Efficient Similarity Search With A Pivot-Based Complete Binary Tree
IEICE TRANSACTIONS ON INFORMATION AND SYSTEMS(2017)
摘要
This paper presents an efficient similarity search method utilizing as an index a complete binary tree (CBT) based on optimized pivots for a large-scale and high-dimensional data set. A similarity search method, in general, requires high-speed performance on both index construction off-line and similarity search itself online. To fulfill the requirement, we introduce novel techniques into an index construction and a similarity search algorithm in the proposed method for a range query. The index construction algorithm recursively employs the following two main functions, resulting in a CBT index. One is a pivot generation function that obtains one effective pivot at each node by efficiently maximizing a defined objective function. The other is a node bisection function that partitions a set of objects at a node into two almost equal-sized subsets based on the optimized pivot. The similarity search algorithm employs a three-stage process that narrows down candidate objects within a given range by pruning unnecessary branches and filtering objects in each stage. Experimental results on one million real image data set with high dimensionality demonstrate that the proposed method finds an exact solution for a range query at around one-quarter to half of the computational cost of one of the state-of-the-art methods, by using a CBT index constructed off-line at a reasonable computational cost.
更多查看译文
关键词
algorithm, similarity search, index, tree, pivot
AI 理解论文
溯源树
样例
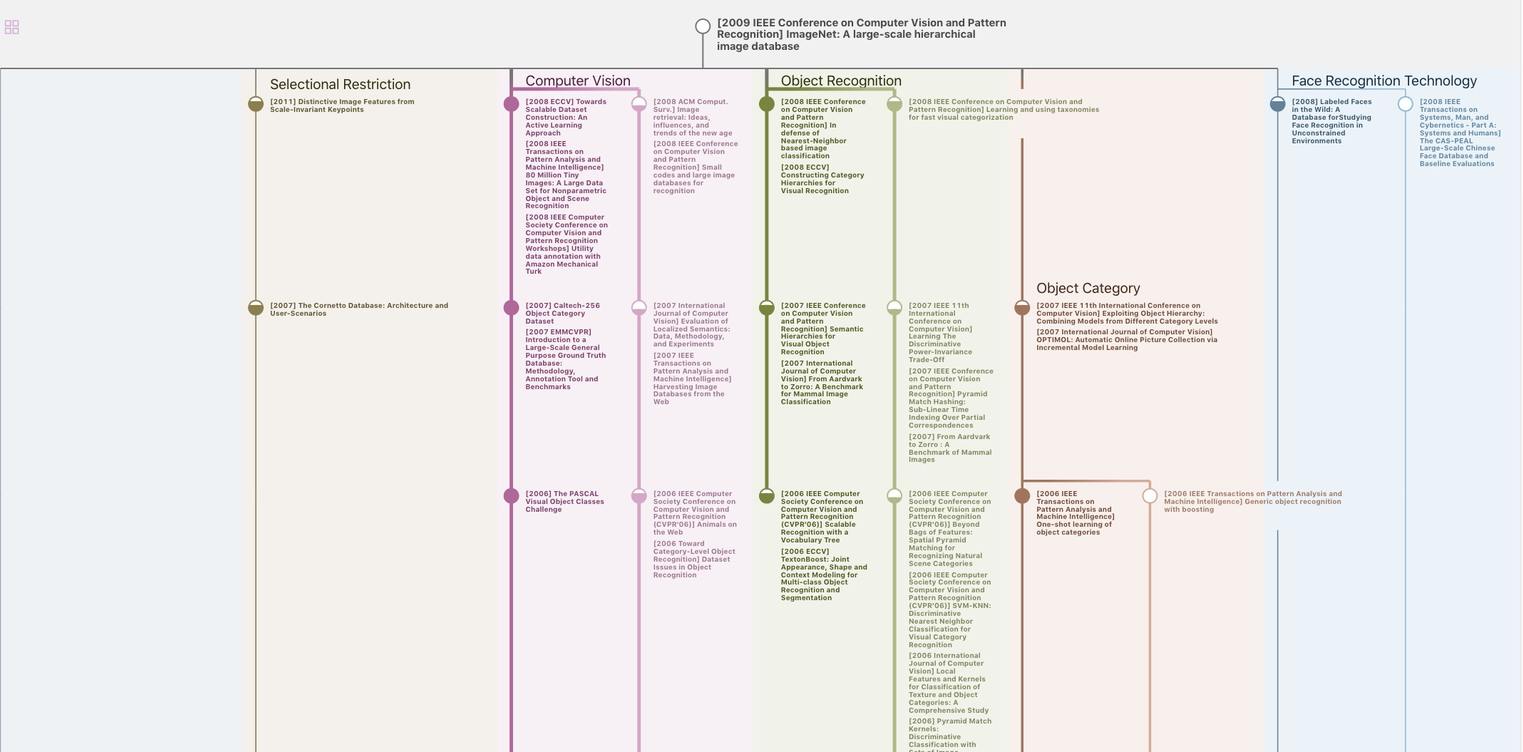
生成溯源树,研究论文发展脉络
Chat Paper
正在生成论文摘要