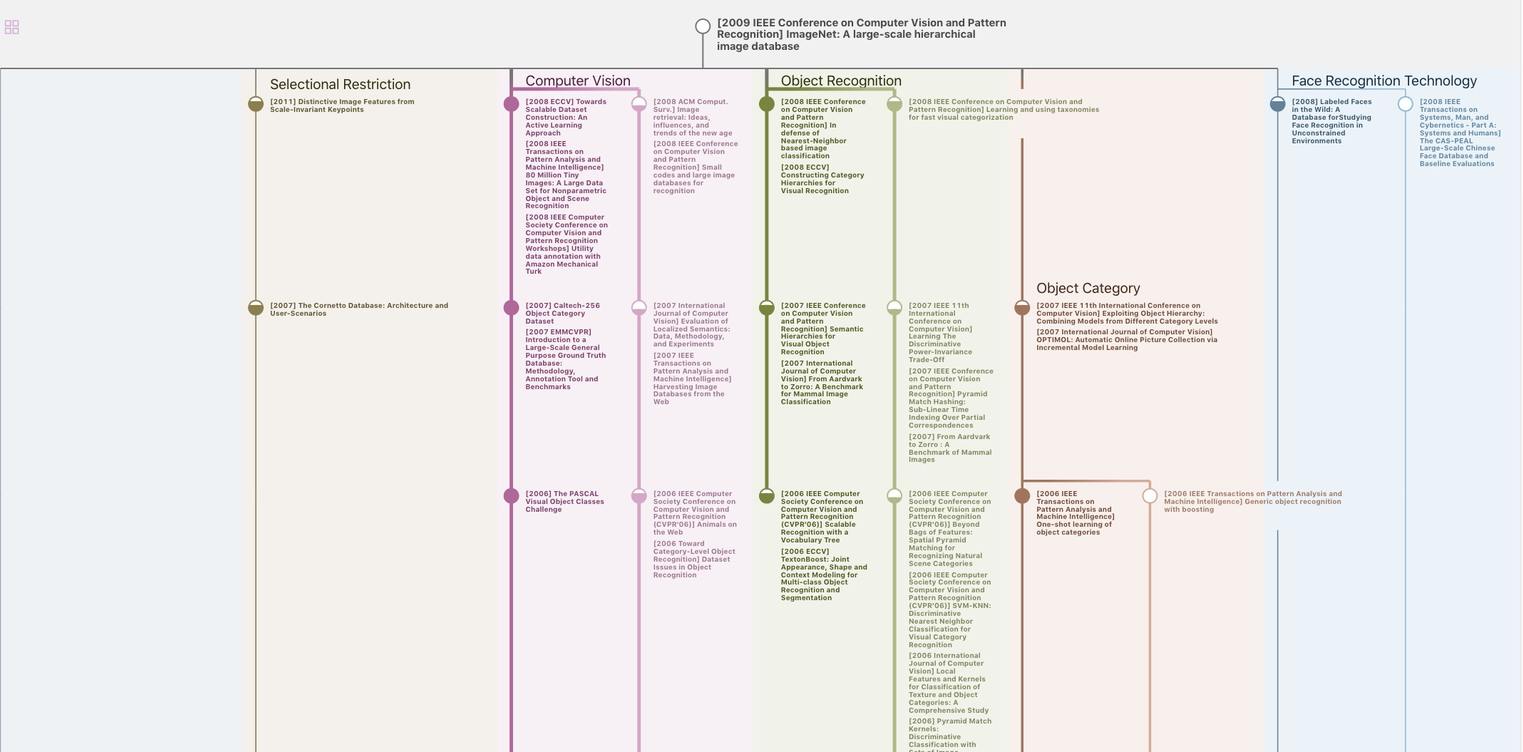
Generate MRT to find the research sequence of this paper
Chat Paper
Summary is being generated by the instructions you defined